Table of content
Demand forecasting is an indicator of possible potential goods or service demand. It is also used sometimes with demand planning. However, demand planning is a process that is not limited to forecasting but commences with it.Data Science and Machine Learning can predict human behavior and give valuable figures and suggestions for decision making. If we look at human activity from sales data research, we can have more useful perspectives than public polls.[lwptoc skipHeadingLevel="h1,h4,h5,h6"]We will discuss the data science and machine learning approaches and logic to help customer demand forecasting in different environments in this article.
Machine Learning In Demand Forecasting Solutions
Increased computing capacity and increased demand uncertainty established the requirements for increased machine learning used to design forecasts.
Why do we need it?
An extremely detailed demand prediction is the only way retailers can foresee which products are required for each store — and is the only way to maintain strong consumer supply while holding stock risks minimal.
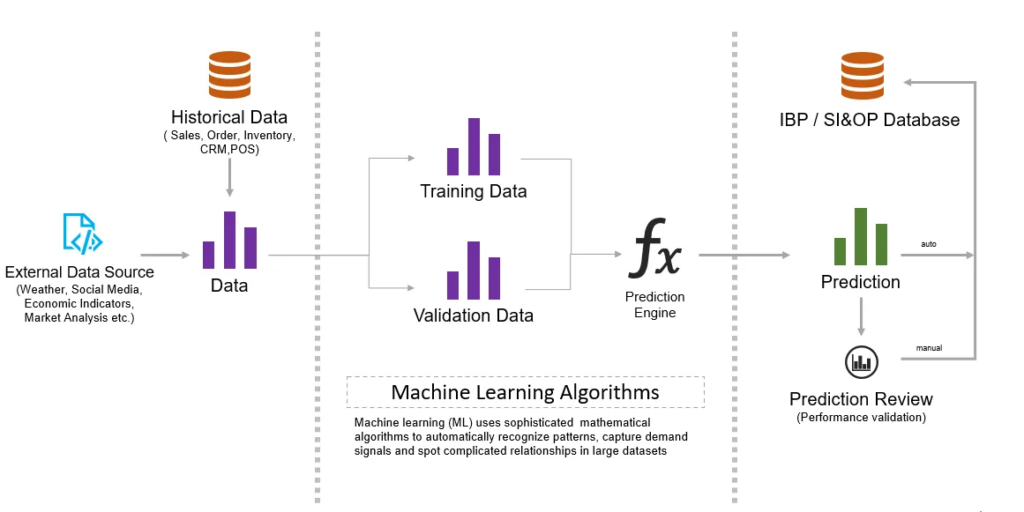
Image Source: Digitalsoft
The detailed forecasting in stable conditions is usually pretty easy, but retail is fundamentally complex, with tons of regularly influencing demand. To handle such complexions, we need Machine Learning to simplify things for us.An accurate prediction throughout retail companies may also promote capacity management, maintain the correct number of employees in stores and dealer centers, or help customers handle long-term sales dynamics.
Machine Learning Methods in Demand Forecasting
Machine learning methods can estimate the amount of products/services that can be bought over a given period. A software system may then benefit from the data for better evaluation. In this situation. Machine learning, as opposed to conventional forecasting methods, helps in the following ways:
- It gives a more reliable calculation.
- Determines hidden data trends.
- Online prediction updates based on the most recent information.
- Improves adaptability.
- Speeds up data processing and analyzes more data.
- It makes a stable framework.
Machine learning has been applied in numerous forms through varied industries. Its implementation depends on the availability of data, the nature of the activities, and what the business needs from its demand prediction.
Also Read: 5 Data Science Integration Challenges in Your Existing System
It can foresee the shifts in the pricing of a current product as the season changes or plans to adjust rates and build a market for any upcoming products or services.

Image Source: RelexSolutions
Machine learning helps to include the numerous variables that affect demand on your retail forecasts regularly. This is incredibly useful since data comprises scores of multiple variables that can affect the market.Machine learning algorithms create quality improvement models dynamically, utilizing either the data supplied by you from your organization or external data sources. The most significant advantage is that such a system will handle retail data sets from several sources without any human effort.
Data Sources for Machine Learning
Machine learning is based on mathematical models, allowing more precise, data-driven forecasts utilizing both internal and external information sources. Machine Learning engines may use structured and unstructured data, including previous financial and revenue records, surveys, macroeconomic metrics, social media signals, and more.

Image Source: IBF
It would help if you had ample computing resources and vast batches of high-quality data to best make the machine learning approach. If not, the machine would not be able to understand and develop valuable forecasts.
Data Science for Modernized Demand Forecasting
Although modernized demand forecasts may offer more precision and specificity in predictions than conventional approaches, You should remember that Data Science methods tend to foresee how the system is designed without human interference.That being said, manual quality monitoring is necessary to maintain effectiveness. There are situations in which incorrect signals are obtained in demand sensing. Thus it is needed to have human intervention during the rising process.
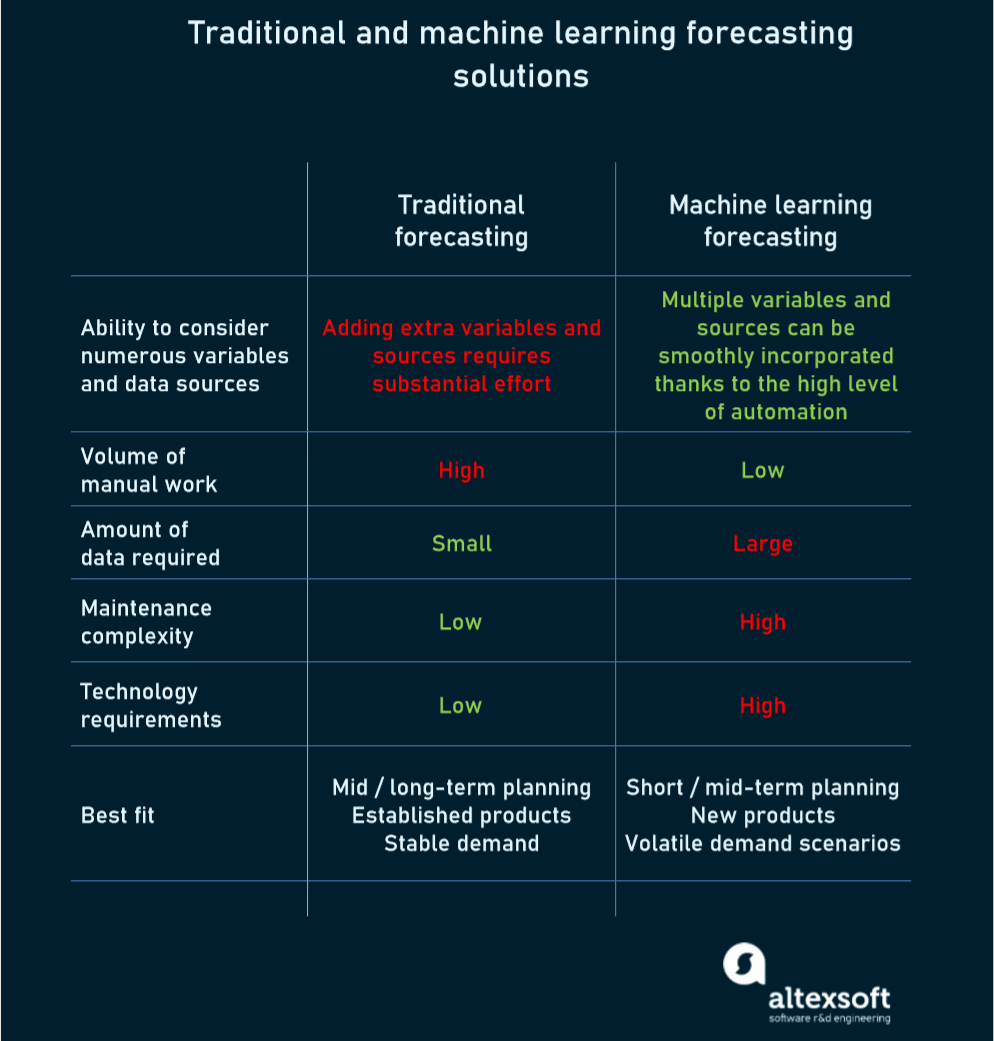
Image Source: AltexSoft
Different machine learning and data science approaches can accommodate more extensive data and more complicated variations and trends. Intelligent demand forecasts evaluate large volumes of data and continually update the design to react to fluctuations by modifying it to new environments. This all results in more precise and credible forecasts in the retail sector.Regardless of how intelligent a predictive approach is, the most important decisions rely on human beings. They test the efficiency of the predictive model to ensure it is reliable in a dynamic and changing market setting. Only by having the full use of both AI and human intelligence can we identify and prepare a better future for our retail sector.According to Mckinsey Digital, Machine Learning forecasts can minimize supply chain networks' errors by 30 to 50 percent. Improved precision contributes to a 65% drop in lost revenue because of stock product circumstances and warehousing prices. ML's projected effect on the production and supply chain preparation supply chain is between $1.2 and $2T.

Source: McKinsey
ML-based Demand Forecasting for Retail Design Algorithm
When creating the demand forecasting feature, you need to understand the Machine Learning Modeling workflow. This creates a data-driven roadmap for optimizing the phase of growth. Follow the following steps:
- You need to provide the consumer with meaningful insights when starting the project. Start with gathering all the data that is accessible and review the structure, consistency, and accuracy, followed by running some tests.
- Establish the customer's outlines of market goals and additional requirements. Set your business goals and see which products you will be analyzing as different products have variable demand forecasting outputs. You need to pick the time frame for the demand forecast as well; usually, short-term predictions are made in less than a year, and long-term forecasts can last more than a year. After that, you need to set a minimum forecast accuracy level.
- Prepare your data by cleaning, testing, and structuring. Your data should be accurate, complete, consistent, and relevant, as data quality is a vital part of a precise demand forecast.
- Select the machine learning model depending upon the type of data, quality, amount, business goal, time frame, etc. The most popular approaches are time series and linear regression approaches. Feature Engineering and Random Forest are also quite common approaches.
- As the forecasting models have been created, you should begin the training phase. Optimize model parameters for forecasts to obtain high efficiency. Maximize the empirical outcomes in the improvement step. The final implementation process includes the incorporation of the forecast model(s) into production.
Conclusion
Data Science and Machine learning in Demand Forecasting For Retail has progressed to a stage of accurate, transparent, and replicable results. Human logic is also needed to ascertain the importance of digital results and conclude with rational thinking and domain knowledge. Data Science and Machine Learning based demand planning frameworks provide a collaborative forum that enables multiple experts to engage in a forecasting process.Let us know in the comments section below about your experience or queries regarding the in-demand forecasting in retail using ML and Data Science.