Table of content
AI Consultancy in a Nutshell
AI consultancy is the domain that is intended to provide services to help businesses deploy Artificial Intelligence (AI) or Machine Learning (ML) methodologies across different departments to improve their functional efficiency and operational tendency. Different consulting firms estimate differently about AI’s economic share and future growth, but companies like McKinsey, PwC all rightly claim that AI is a multi-trillion dollar economic opportunity for the world that will be unleashed by the mid of the 21st century.
However, as determined in a report by BCG and MIT Sloan Management Review, think tanks state these 3 factors as the leading cause of slower transition and adaptation towards AI.
- Absence of an effective AI strategy
- lack of awareness about AI and its true potential
- Talent Deficit with regards to AI or ML professionals in the organizations
This is where the role of AI Consultancy came into play as they help organizations in coping up with such issues.
- Strategy & Planning is their primary tool.
- They have trained & motivated professionals doing research on different AI models and identifying AI use cases for businesses.
- They can assist businesses in improving their approach regarding the AI talent hunt and help develop intelligent solutions.
Why is AI consultancy becoming important?
Integration of AI consultancy services and applications is becoming a new norm. Currently, there is a huge demand for AI-based products, but the supply line is sluggish. Either the technology produced by immature personnel isn’t mature enough, or the businesses don’t know which firm to outsource from. Hence, AI consulting will be in overwhelming demand in the near future.
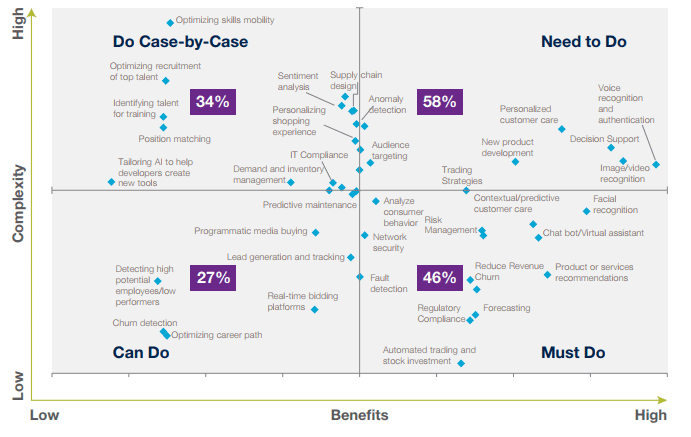
Courtesy: Capgemini Consulting
This illustration by Capgemini Consulting showcase this problem. Many firms fail to crack the opportunity to implement less complex and highly beneficial AI use cases. This case falls inside the “must do quadrant”. According to the survey, 54% of the firms in this quadrant failed to implement the recommended use cases. Another survey conducted by Boston Consulting Group and MIT Sloan Management Review reveals the expectations of business entities. Many industries possess the potential to adapt to the new technology, and the rest projected a 5 years span to attain the same potential.
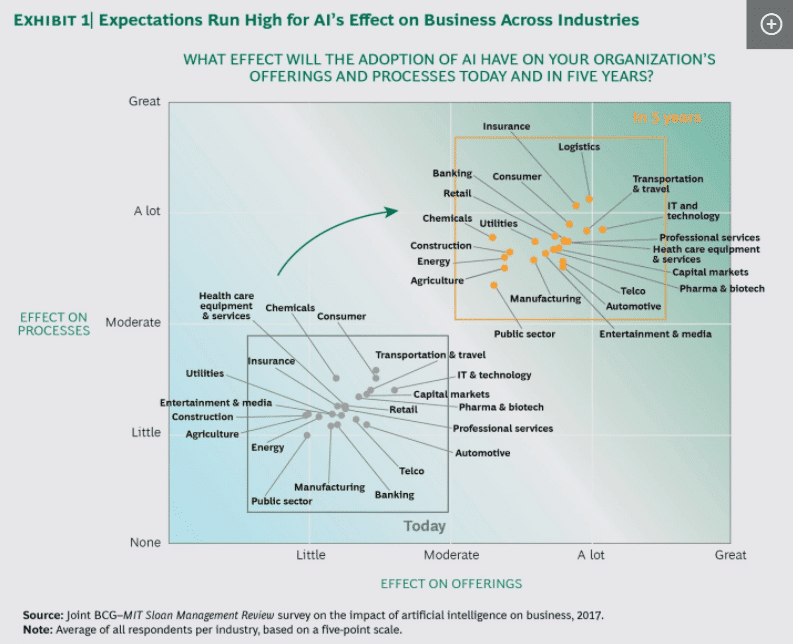
The aforementioned evaluations reveal that while businesses have high expectations from AI, they are not experiencing all the perks and advantages of AI due to a lack of understanding and underwhelmed strategies.
What are the typical AI consultancy activities?
In general, AI services providers assist businesses in adapting to AI transformation seamlessly and effortlessly. Every consulting practice can be segmented into 4 primary aspects and AI consultancy is no exception.
1- Strategy formulation
How should the client use AI? It’s crucial to acknowledge the challenges and opportunities experienced by the company by analyzing the client’s data & capabilities. Integrating all these factors with an understanding of high-end AI methodology, a consultant can outline and suggest the most important AI initiatives for the client’s organization. By following the suggested initiatives, companies’ overall existing strategy also needs to be revised. For instance, Business Process Outsourcing (BPO) companies produce a notable amount of revenue by handling invoices for other companies. These services revenues are vulnerable to a high extent since invoice automation can be handled by AI technology. Vendors like Hypatos can utilize ML and deep learning models to fetch data from semi-structured documents and process them to generate invoices.
Another example is translation services providers. Such facilities are bound to make revolutionary changes to their operational structure to ensure that it survives and thrives upto the next decade when Google Translator attains human-level perfection and accuracy. It is helpful to have planning sessions to foresee and plan 5-10 years into the future, helping decision-makers understand the true potential of AI so they can identify how their business needs to start evolving rapidly.AI consulting services develop the strategy in the following steps:
- Understanding your company’s contemporary status: Using methodologies like process mining and analyzing the company’s strategy to get a deeper understanding of the company’s contemporary status and standing.
- Developing a portfolio of potential AI initiatives: This step involves identifying pain points and understanding how AI can solve the potential issues and clear unseen roadblocks.
- Predicting the value of a project: Most AI projects fail to produce promised value. AI consulting vendors assist businesses in foreseeing the value of the project so that businesses refrain from investing more than the expected outcome making a perfect balance between price and value.
- Choosing AI methodologies and data sets to train machine learning models: AI consultants should be familiar with the capabilities and boundaries of each technology in a certain domain. According to business requirements, consultants opt for the right AI solution and appropriate data training sets for implementation. If the organization does not have the necessary data that can be used as an effective train set, consultants can help find or label data with their rich market expertise. To acquire clean and relevant data, businesses depend on data collectors such as Bright Data. Bright Data’s data collector provides real-time public data belonging to different market domains or eCommerce entities that can be used by businesses in designated formats.
- Launching pilot projects: Consultants help initiate initial small to medium-scale projects to ensure the quality of the data and methodology as well.
- Identification of scaling challenges and coming up with a solution: After analyzing the results of supplementary projects, AI consultants provide businesses with a strategy and planning regarding a comprehensive approach.
2- Commercial due diligence
Though due diligence is deemed a strategy project, a rich how-know of the AI market is necessary to conduct effective due diligence because success factors in AI vary greatly compared to other fields. Consulting firms having a rich history of conducting due diligence drives such as Solon are pushing their limits to establish their presence in this trending domain. Due diligence demands a consulting team to prepare the inputs to a valuation in a short span of time, typically in 20 to 30 days. Based on the commercial and other due diligence variables, the buyer that might be private equity, corporate, or any investor offers a bid. It involves 1 or 2 steps. As we have mentioned earlier, evaluating the corporate success of an AI solution is different than other software projects primarily due to:
- The superiority of deep-learning-based ML methodologies requires an enormous amount of perfectly labeled data than competitors. Any software improves as product owners outline key patterns from usage trends. However, in the case of AI or ML, the accuracy of the model predictions improve as more and more unique data entities are made available.
- Evaluating data science teams is different from evaluating mainstream software project management teams. For instance, the academic record can be more helpful for data science experts compared to typical software developers.
Therefore, companies are pushing their limits and expanding budgets to spend on AI-specific due diligence capabilities. These include understanding and evaluating data sources that can be beneficial for AI or ML models, suggesting fast approaches to benchmarking different AI vendors’ solutions, and embracing AI-oriented academic and work credentials.
3- Implementation
The strategy will result in a number of initiatives. Implementation should also be considered as multiple activities such as planning, vendor selection if needed, project management, development, improvement of business processes impacted by the project, change management, and so on. As with any consulting service, some or all services can be completed by consultants. Or implementation can be in-house and in most cases it is in-house. However, for example, if the client lacks the tech know-how to implement urgent initiatives, starting with consultants can help the client progress faster. However, please bear in mind that, in the long run relying on consultants completely for implementation will likely be more expensive than completing those activities in-house.
4- Training
Ideally, consulting projects should improve the culture and skills of the client. This is especially relevant in the field of AI where talent is scarce. AI consulting projects need to ensure that client teams are capable and knowledgeable about the technologies they will be working on.
How to choose your AI consultancy firm?
Of course, deciding on which firm to hire depends on many factors; but here are three major questions you need to ask;
- Is it really necessary? That should be the question you need to start with. There are many publications indicating the potential gains from implementing the solution, but is it going to be a positive return in the short run? You should ask your consulting about her projections for the short-run, medium-run, and the long-run. Maybe it would be wiser to implement some other technology for the short run if the firm is in the early growth stage or there are much more important opportunities to invest in.
- Do you have the necessary human capital? After the solution scheme is provided it would also be important to decide on doing the project in-house or outsourcing it. You should always make sure that the people with rights skills deal with the issue so that your employees would have a greater likelihood to learn something from the process and, can help you in later stages. Thus your team’s skill level will make it easier or harder to evaluate the performance of the consultant. So that, there would be constant sharing of information to ensure that state of an art solution is implemented.
- Does the consultant have the necessary experience? Right now, there are many small size artificial intelligence consulting firms. Depending on your industry you need to make sure you selected the right vendor. Different industries need different types of skill sets. Vendors’ past projects would be the greatest source of information. The profile of the team also matters. It is quite likely that people with advanced degrees have the capability to ensure the highest quality of work. The different consulting firms have different expertise, one can have the best team to do text classification and the other can be good at object detection.
What is the future of AI Consultancy?
We see two trends shaping the industry:
Rise of AI Consultancy Firms
AI is consuming the world just like the software domain did once. The largest consultancy of the internet era, for having the higher number of consultants, is Accenture which is based on software consultancy & deployment. The largest AI consultancy in the next 2 decades, will likely focus on Artificial Intelligence. Renowned consulting companies may have similar opportunities. As we have witnessed that facilities like Quantum Black and initiatives like BCG Gamma, established sibling firms centric around AI. However, we experienced a paradox here:
- Established companies in general and consultancies refrain from compromising on their pricing structure as it would threaten their existing products and services.
- When you have a hammer, everything appears like a nail. Consultancies have a great number of market experts and resources skilled in performing manual data analysis. It is difficult for such organizations to make the transition towards machine learning to fetch valuable insights from data and automate their analytical practices.
Based on these factors, we expect specialized, machine learning-oriented consultancies like Palantir to surpass the potential competitors and tech giants. Whereas, established consultancies remain at the bottleneck of providing expensive AI-based solutions that only the most profitable companies with enormous budget expenditure can leverage from.
The continued influence of entrepreneurship driving consultancy projects
In the report by Gartner, it was revealed that by the end of 2021 startups will be dominating the artificial intelligence domain and it is happening right now. Although being a typical industry analyst, it is difficult for think tanks like Gartner to make predictions, but the startups have an overwhelming influence in emerging tech domains areas like AI and ML. It comes amidst more workload for consultants as startups tend to partner with consultants to promote and incorporate their solutions. Sooner or later, tech giants like Google and Facebook will have less presence in AI methodologies unless some revolutionary measures are put into place. We indeed see that AI vendors are becoming highly specialized and offer market-based solutions. But it is too soon to make accurate predictions as tech giants have all the required resources and tendencies to dominate the AI domain by absorbing AI startups and hiring researchers as they have been doing historically.
Traditional Consultancy in a Post AI World
We have discussed how AI consulting works. It is also worth noticing what will happen to mainstream consultancy mediums as AI becomes more instrumental in every key area. In this blog post, we have pondered over some critical aspects including the USPs of consultancies that are likely to be strangled by skyrocketing trends in AI and why it is normal to expect that consulting, especially the business of conventional management consultants is likely to shrink in the near future. As more AI projects fail due to a poor understanding of problem statements and possible solutions, choosing the best AI consulting partner is crucial to mark the 100% success of any AI project or transformation drive. It involves a wide array of services from data wrangling to deep learning to assisting organizations in cryptocurrency, finance, healthcare, eCommerce, aerospace, and digital marketing.