Discover the latest trends and insights
Artificial Intelligence
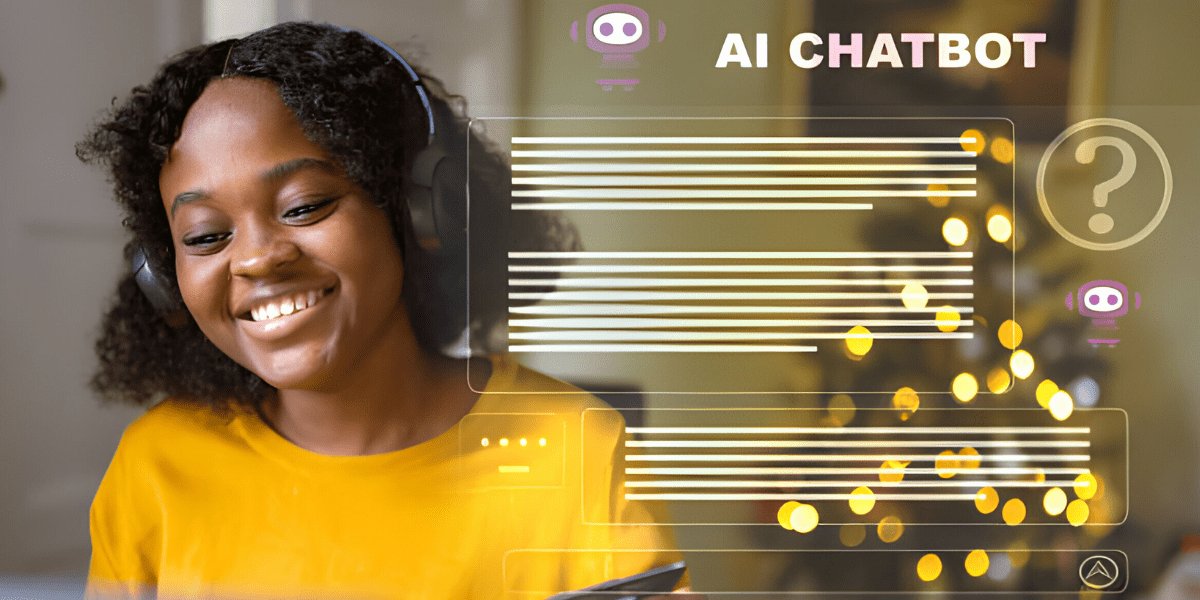
10 Best AI Shopping Assistant Tools for E-commerce in 2025
E-commerce businesses have a common goal of enhancing the shopping experience for customers. While some brands find a way to understand what the customer is looking for, others don't. That said, we live in the age of artificial intelligence, which means we don't need to rely on guesswork.
This is where an AI shopping assistant comes in handy. It creates personalized recommendations and enhances search capability for added convenience. Here, we look at best shopping assistant tools for eCommerce in 2025.
How AI Shapes E-commerce Strategies
In a matter of years, AI has reshaped the way eCommerce sellers manage their operations. For instance, they are using generative AI to write compelling product descriptions. Customer support is now completely automated with the use of GPT4. It’s only a matter of time before AI creates paid ad campaigns and launches them on its own.
It's no surprise that the market value of AI in eCommerce retail was USD 6.63 Billion at the end of 2024 The value is projected to grow at a CAGR of 14.60% by 2032.
Here's how AI chatbot shopping assistants can benefit eCommerce businesses:
Personalized User Experience
When your goal is to stay ahead of the competition, personalization is your ticket to success. AI analyzes search patterns, behaviors, and conversations with chatbots to learn customer information. This information is then used to offer personalized product recommendations. Today, AI shopping assistants can help solve customer queries without human input. Not only that, but they also make it easy for customers to find the desired products using visual search.
Streamlined Operations
Apart from content creation, AI is also improving operational efficiency. E-commerce retailers are leveraging AI tools to reduce operational costs and reduce time to market. For instance, AI automates inventory management by analyzing supply and demand. It identifies raw materials and supplies you need and suggests the right vendors. AI can also analyze sales data and customer behavior.
Improved Sales
AI can also improve sales by providing eCommerce businesses with valuable insights. It analyzes competitor pricing strategies to help you optimize yours. Moreover, enhancing search capabilities using AI shopping assistants can also help boost sales. It can identify cross-selling and upselling opportunities to extract higher revenue.
What to Look for in AI Tools for E-commerce
With more and more AI solutions being introduced to the e-commerce market, how can you select the right one for your business?
Let’s start with the basics:
- Identify the problem you want to solve using the tool. Your eCommerce business can use AI to improve data analysis and enhance customer service.
- Learn whether the tool can improve efficiency or reduce operational costs.
- Evaluate the best AI-powered personal shopping assistant tools available in the market. Look for the capabilities that will enhance your operations.
- Check for accuracy, integration, and scalability. Determine whether the AI solution can integrate with your eCommerce infrastructure.
- Ideally, the AI tool must be able to work even when your business grows in terms of size, channels, or locations.
- Consider the appearance, customizability, and user-friendliness of the AI solution. Your teams should not find it difficult to get their heads around the tool’s functionality.
- Another important aspect of AI tools is that they learn from the data they process. Does the tool require large datasets or specific formats?
10 Best AI Shopping Assistant Tools for 2025
While every AI shopping assistant tool is built with the same motive in mind, they all have their pros and cons. At the end of the day, the right tool for your business is the one that fulfills your unique requirements.
1. shopdev Shopping Assistant
shopdev's AI-powered Shopping Assistant transforms e-commerce. It provides personalized product recommendations, enhancing discoverability, satisfaction, and conversions. Empower your customers with our advanced AI-powered chatbot.
Conversational shopping assistant assists customers through their online shopping journeys. Its algorithms detect keywords entered by customers to find the right products.
AI-powered shopping recommendations are provided to customers based on the search query.
Multimodal AI combines information from different sources of a product page to deliver accurate results.
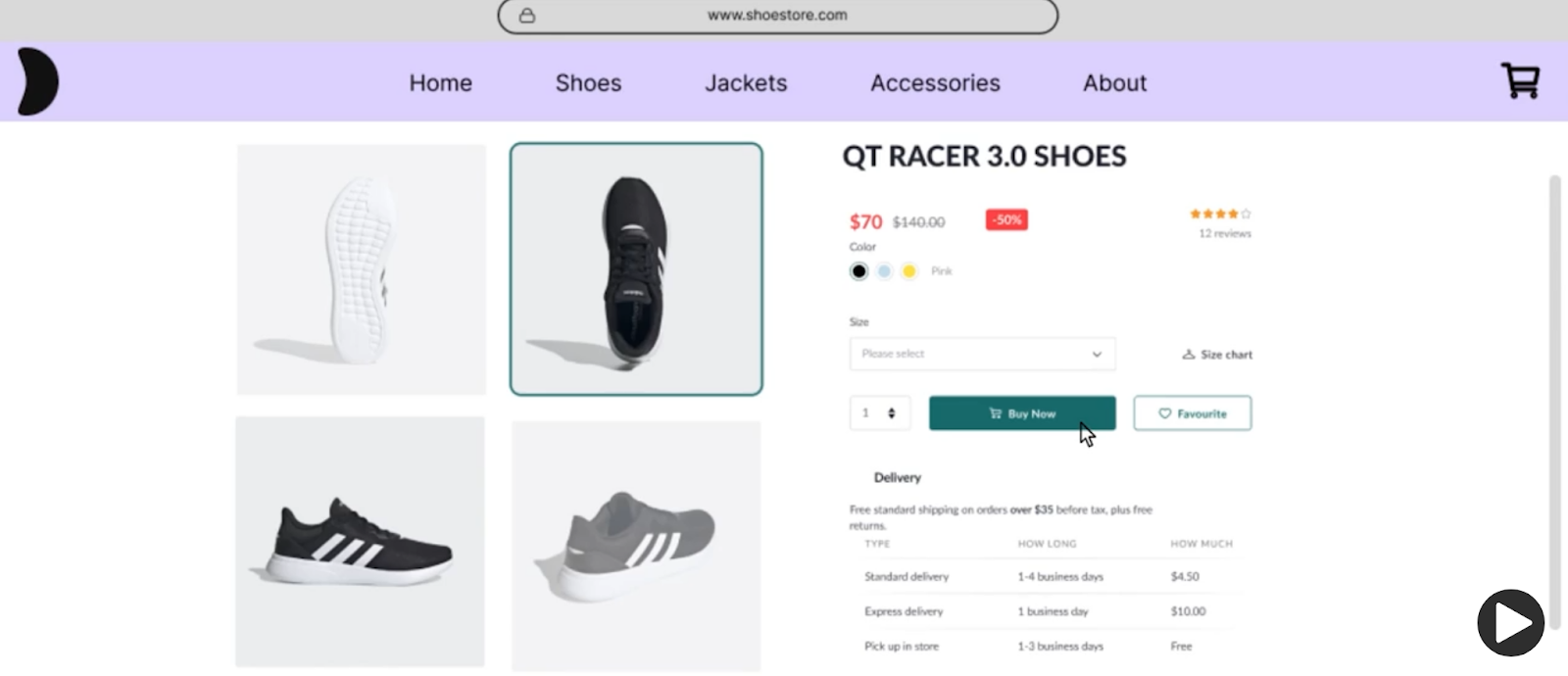
Features
- Personalized recommendations based on search history and preferences
- Object detection to differentiate between products
- Product discovery to find the right product for the customer
- Customizable UI for your brand’s design language
- Natural language processing to interpret search queries
- Computer vision to extract data from images
- Omnichannel support for connecting physical and online channels
- Multilingual support to serve a wide range of customers in different regions
2. Manifest
Manifest AI promises to improve sales by creating long-lasting relationships and is said to cut down the customer drop-off rate by 40%.
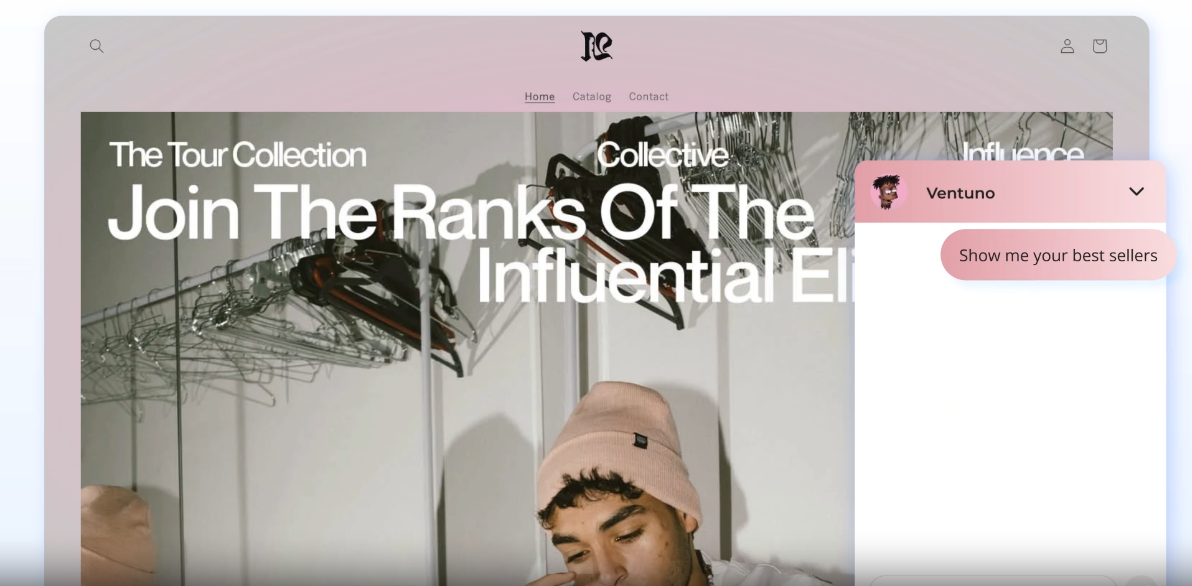
Natural language processing provides the capability to interpret any kind of customer query and help customers make purchase decisions. This means that the tool will find the right product even if the customer enters a vague description.
Image classification makes product search significantly easier and faster. To be on the safe side, Manifest AI provides 5 product recommendations as a result of the customer query.
Customizable UI allows eCommerce businesses to follow their brand guidelines.
Features
- Product discovery
- Natural language processing
- Image classification
- Customizable UI
- Multilingual support
3. Shopping Bot
ShoppingBot is a personalized chatbot that is trained on your website data to assist visitors in the best ways possible. It works around the clock to help eCommerce retailers enhance customer satisfaction and make them brand advocates.
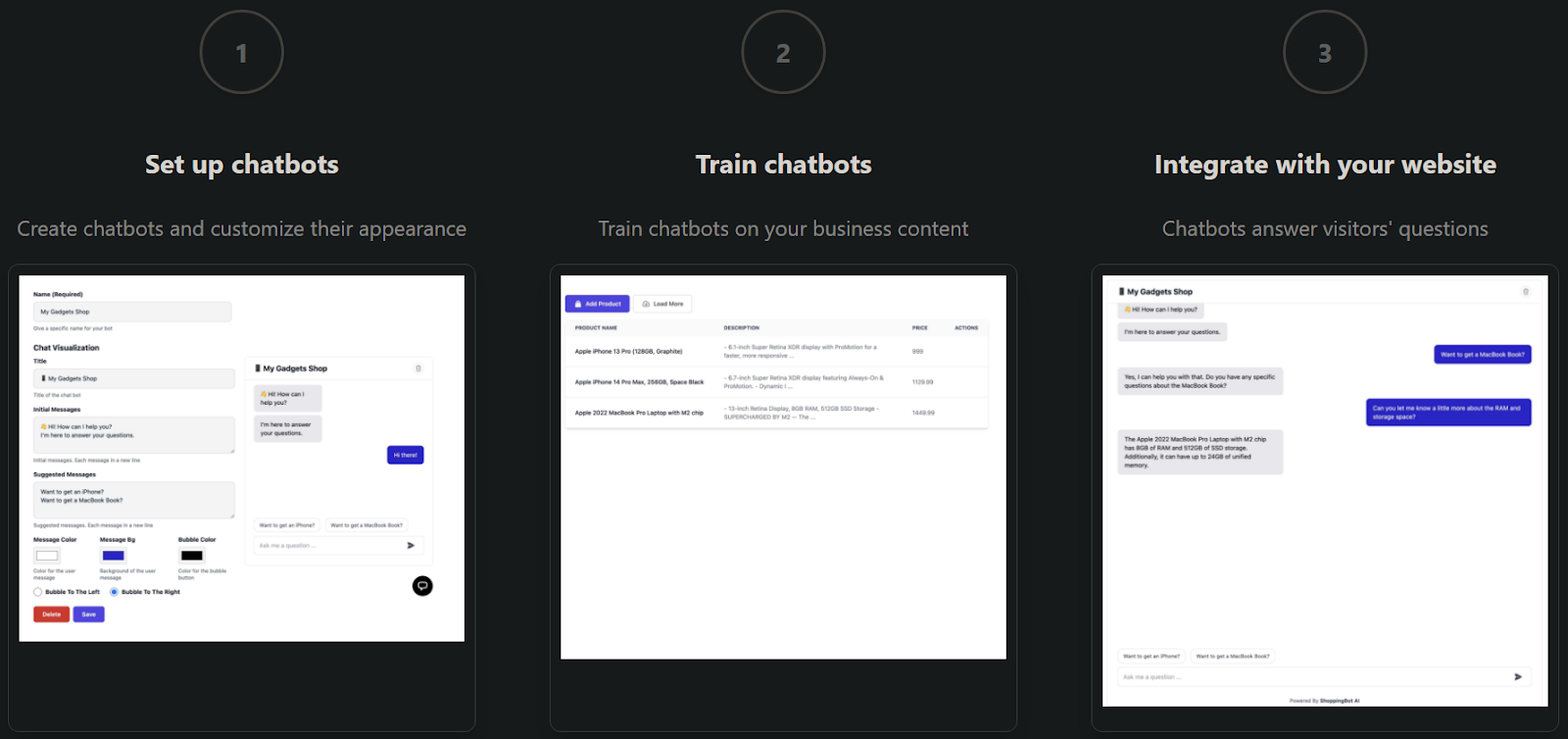
Easy to set up with email support for all users.
Ability to respond to customer queries within 48 business hours.
Strong security mechanisms with firewalls and encryptions to keep all of your data confidential.
Multilingual support is beneficial for eCommerce businesses operating out of multiple countries.
Features
- Personalized recommendations
- Secure conversations
- Product discovery
- Natural language processing
- Image classification
- Multilingual support
4. Shopmate
Shopmate is an AI chatbot that boasts 24/7 customer support, but it only needs 3 minutes to know your products and store information.
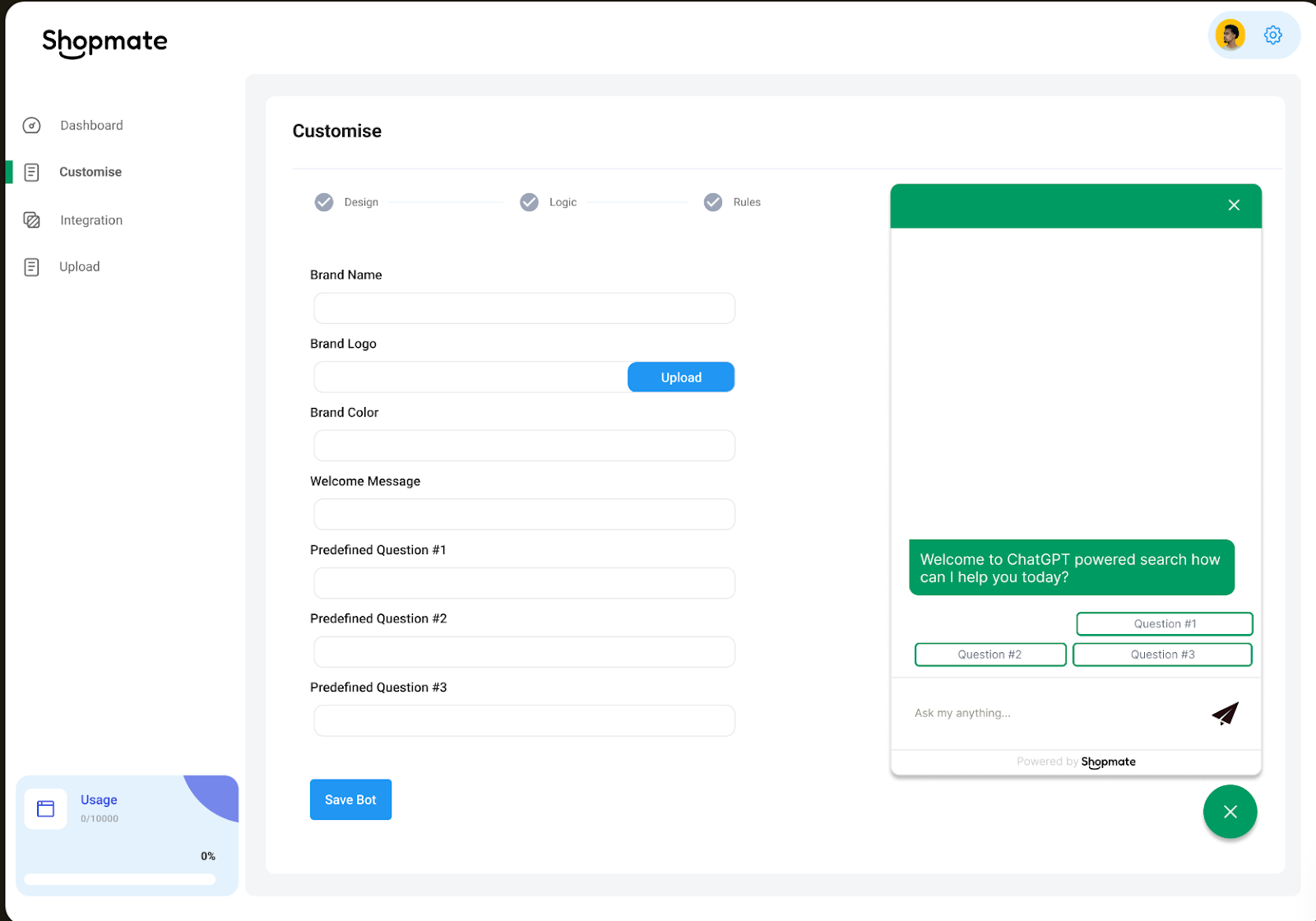
It analyzes your customers’ shopping history and preferences to intelligently recommend the right products.
Support handling feature allows you to answer customer queries instantly and efficiently.
Automatically analyze information from blogs, internal documentation, and FAQs on your site. This allows the AI shopping assistant to provide customers with accurate information.
Features
- Fast processing speeds
- 24/7 availability
- Personalized recommendations
- Secure conversations
- Product discovery
5. Intercom AI Chatbot
Intercom AI chatbot is focused on improving team efficiency and resolving customers’ concerns. The chatbot resolves 50% of support questions on its own, within an instant.
The chatbot named Fin is the result of a big breakthrough by Intercom in the field of sophisticated AI language models.
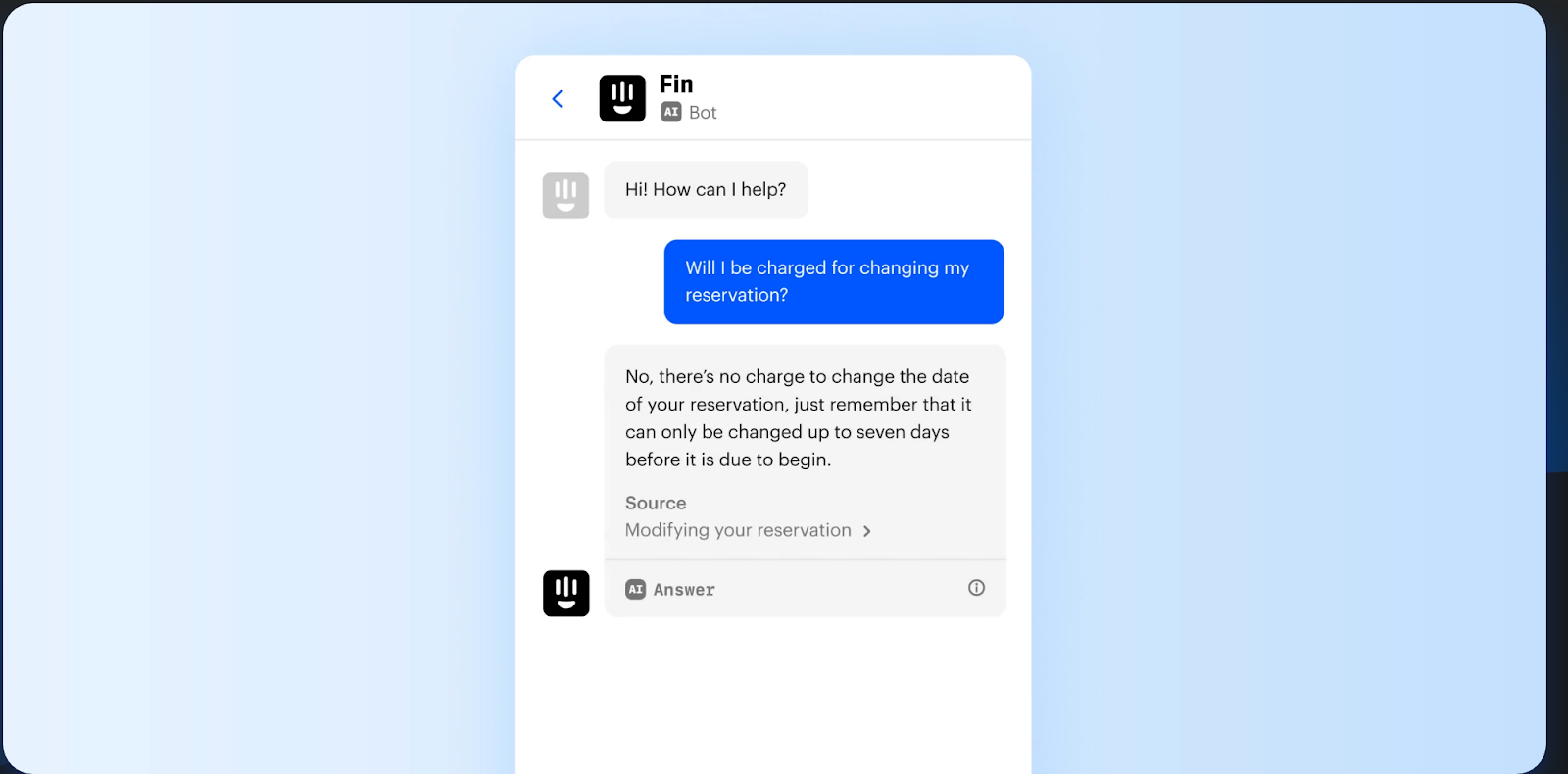
It provides accurate answers and maintains a conversational tone to make your customers feel at home.
Built-in safeguard to ensure that Fin only answers customer queries based on your brand’s guidelines.
It’s an AI tool you can trust with your brand’s secrets as your team can also monitor all of the bot’s responses.
Features
- Natural language processing
- Accurate product discovery
- Personalized recommendations
- Secure conversations
6. Infichat
Infichat is an AI-powered shopping chatbot that assists your customers in product searches. Customers can ask questions based on the descriptions, sizes, and colors of the product.

Personalized recommendations based on their shopping history.
24/7 availability makes it one of the best tools to enhance customer satisfaction and boost sales.
Minimal setup required. The AI tool is easy to use for any of your team members.
Features
- Seamless customer support
- Instant product discovery
- Personalized recommendations
- 24/7 availability
7. Tidio AI
Tidio AI, a user-friendly and functional live chat app comes with the best customer support out there. The chatbot and ticketing solution is purpose-built to serve mid-sized eCommerce businesses that wish to solve customer problems and improve sales.
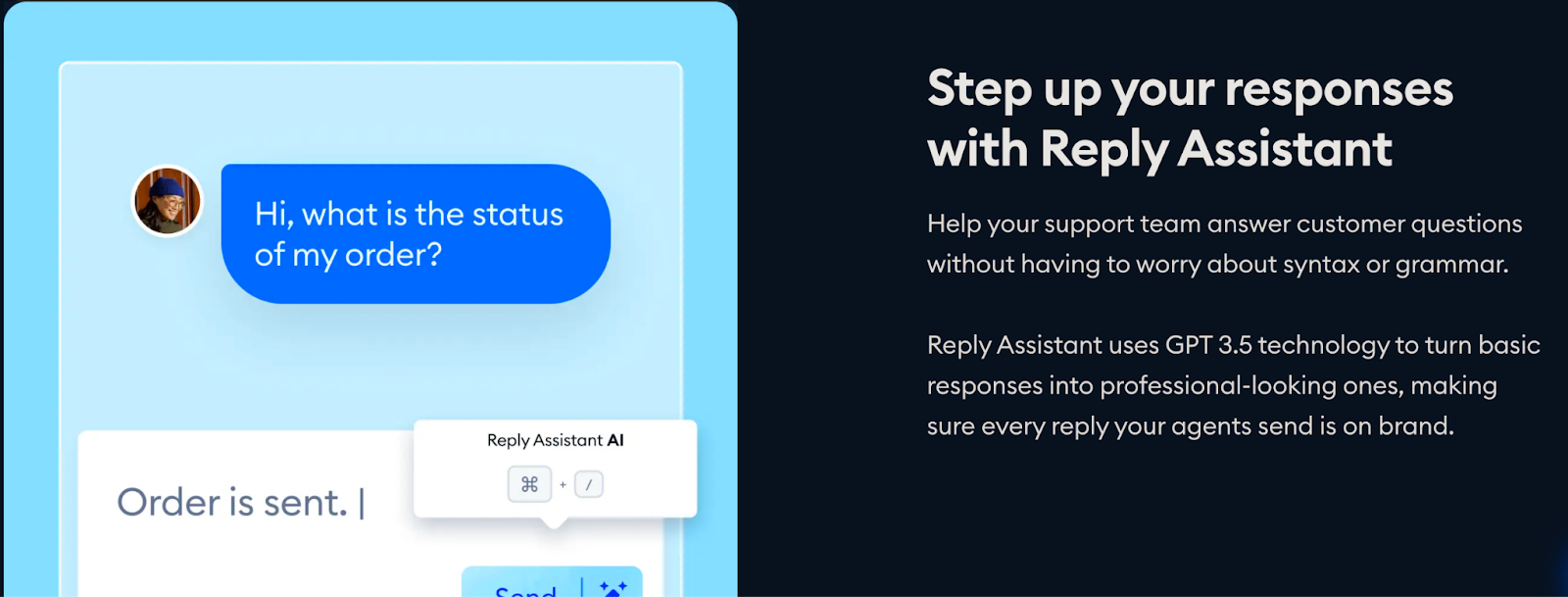
Customization based on your brand requirements.
Easy setup with extensive chatbot template library. These templates cover both sales and support scenarios, meaning your eCommerce brand doesn’t have to worry about responding to longer conversations.
The advanced AI behind Tidio is capable of holding long conversations with customers in case of highly-priced items.
Features
- Customization
- Built-in templates
- Seamless customer support
- Instant product discovery
8. Chatfuel
Chatfuel takes the convenience of setting up an AI chatbot to the next level. You don’t need any special skills in coding or programming languages. All you need to do is click a few buttons to select what you like the most.
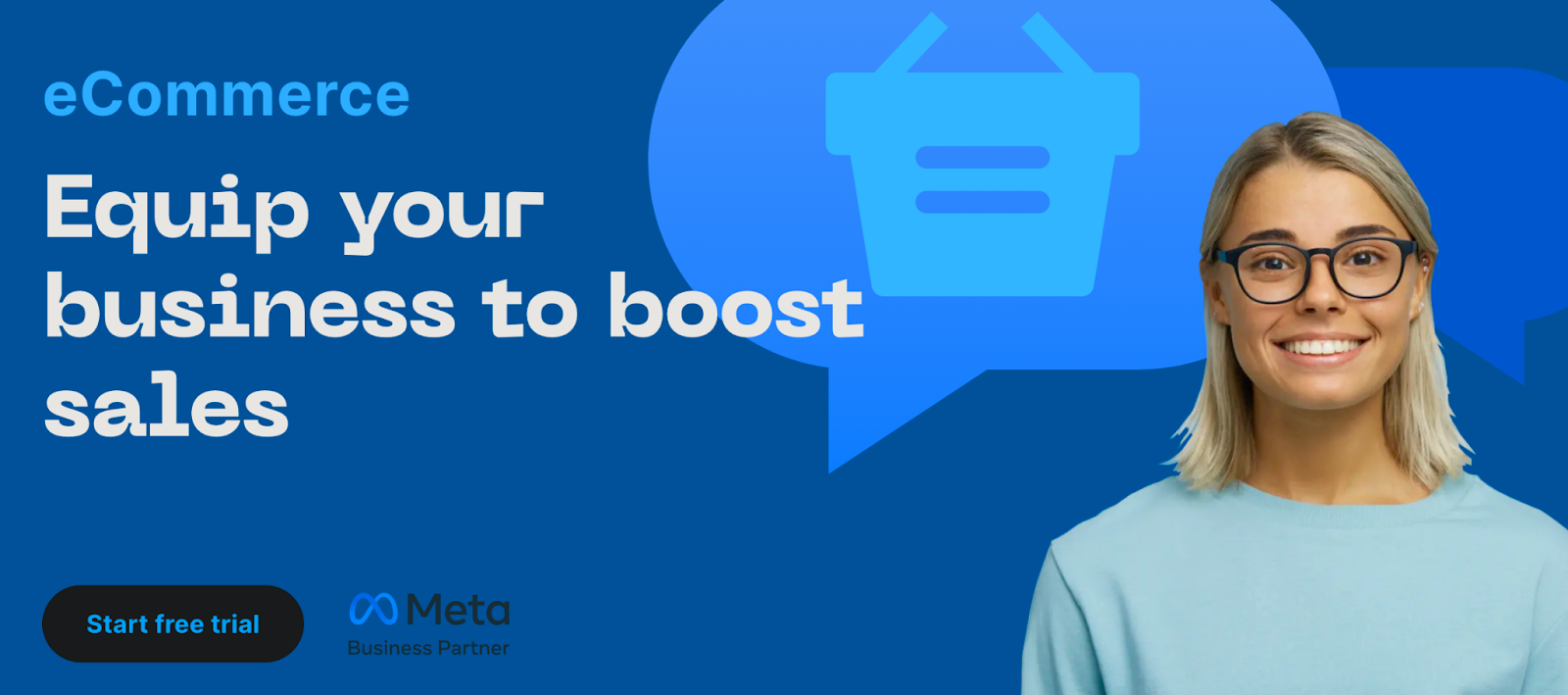
Drag-and-drop builder for creating your customized chatbots.
Ready-to-use templates and free catalog for popular eCommerce platforms.
Easy to use with an organized UI which makes the work all the more productive.
Integrates with external systems to enhance automation and performance.
Features
- Drag-and-drop functionality
- Easy to use
- Customization
- Ready-to-use templates
- Free catalog
9. Verloop
Verloop enhances your customer support by responding to customers in the way they like. This includes voice conversations, WhatsApp, Instagram, and web applications just to name a few.
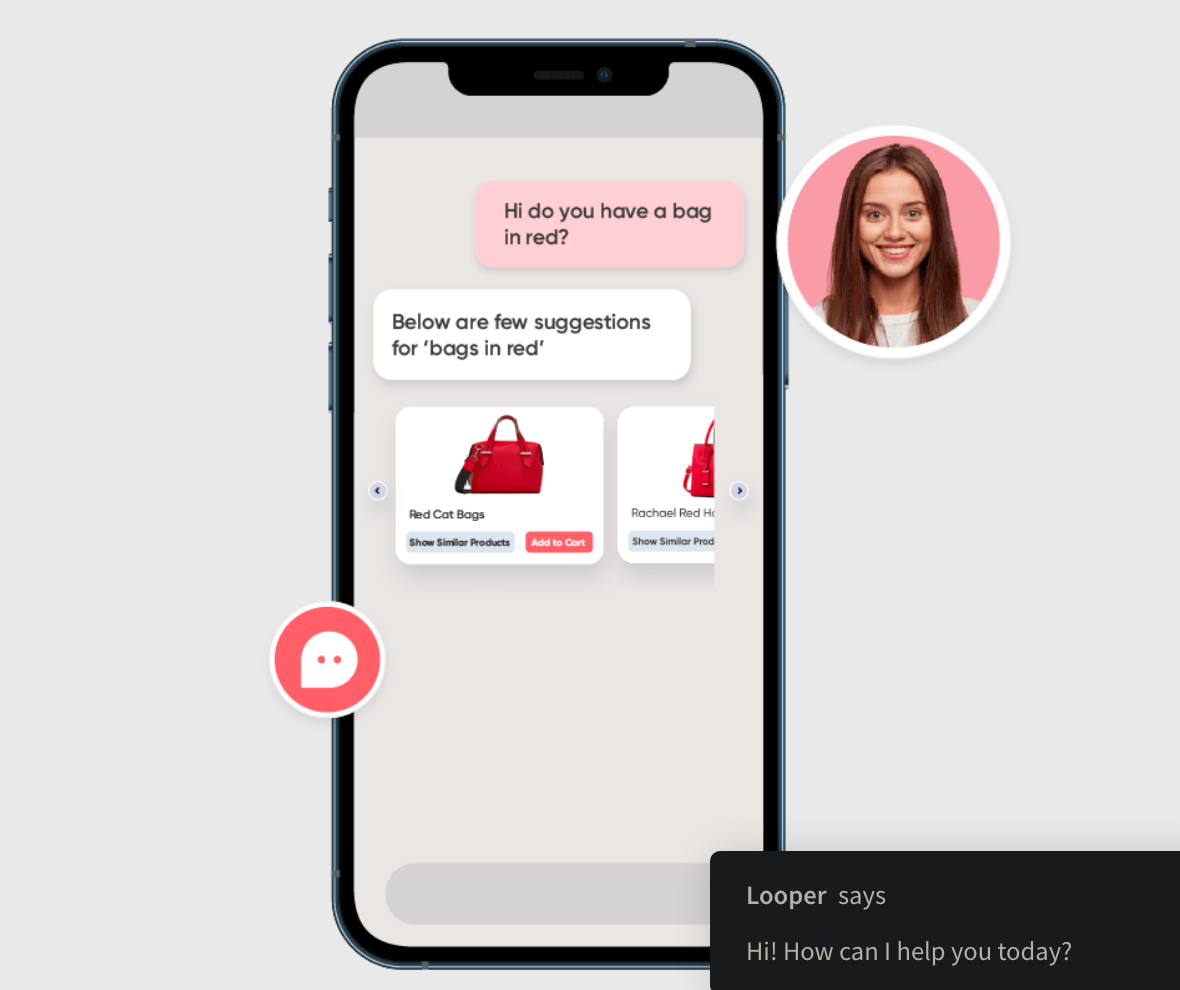
It is user-friendly and allows you to carry on conversations with up to 3 customers at the same time.
Your team can address more customer queries in less time.
You need fewer customer support executives to pay salaries to.
Features
- Customized bot solutions
- Personalized conversations
- One-to-many conversations
- Instant product discovery
- 24/7 availability
10. Giosg
Giosg is a sales acceleration solution that automates live chat using AI chatbots. Its interactive content helps build engaging online experiences for your shoppers and turns them into loyal customers.
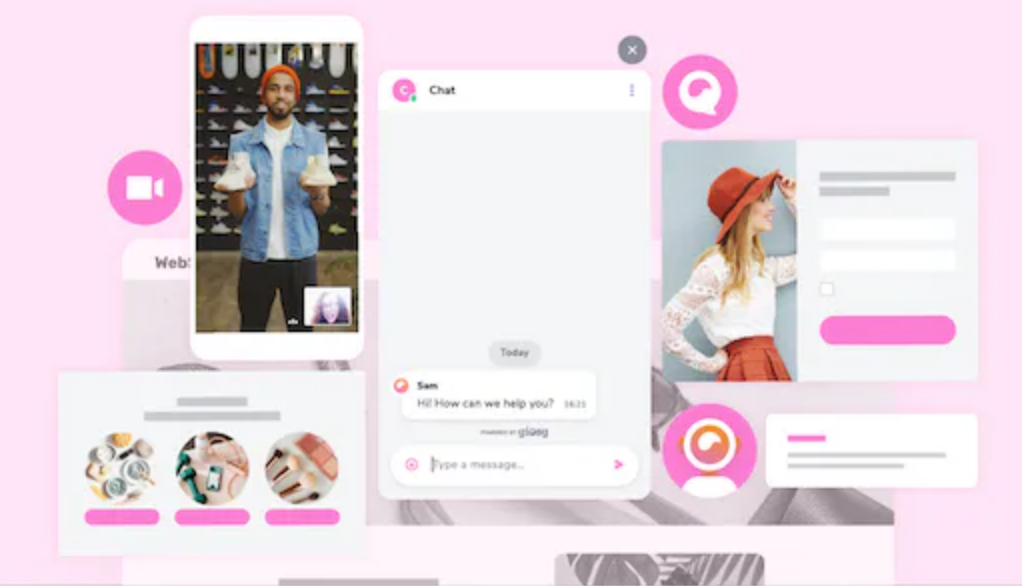
Easy navigation and built-in tools for marketing, communication, and handling support tickets.
Interaction builder allows you to create elements and interactions with customers on your website when required.
Features
- Seamless customer support
- Easy navigation
- Interaction builder
- 24/7 availability
Key Takeaways
As technology evolves, eCommerce businesses are looking to speed up their operations. AI shopping assistants are quickly gaining popularity in the industry. Both businesses and customers benefit from personalized product suggestions and accurate search results.
If you’re looking to build brand loyalty through AI-based personalized recommendations, look no further. shopdev’s AI shopping assistant has everything you need to take your brand’s customer experience to the next level.
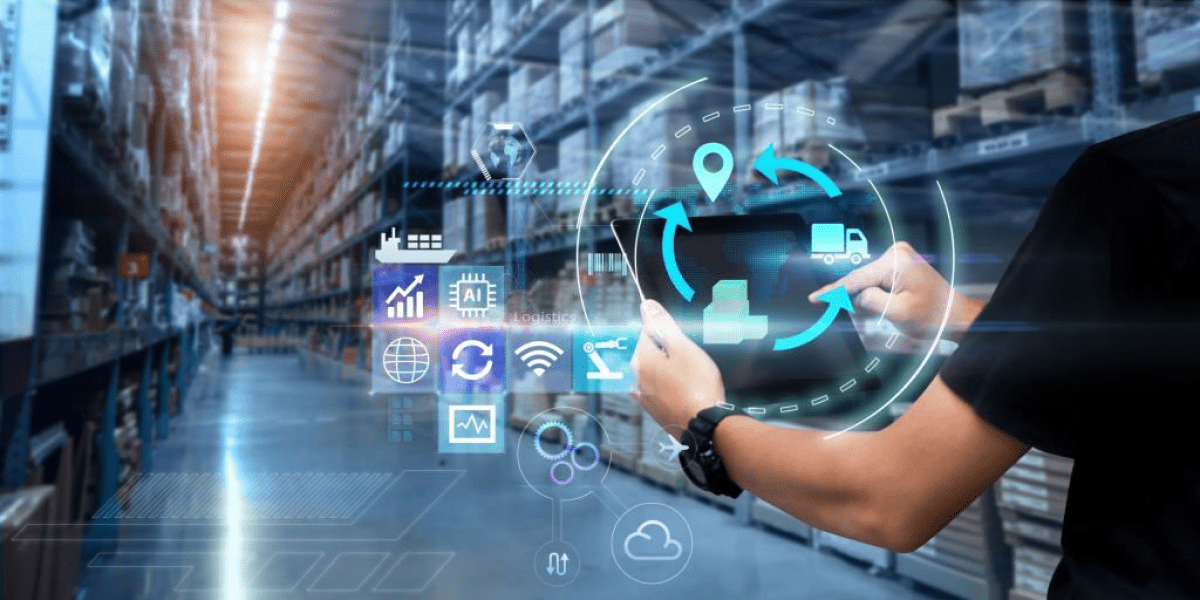
9 Generative AI Use Cases for Retail Success in 2024
Exceptional customer experience and loyalty are the keys to running a successful retail business in 2024. With thousands of brands to choose from, customers tend to fall for the ones that market their products with high-quality personalized content and offer the best customer service.
Thankfully, the retail industry now has a solution in the form of generative AI, which allows them to enhance their services while meeting their growth targets. According to McKinsey, generative AI can boost productivity in the retail sector by around 1-2% of global revenues, which is estimated to fall between $400B - $660B per year.
Read on to learn more about the applications of generative AI in retail.
Significance of Staying Updated with AI Trends
ChatGPT, Bard, Copy.AI, and DALL-E have quickly become buzzwords as businesses have begun to realize their potential in improving operational efficiency. But what if your business never knew about generative AI?
That’s right - success in the retail landscape is synonymous with staying updated. In order to stay one step ahead of your competition, you need to be aware of the latest trends in AI and keep an eye out for solutions that can improve your workflow.
Your customers are not willing to wait for webpages to load and carry out extensive searches to find the right product. Instead, they expect to see relevant content right from the moment they land on your brand website. In fact, 73% of customers expect your brand to know their preferences.
It's no surprise that 82% of organizations are currently using or considering generative AI as the technology that will transform their industry. It allows you to automate repetitive tasks and unlocks new business opportunities.
Generative AI Retail Use Cases
The use cases of generative AI in retail go far beyond enhancing customer experience and creating unique content. It provides business owners with valuable insights to make informed decisions while providing creative assistance in the form of unique texts, graphics, audio, and video.
The following are the most notable generative AI use cases for retail:
Personalized Product Recommendations
71% of customers want retailers to show personalized product recommendations, and 76% are frustrated when this is not the case. Generative AI allows you to analyze large amounts of customer data such as shopping history, items added to a cart, searches, and demographics.
This level of customer behavior analysis means that you can understand your buyers' personal preferences and likings. As a result, your product recommendations are not only more personalized, meaning you have much better chances of improving customer engagement.
The best example of the application of generative AI to create personalized recommendations can be seen in none other than Amazon. The retail giant uses generative AI to analyze customers’ past purchases and searches to offer highly personalized product recommendations. As a result, the shopping experience is enhanced and customers see products that are relevant to their interests.
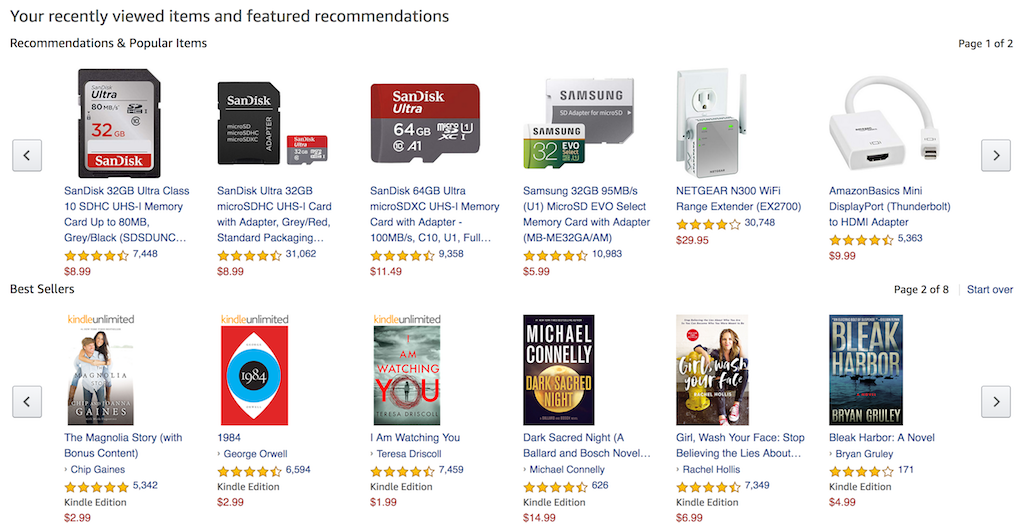
That being said, offering such recommendations is not possible without specialized software working its magic in the backend. For instance, shopdev’s very own Recommendation Engine, which is part of its next-generation operating system XStak, provides accurate and personalized suggestions to improve customer engagement and increase AOV in leading retail brands.
Virtual Try-Ons and Fittings
One of the problems customers face while shopping for clothes and other wearables online is knowing whether the size will fit perfectly. Now, retailers can offer customers immersive and interactive shopping experiences through generative models and computer vision. With generative AI, you can use conversational virtual assistants that help customers through their online shopping journeys.
Virtual try-ons and fittings with generative AI allow customers to see how the apparel and accessories, or even furniture items they buy online will fit them and their spaces. This reduces the hesitation that customers feel while shopping for wearables online and also eliminates the need to visit brick-and-mortar stores for try-ons. In this way, the use of generative AI in virtual try-ons reduces product return rates and improves customer satisfaction rates in retail.
Zolando is one of the leading fashion retail brands that has introduced a virtual try-on room for online shoppers.
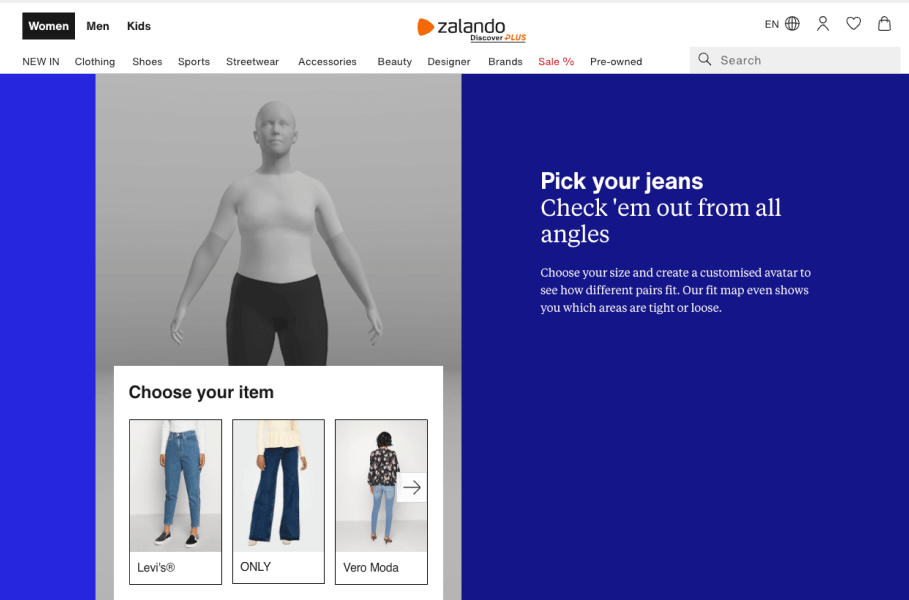
Using machine learning models, computer vision, and other AI tools, the brand’s fitting room predicts the right sizes for customers. Although the virtual try-on model is still in its infancy, more than 30,000 customers have already tried it.
Dynamic Pricing Optimization
Dynamic pricing allows retailers to get a competitive advantage and target the right customers. Both price elevations and discounts account for a higher number of sales when done smartly. Speaking of smart solutions, you don’t need to look further than generative AI to get your prices right. Generative AI factors in customer data, demand, competitor pricing, and ongoing trends to optimize prices in real time.
Not only that, but retailers are also using generative AI to create personalized discounts based on customer data analysis. This helps curate custom shopping journeys and provide customers with relevant content, ultimately helping retailers boost sales and loyalty.
One of the best examples of dynamic pricing in retail is seen in Walmart Marketplace. The renowned retailer allows sellers to adjust their prices in real-time to stay competitive and boost their profits. This is made possible by a generative AI-based Repricer tool that allows third-party sellers to take control of their own dynamic pricing strategies. These strategies consider Walmart.com prices and the prices of the given products on other eCommerce sites to set up the right prices.
AI-Powered Visual Search
Visual search is a useful feature in retail that allows customers to find the right products using images uploaded to engines like Google Lens and Pinterest Lens. Generative AI plays a major role in making visual search possible, as it analyzes and extracts important features from images to help find similar products.
When customers upload images of products they want to find, the generative AI system picks particular features from the image to enhance product discovery and shows similar products available on the market. This significantly improves the shopping experience as customers do not have to try entering different product names to find what they need.
For example, Forever 21 has adopted the visual search feature to offer better shopping experiences to its customers. The brand is an advocate for the visual way of going about product searches and purchases. It aims to use visual product discovery with AI to make online shopping more convenient.
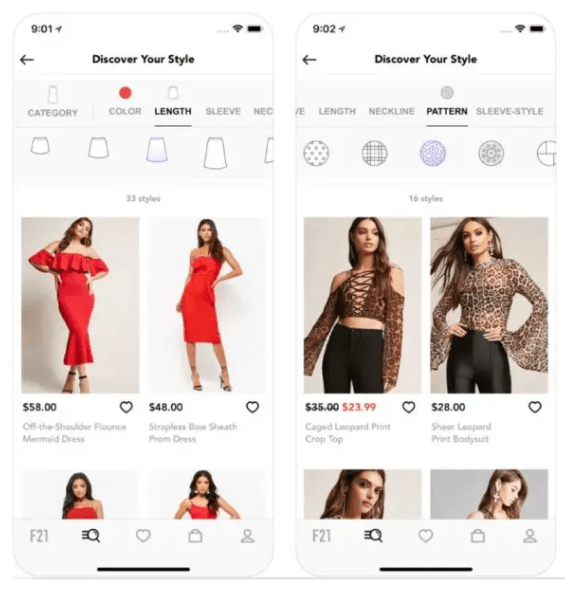
If you are thinking about incorporating a visual search feature in your retail brand, look no further. Our expert software engineers at shopdev have developed and rolled out an Image Similarity module as part of our retail operating system known as XStak. The system is helping retailers improve their brand image by enabling customers to easily find their desired products. Shoppers get instant access to product details and can make their purchases confidently.
Automated Customer Service and Chatbots
Providing customers with fast responses is key to improving satisfaction rates and gaining loyalty. Fortunately for retail businesses, generative AI can help automate customer services with the help of intelligent chatbots. AI can provide answers to customer queries based on their history and demographic information.
It also provides personalized support and recommendations with the help of conversational interfaces. In this use case, generative AI makes use of natural language processing and large language models (LLMs) to resolve customer queries as fast as possible. This helps create a flawless shopping experience and allows retailers to boost the efficiency of their customer support processes.
Retail brand American Eagle gives us an example of AI being put to use in customer support. The brand has demonstrated customer service innovation by leveraging AI to transform its in-store dressing rooms. By using computer vision and machine learning to analyze a customer’s clothing preferences, size, and skin tone, American Eagle offers them the most suitable products that are likely to fit well.
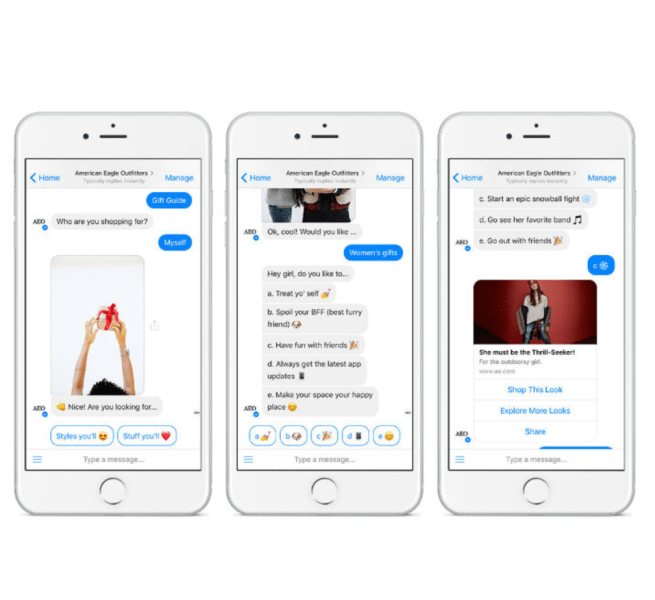
With the help of AI, the brand has significantly enhanced the shopping experience and made it highly personalized. As a result, retail customers get served in the best way possible using the best-in-breed technology.
Inventory Management and Demand Forecasting
Supply chain and shipping issues are the constant companions of every retail business. As these issues became common during the pandemic, more and more retailers have now adopted automated inventory management and demand forecasting systems. Here, generative AI helps retailers analyze sales data and manage their inventory effectively.
It forecasts trends by processing and analyzing historical data, customer demands, and competitor data to make better decisions while ordering inventory items. This helps prevent over-ordering, stockouts, and dead inventory - hence optimizing your supply chain and delivery processes.
H&M, a leading retail fashion brand, has implemented AI to improve inventory management. The AI algorithms capture data from search engines and blogs to learn about the latest fashion trends. This data clarifies how much customers are paying, what they are buying, and what kind of shopping channels they are using. This helps H&M make informed decisions about restocking popular items and smartly distributing them throughout their franchises.
Customer Sentiment Analysis
Knowing what your customers think about your brand and your products is crucial to improving sales. Sentiment analysis is one of the tactics that allows retailers to get a better understanding of how customers feel about their products. While the most basic way of performing sentiment analysis is to analyze online reviews, generative AI takes it to another level.
You can now analyze any kind of text with generative AI to understand whether your customers are demonstrating positive, negative, or neutral sentiments for your products. Furthermore, AI algorithms are now advanced enough to understand new texts apart from the data they are trained on.
As an example of a retail brand using AI for customer sentiment analysis, we look back at the biggest online retailer of them all - Amazon. The platform’s AI tool Amazon Comprehend analyzes customer sentiments from product reviews and categorizes them based on positive, negative, and neutral sentiments.
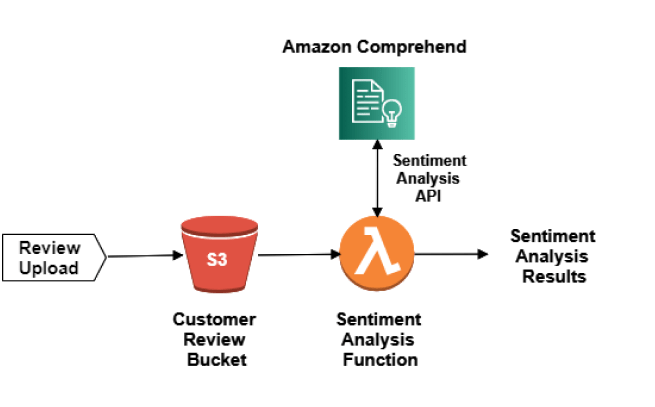
This helps Amazon identify loopholes and work on improving the products and services that generate negative sentiments. By better understanding what customers expect and want, the retail giant continues to improve its service quality.
Fraud Detection and Prevention
Last but not least, generative AI also finds a useful application in fraud detection and prevention. The retail sector is quite used to concerns of fake purchases and returns in fraudulent efforts to gain easy money. In such scenarios, generative AI algorithms can save retailers a lot of money by detecting fraudulent transactions.
Not only does AI prevent fraud from the customer end, but also identifies counterfeit products from unauthorized sellers in online marketplaces. The algorithms can pinpoint deviations from normal behavior in transactions and inconsistencies in product descriptions, hence helping prevent fraud.
Walmart demonstrates an example of AI being used for fraud prevention in retail. The retail chain has filed a patent for a machine learning system that can detect fraudulent transactions. The AI-based system is trained on past payment transfer data to detect deviations in payment patterns. It is capable of detecting a fraudulent payment transfer the moment it is initiated or received by a user.
AI Adoption and Integration Challenges For Retailers
Although generative AI is gaining popularity at a fast pace, many businesses are still facing challenges in utilizing its full potential. For instance, many startups and mid-sized retail businesses do not have the understanding and expertise required to implement AI in their systems.
Bias and Poor Quality Data
As mentioned earlier, generative AI models need to be trained on extensive, high-quality data to be able to produce accurate results. This is a challenge for retailers as they cannot guarantee that the data used to train the AI models will not be biased, unethical, or of poor quality.
Complexity of AI Models
Even when you think you can gather the right data, there is the next challenge of understanding and interpreting the generative AI model. Only AI experts can understand why a model makes a particular decision in a given scenario. For retailers, the decisions or outputs are often unexpected, making them question the reliability of AI tools.
That being said, making your mark in the competitive retail environment does require high brand visibility and exceptional customer service. In order to ensure that your brand stays on top, you need to consider these integration challenges as stepping stones towards business growth. After all, the result of successfully incorporating generative AI into your business will be a significant competitive advantage. And in retail, that is all you need.
Key Takeaways
Establishing a retail brand in 2024 requires a deep understanding of customer preferences and competitor strategies. By using generative AI, you can stay ahead of the competition and come up with not one but multiple strategies to pull more and more customers. As AI tools accelerate your business operations, you can offer your customers smooth shopping experiences that make them come back for more.
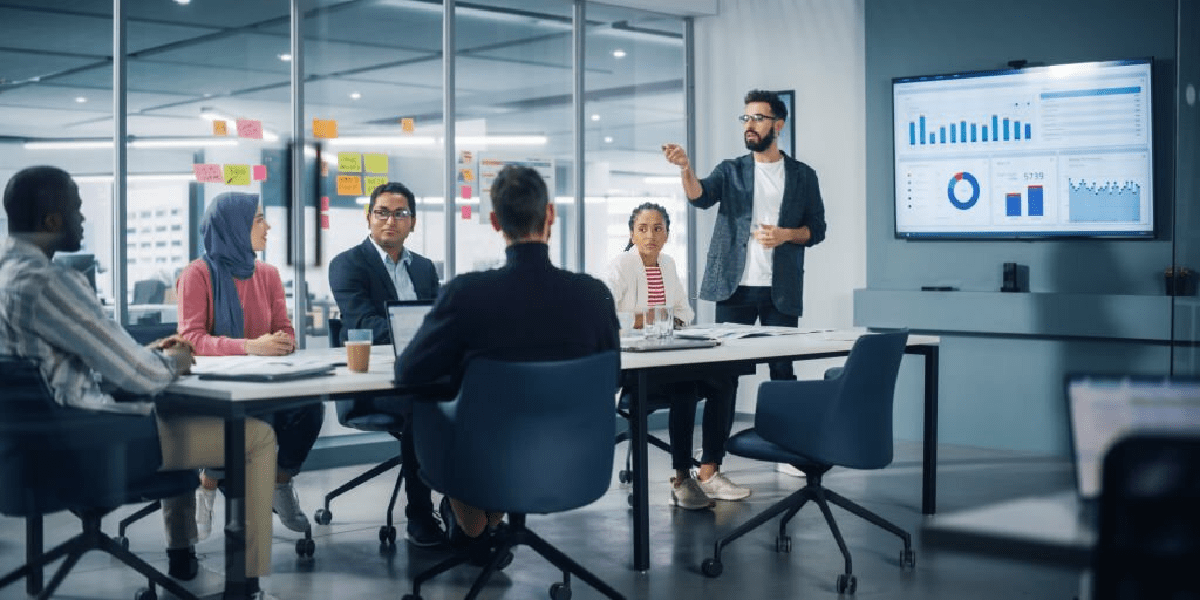
15 Generative AI Use Cases for Enterprise Businesses
In today’s tech-driven era, enterprise leaders find themselves at a crossroads where they have to deal with high-interest rates, inflation, and geopolitical concerns. They look for ways to accelerate product development and enhance service quality. One such ‘way’ is through a technological revelation you have already heard of - Generative AI.
Generative AI isn’t just the most buzz-worthy tech trend of 2023. Between 50% to 60% of all organizations have already adopted the disruptive technology.
Whether you consider customer relationship management (CRM), enterprise resource planning (ERP), product engineering, or service delivery, the applications of generative AI for enterprises just keep growing.
Generative AI Enterprise Use Cases
Generative AI is a subset of AI that goes beyond data analysis to create new and unique content. Based on patterns learned from the data they are trained with, generative AI tools like ChatGPT, DALL-E, and StyleGAN have demonstrated groundbreaking results.
In general, the enterprise use cases for generative AI revolve around automating, simplifying, and enhancing the quality of operational workflows.
Quick Automation of Project Workflows
When it comes to workflow automation, generative AI helps enterprises, especially marketing and sales-driven organizations with content creation. With automated content generation, businesses can speed up their entire digital marketing workflow. Generative AI can create a variety of engaging blog posts and ad copies, as well as personalized social media posts and product descriptions to target the right audience.
Simplify Repetitive Tasks for Employees
Regardless of the industry you pick, there are always going to be repetitive tasks that become a hurdle for innovation in enterprises. In this scenario, generative AI not only streamlines repetitive tasks for employees but also provides consistent and high-quality outputs. This means you have better customer engagement and a higher number of satisfied customers across the board.
Maintain High-Volume Production Standards
As generative AI continuously learns from the data it processes, it can interpret complex data to refine production standards. Whether you are on the factory floor or in any other part of the organization, generative AI equips you with the right tools to address quality standards in production.
How Enterprises Are Using Generative AI Today
Although the technology itself is the same for all, not all enterprises are able to use generative AI to its full potential. In some enterprises, it may be used as part of a conventional subscription-based tool to improve operations to a certain extent. In other cases, organizations are even building their own AI models to add to their tech stack.
Industries Embracing Generative AI
Let’s look at the ways different industries are utilizing the power of generative AI:
E-commerce and Retail
To understand the use of generative AI in retail, we take the example of Shopify. The eCommerce platform has now introduced a feature called Shopify Magic, which allows retailers to generate product descriptions using generative AI.
Users can input their desired tone and keyphrases for the product-related content they want to generate. Then, Shopify’s magic tool automatically generates a description based on the given parameters. In the background, of course, its generative AI working its magic.
Shopify makes the list of a handful of retail enterprises that have adopted generative AI, and even though Shopify Magic is still in its infancy, it is being well-received.
Software Development and FinTech
Where there is online shopping, there ought to be transactions. That brings us to two of the notable industries that have a high AI adoption rate - FinTech and software development.
Stripe is a renowned financial services SaaS provider that uses OpenAI’s GPT-4 to enhance its operations.
The generative AI tool allows Stripe to improve query management, summarization, documentation, and more. An internal feature called Stripe Docs is also available for users to enter their natural language queries related to documentation.
Upon submitting their query, users receive a response where Stripe’s AI summarizes and extracts important information in a human-like format. Moreover, the SaaS provider is also working with OpenAI and other generative AI companies to create smoother subscription and checkout processes for customers.
Travel and Hospitality
Here’s yet another industry that is using generative AI to improve customer experience. The travel and hospitality sector is all about ‘looking after’ the customers, and Expedia understands that.
Expedia uses the beta version of the popular generative AI tool ChatGPT for its travel planner. The AI integration allows Expedia users to ask questions and receive recommendations for travel, lodging, and other recreational activities. Not only that, but it also saves the suggested hotels and destinations using an AI-based feature. This means that customers can see what was recommended earlier and easily book their next destination.
In the context of enterprises, Expedia’s AI use case comes in handy for frequently-travelling employees. The solution streamlines the travel process and gives the employees more time for their high-priority tasks.

Generative AI Use Cases: Ethics and Compliance
Before we get into generative AI use cases, here’s a quick heads-up. With all the applications and developments in the field of generative AI, we often ignore the fact that the technology is still in its infancy. This means that there are still many unknown factors. For instance, the end-users are not aware of the way the AI models are trained, or what data is used to train them.
The fact is that generative AI models have a wide range of capabilities that can be very useful, but also very harmful when it comes to cybersecurity. Not to mention, the quickly growing skillset of generative AI models may result in them replacing skilled professionals.
Considerations for Ethical Use of Generative AI
With that said, what should enterprises do to ensure that they are ethically using generative AI? Here are a few tips to get started:
- Do not use sensitive data to train an AI model. The inputs must be depersonalized and non-sensitive
- Stay updated with current generative AI trends so that you know which tools are credible
- Set up an ethics policy for AI usage in your enterprise. It should guide internal users to use the right AI tools
- Provide training to all employees so that they stay one step ahead of AI and use it to advance in their careers.
15 Implementable Generative AI Use Cases
Code Generation, Documentation, and QA
Generative AI assists software developers and programmers by writing and completing code faster than ever before. Software teams use AI tools to write code in multiple languages and speed up their development process, hence increasing their productivity and efficiency.
Once the code is written, the most useful and quickly emerging use case of generative AI comes into play. Quality assurance is one of the most crucial phases in software development. With generative AI, it becomes as easy as implementing the right tools and entering the right prompts. AI models are capable of fixing bugs, analyze test cases, and also create various types of documentation.
Furthermore, generative AI-based coding tools are now advancing beyond the basics to help non-developers write code by using simple queries. By entering scenario-based queries in natural language, they can leverage generative AI to write code that works. This is a giant leap in the world of enterprises that are aiming at tech democratization.
Product and App Development
While writing code speeds up the process for software developers, generative AI is also being used to develop entire apps and products. From analyzing material options to proposing new designs, it has given a futuristic angle to product development. With generative AI, iterative product development teams can make design refinements in real and perform more efficiently.
Apart from assisting the development of digital products and apps, generative AI also finds use cases in semiconductor chip manufacturing and design. Not to mention, it has significantly improved customer support services by allowing companies to build chatbots based on OpenAI foundation models. Examples of such solutions include GPT-4, A121 Labs, Stability AI, and MOSTLY AI.
Blog and Social Media Content Writing
As we have seen earlier in this blog, generative AI is empowering marketing enterprises with automated content creation. Every marketing team aims to save time and resources and have a favorable time-to-market.
Generative AI is good news for marketers, as its large language models (LLMs) allow you to create unique content for blog posts and social media platforms. It also helps create engaging content for business websites and prevents you from missing out on details when writing product descriptions.
Most of these AI models allow users to provide instructions and specify the tone, voice, and other aspects of the content they want to generate. Enterprise users can even input past content written by their company and prompt the AI tool to follow the same tone. Examples of these AI tools include HubSpot Content Assistant, Phrasee, Notion AI, and Jasper.
Inbound and Outbound Marketing Communication
Apart from blog and social posts, marketing teams also need to enhance their inbound and outbound marketing campaigns. The traditional process requires employees to manually create contextualized emails and chats. These are then sent to current and prospective customers. Generative AI solutions are now automating this process by creating and sending emails.
Not only that, but generative AI can also qualify certain contacts for the next step in the enterprise’s customer relationship management (CRM) platform. Examples of AI solutions enhancing project management capabilities in enterprises include Salesforce Einstein GPT and Twain.
Graphic Design and Video Marketing
Graphic designing and video creation are also crucial to a successful digital marketing team. Generative AI helps creative designers by generating realistic animations, images, and audio that can improve graphic designing and video marketing projects.
In addition to videos and images, some generative AI service providers also offer avatars and voice synthesis features. These allow marketers to complete their video production without the need for actors, expertise, or equipment.
While the avatars and voices being offered today still require some manual input to replace real human speakers, it is still a step ahead in the right direction. Rephrase.ai, Diagram, Synthesia, and Lightricks are examples of AI solutions offering graphic design and video marketing services.
Entertainment Media Generation
Moving on, the entertainment media industry is probably the one to benefit most from high-quality and realistic AI-generated images. Generative AI finds applications in movie making, video games, virtual storytelling, VR experiences, and podcast generation.
The entertainment industry finds it convenient to use these AI tools instead of setting up cameras, editing footage, and recording audio. According to tech experts, generative AI is all set to revolutionize film content creation, script writing, and video production. As we speak, AI tools like Latitude Voyage, Plask, Charisma, and Stability AI’s Stable Diffusion are being used to create interactive non-player characters (NPCs).
Performance Management and Employee Training
Apart from enhancing operational efficiency and accelerating processes, generative AI is also being used to analyze business performance and train employees. For instance, generative AI can be used in contact centers of an enterprise to analyze call documentation.
By using sentiment analysis, generative AI can provide business owners with valuable insights. With this information, you can assess customer service quality and set up training sessions for employees. Conversational AI tools like Gong, CoachHub AIMY, and Anthropic Claude allow your employees to get performance feedback and understand areas for improvement.
Reporting and Data Analytics
One of the reasons why generative AI has quickly become a part of enterprise reporting is its ability to process massive amounts of data. When it comes to analysis of unstructured and qualitative data, nothing can match the power of generative AI. It is improving business reporting and intelligence by providing real-time data-driven insights.
Of the areas where generative AI is being used, data narratives are probably the most interesting. There are highly contextualized explanations of datasets provided by AI that are far more advanced than typical visualizations and data dashboards. Examples of such AI solutions include Narrative BI, Dremio, and SparkBeyond Discovery.
Customer Support and Customer Experience
Generative AI chatbots aren’t really a new thing. They handle customer service queries along with virtual assistants all day long. That being said, the chatbots that have been used for straightforward customer service engagements are not as advanced as generative AI.
Now, with advanced AI models, you get additional resources to provide comprehensive human-like answers without manual inputs. Enterprises that have adopted this technology in its early days are building their own customer service solutions using OpenAI’s ChatGPT and API. Forethought SupportGPT, Gridspace, and IBM Watson Assistant are some of the examples of chatbots that are enhancing customer experience in enterprises.
Pharmaceutical Drug Discovery
Generative AI isn’t just the future of the pharmaceutical industry. It is already being used to enhance the efficiency of drug discovery and design operations. Using the valuable insights provided by generative AI, scientists are creating novel molecules and discovering disordered proteins. They are also designing better drugs and predicting clinical trial results using the groundbreaking technology.
This particular enterprise use case of generative AI is expected to grow by a significant margin in the years to come. Entos, Aqemia, New Equilibrium Biosciences, and Insilico Medicine are a few examples of AI tools being used in the pharmaceutical industry.
Medical Diagnostics and Imaging
When you consider the field of medical diagnosis and imaging, generative AI is still a relatively new concept. However, its potential is growing exponentially. As AI-based image processing and generation tools become more capable of zooming into medical images, professionals can now get a better understanding of areas they haven’t seen so clearly before.
Even in its infancy, generative AI is performing medical image analysis and offering basic diagnostics. Well-known tools like ChatGPT and GPT-4 are being tested in the pathology space, with ChatGPT even passing the U.S. Medical Licensing Exam. That said, the results still need to be cross checked with medical professionals and analyzed for anomalies. Other examples of AI solutions in medical are Paige.ai and Google Med-PaLM 2.
Consumer-Friendly Synthetic Data Generation
Considering the potential security concerns that can be caused by generative AI, you may assume that this is just one of its negative aspects. But hold on - what if we could use the same factor to improve the data being generated and make it more consumer-friendly?
When you get your prompts right, generative AI will provide you exactly what you’re looking for. It can be used to create synthetic copies of data that is actually sensitive, hence allowing analysts to get what they need without being non-compliant. With these copies, data analysts and other enterprise members can create their own AI models. Examples of synthetic data generation tools include Infinity AI, Syntho Engine, Synthesis AI, and MOSTLY AI.
Smart Manufacturing and Predictive Maintenance
The manufacturing industry sees another use case of generative AI for enterprises. As it continues to transform modern manufacturing, generative AI is helping workers innovate and achieve their production goals. With predictive maintenance, generative AI models can create to-do lists and timelines to assists enterprises in improving their workflow. These models also suggest areas for improvement and simplify your process of collecting data from different parts of your production line.
Moreover, another process called inverse design also uses generative AI to analyze what’s missing in a process. Tools like Clarifai, C3 Generative AI Product Suite, and Biomatter can identify missing materials from a process and generate their replacement to suit the requirements.
Fraud Detection and Risk Management
Another area of enterprises that must not be missed is fraud detection and risk management. Although your business might get lucky without such measures, it’s always better to have AI by your side. In this use case, generative AI analyzes transaction data and identifies anomalies in the patterns. It helps in fraud detection to ensure smooth operations in finance and insurance sectors.
As of now, enterprises must invest in anomaly and fraud detection solutions that use generative AI to catch even the slightest deviation in transactional patterns. Simplifai InsuranceGPT and Docugami are the best known examples of such solutions.
Optimized Enterprise Search and Knowledge Base
Where there are enterprise tools, there needs to be documentation and a knowledge base. Generative AI is helping enterprises enhance both internal and external search capabilities. AI tools like Elasticsearch Relevance Engine, Glean, and Coveo Relevance Generative Answering not only identify enterprise resources for users but also company applications, web properties, and messaging tools.
Visitors can get a self-service by using generative AI tools incorporated into business websites and customer-facing displays. They can get answers to their brand-related questions and also get the right resources using AI chatbots.
Key Takeaways
Considering the rapid evolution of AI, it's not hard to guess that there will be even more enterprise use cases for generative AI in the days to come. As AI technology develops new capabilities, enterprises will have more opportunities to improve their operations. If you are an enterprise looking to adopt generative AI, you need to find the right model that works in your specific use case and aligns with your objectives as well as your customers.
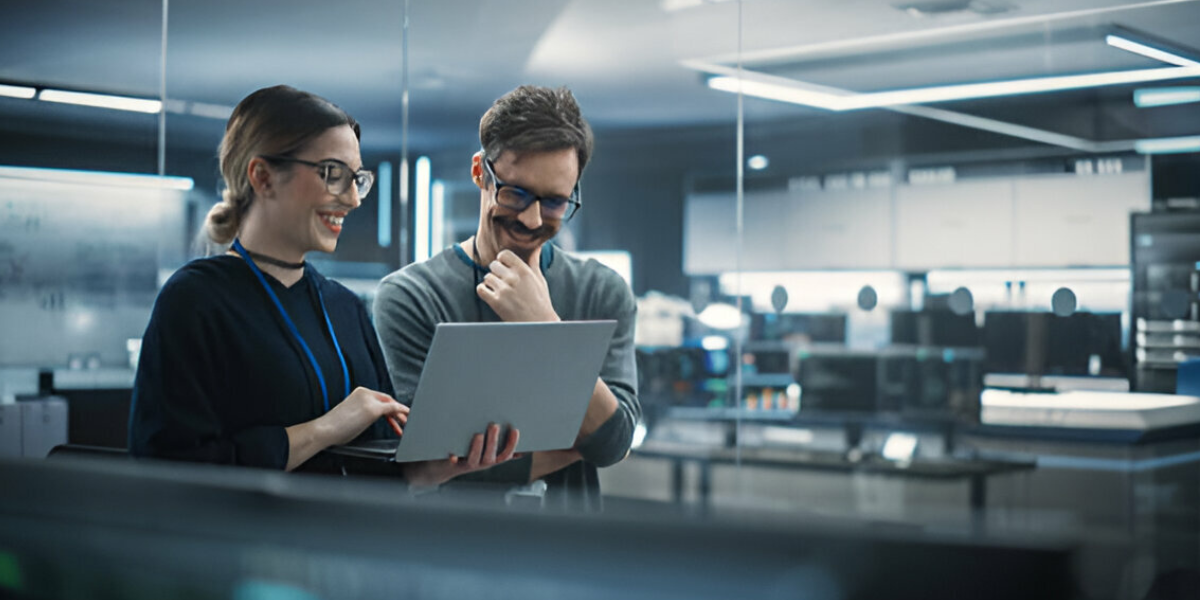
7 Mistakes to Avoid When Selecting an AI Development Company in 2024
In the fast-evolving landscape of artificial intelligence, selecting the right AI development company is more crucial than ever. As AI technologies become integral to business operations across various industries, the stakes for choosing a competent and reliable AI partner have soared. The decision you make can either propel your business forward by leveraging cutting-edge AI solutions or set you back due to mismatches in expertise and expectations.
With AI advancements rapidly progressing each year, businesses must approach the selection process with a well-informed strategy. However, common pitfalls often trip up even the most astute business leaders. From failing to conduct adequate research to overlooking crucial aspects like scalability and data security, these mistakes can lead to disappointing outcomes. In this article, we will guide you through seven critical mistakes to avoid when selecting an AI development company in 2024, ensuring that your business makes a choice that aligns with its long-term technological and operational goals.
1. Insufficient Research
The foundation of a successful partnership with an AI development company is thorough research. Yet, many businesses rush into decisions, swayed by superficial evaluations or compelling sales pitches. This oversight can lead to a poor match between your project's requirements and the chosen company's capabilities, potentially resulting in project failure.
To avoid this, it's essential to dive deep into the backgrounds of potential AI partners. Start by examining their past projects, client testimonials, and independent reviews. Understanding their track record in detail will help you gauge their success rate and reliability. Websites like Clutch or Glassdoor provide comprehensive reviews that reflect real customer experiences and can be invaluable in this phase.
Also, consider reaching out to past clients directly. This can give you unfiltered insights into the AI company’s ability to deliver on promises and handle challenges. Learning from the experiences of others can steer you away from companies with a history of projects that do not meet client expectations.
Finally, do not overlook the importance of case studies. These often contain detailed descriptions of how the company approached different challenges and the outcomes of their projects. A company that can demonstrate a history of innovative solutions and successful implementations across various scenarios is more likely to adapt and thrive in your specific context.
2. Unclear Objectives
A fundamental error many companies make when embarking on an AI project is not having clear, defined objectives. This lack of clarity can cause misalignment between your company's needs and the AI development approach, leading to outcomes that don't meet your expectations.
With 77% of companies either using or exploring AI, it's evident that businesses are increasingly dependent on AI to enhance various aspects of their operations, such as operational efficiency, customer experience, and product innovation (National University). This trend underscores the necessity of having well-defined objectives to effectively leverage AI for these purposes.
Before you start looking for an AI partner, it's crucial to outline what you want to achieve with AI. Are you looking to improve operational efficiency, enhance customer experience, or perhaps innovate a new product? Clearly articulating your goals will guide you in selecting an AI company that specializes in the specific areas you need.
For instance, if your goal is to integrate AI into customer service operations, you would look for a company with proven expertise in natural language processing and chatbot development. This specificity will narrow down your options and increase the chances of success.
It's also important to communicate these objectives clearly when engaging with potential partners. Clear communication ensures that the AI development fully understands your expectations and can provide a tailored solution that meets your specific requirements.
To facilitate this, consider creating a detailed project brief that includes not only your goals but also any constraints, such as budget and timeline. Share this document with potential AI companies during your initial discussions to ensure everyone is on the same page from the start.
Defining and communicating your objectives is not just about avoiding missteps; it's about paving a path to a successful AI implementation that is aligned with your strategic business goals.
3. Neglecting Industry Expertise
Choosing an AI development software solution company without considering its expertise in your specific industry is a critical oversight. Each industry presents unique challenges and regulatory requirements that require specialized knowledge. A company proficient in AI for finance may not be as adept in AI applications for healthcare, for example.
When selecting your AI development partner, it’s crucial to verify their experience and success in projects within your industry. This can involve reviewing case studies that highlight their work in similar sectors or requesting references from past clients in your industry.
Furthermore, consider the specific challenges your industry faces. For instance, if you are in healthcare, data privacy is a paramount concern, governed by strict regulations like HIPAA in the U.S. An AI company with a deep understanding of these regulations will be better equipped to ensure compliance and secure handling of sensitive data.
Inquiring about the technology stack the company uses is also essential, as some technologies might be more suited to particular industries than others. For example, a company using advanced neural networks would be preferable for image recognition tasks commonly required in the security or automotive industries.
To effectively assess a company’s industry expertise, prepare questions related to their past projects, the challenges they faced, and the solutions they implemented. This will help you gauge whether they have the practical experience and in-depth knowledge necessary to handle the specific needs of your industry.
4. Overlooking Data Security
Ignoring data security when selecting an AI development solution can expose your business to significant risks. Data breaches can lead to financial loss, legal repercussions, and severe damage to your company's reputation. It's crucial to ensure that the AI company you choose has robust security measures in place.
Start by inquiring about the data security practices the AI company follows. This includes understanding their data encryption methods, how they manage data access, and their compliance with relevant data protection regulations, such as GDPR or HIPAA. A reputable AI company should be transparent about their security protocols and willing to discuss how they will protect your data.
Additionally, consider the security of the technology stack they use. The programming languages, frameworks, and infrastructure should all meet the highest security standards to prevent vulnerabilities. For example, ask if they employ secure coding practices and regular security audits to detect and mitigate potential security issues.
It’s also wise to discuss how the AI company handles data breaches, should they occur. Understanding their incident response plan can give you confidence that they are prepared to act swiftly and effectively to minimize any damage.
To assess their commitment to data security, request to see certifications or attestations such as ISO 27001 or SOC 2. These certifications demonstrate a level of dedication to maintaining high-security standards.
5. Ignoring Scalability
Scalability is often underestimated during the selection of an AI development solution. As your business grows, so will your demands for AI capabilities. Selecting a partner that can scale solutions to match your evolving needs is essential for long-term success.
To ensure scalability, assess the potential AI company’s infrastructure and technology stack. Ask about their experience handling increasing volumes of data and user engagement. A company that has successfully scaled projects similar to yours is likely to replicate that success with your project.
Moreover, discuss future growth prospects during initial meetings. Understand how the company plans to accommodate your growth, such as adding more resources or utilizing cloud technologies that offer flexibility. This discussion will give you insight into their capacity for adaptation and innovation.
It's also crucial to consider the scalability of the AI models themselves. Inquire about the AI company’s approach to updating and refining AI models as more data becomes available and as business needs change. The ability to continually improve and adapt AI models is key to maintaining the relevance and effectiveness of AI solutions.
Lastly, review case studies or testimonials that demonstrate the company’s track record with scalable AI projects. These will provide concrete examples of their ability to expand and adapt their services in response to client growth.
6. Inadequate Post-Development Support
Neglecting the importance of post-development support is a common oversight that can undermine the long-term success of AI projects. Once an AI solution is deployed, it requires continuous maintenance, updates, and support to ensure its effectiveness and relevance over time.
When selecting an AI development company, evaluate their commitment to post-launch support. This includes regular updates to the software to incorporate the latest AI advancements and patches for any security vulnerabilities that may arise. A company that offers comprehensive support will help ensure your AI system remains robust against evolving threats and technologies.
It's also important to discuss the specifics of the support agreement. Determine what types of support are available—such as 24/7 customer service, on-demand technical assistance, and routine check-ups. These services are crucial for quickly resolving any issues that might arise, thereby minimizing downtime and maintaining operational continuity.
Furthermore, ask about the company's procedures for handling feedback and implementing changes. An AI development that actively seeks and responds to client feedback demonstrates a dedication to service quality and continuous improvement.
Finally, consider the scalability of support services. As your business grows, your support needs may increase. The right AI development partner will offer flexible support options that can be scaled to match your evolving requirements.
7. Poor Communication and Collaboration
Effective communication and collaboration are pivotal for the success of any AI project, yet they are often overlooked when selecting an AI development. Poor communication can lead to misunderstandings, misaligned objectives, and project failures.
When evaluating potential AI partners, prioritize those who demonstrate strong communication skills right from the initial interactions. Assess their responsiveness to your inquiries and the clarity of their communication. This can be an indicator of how they will engage throughout the project lifecycle.
During your discussions, observe whether the AI company seeks to understand your business context and objectives thoroughly. A partner who asks insightful questions and proposes ideas that align with your vision is likely to be a collaborative and communicative team player.
It's also important to consider the company's tools and processes for project management and communication. Do they use established platforms like Slack, Microsoft Teams, or Asana? Are their processes structured to ensure regular updates and feedback loops? These tools and processes are vital for maintaining clear and continuous communication.
Moreover, inquire about the involvement of project managers or points of contact who will manage the relationship. Having a dedicated person or team to handle your project ensures that there is always someone available to address your concerns and coordinate between different teams.
Finally, discuss the company's approach to conflict resolution and problem-solving. Understanding how they handle challenges and setbacks can provide insight into their ability to maintain a productive working relationship even under pressure.
Conclusion
Selecting the right AI development company is a critical decision that can significantly influence your project's success. Rushing the selection process without adequate research, or partnering with a firm that lacks the necessary industry expertise, can derail your goals. Clearly defining your objectives, prioritizing data security, and ensuring scalability are essential steps.
Equally important is securing comprehensive post-development support. Regular updates, technical assistance, and adapting to your growing business needs will ensure your AI solution remains relevant. Effective communication and collaboration, facilitated through strong project management and regular feedback loops, are vital for seamless project execution.
Avoiding these seven mistakes will not only help your business choose an AI development partner that aligns with your goals, but will also set the foundation for a long-term, successful partnership. By being diligent in your selection process, you can safeguard your investment and fully harness the transformative power of AI.
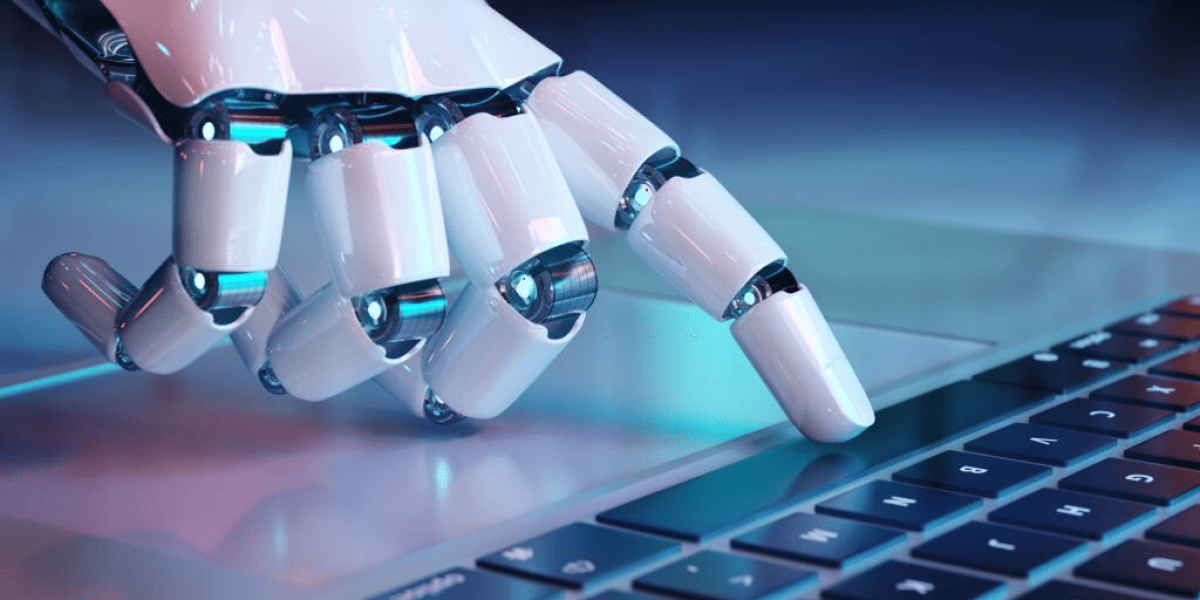
7 Steps to Build a Generative AI Solution from Scratch in 2024
Generative AI is a revolutionary advancement in the tech world that has taken businesses and decision-makers by storm. With everyone using AI models like ChatGPT and Stable Diffusion to speed up development processes, it's safe to say that generative AI is here to stay.
Jasper, one of the successful generative AI tools, has raised $125 million at a valuation of $1.5 billion by assisting businesses with copywriting. Similarly, Stability AI has made $101 million with a valuation of $1 billion. But the most significant achievement is that of OpenAI, which generated over $1 billion from Microsoft at a valuation of $25 billion in 2019.
Clearly, the scope for generative AI solutions is broadening. Read on to learn everything you need to know about how to build a generative AI solution from scratch.
What is Generative AI?
Back in the 1950s, researchers first explored the basic principles of artificial intelligence and generative AI. The initial focus was on simple neural networks and rule-based models to mimic human decision-making.
On the surface, generative AI is an exciting new development where deep learning algorithms are used to create content, graphics, music, and more. Large datasets are used as input for deep learning algorithms that can generate unique content. What makes generative AI special is its ability to generate entirely new and unique outputs, unlike traditional AI which only delivers pre-programmed outputs.
When trained, the AI algorithms learn patterns from given inputs, store them, and utilize them to create unique content for similar inputs. Even though it looks easy, generative AI becomes quite complicated when you dig deeper. For instance, there are various components like transformers, generative adversarial networks (GANs), and variational auto-encoders behind the functionality of generative AI.
Examples of transformers include ChatGPT, LaMDA, Wu-Dao, and GPT-3. Transformers are trained to process natural language and images, as well as learn classification tasks and generate texts. These techniques are designed to mimic cognitive attention and understand the difference between varying inputs.
What Can Generative AI Do?
Generative AI is helping businesses worldwide by generating content in the form of text, images, and videos. This means voice-over and background music in videos are not a problem anymore. Generative AI is also used in data augmentation to boost the accuracy of predictive machine-learning models. As a result, retailers can offer highly personalized experiences to customers.
The following are some of the diverse application areas of generative AI across various industries:
Art and Creativity
The new, state-of-the-art algorithms of generative AI enable you to convert any ordinary picture into an art piece. You can guide the AI tool to add your unique style, features, and other favorite items. Even when you provide the generative AI tool with a rough sketch of the image you want to create, it generates photorealistic masterpieces that go beyond your expectations. Not only that, but the AI can also imitate specific styles of a given human artist, which allows you to create art like never before.
Content Generation
When it comes to automating content creation, you don’t need to look further than generative AI. It has provided marketers with the ideal solution to save time and resources. With automated content generation, you can achieve faster time-to-market and create a variety of campaigns. For instance, you can create social media posts and blog articles, and even design email campaigns.
AI tools like ChatGPT, Jasper, Copy.ai, and Writesonic have accelerated the process of content generation. In some cases, marketers can personalize and refine drafts generated by AI models. Not only that but generative AI can also be used to update existing content with valuable inputs and improvements.
Natural Language Processing
Generative AI is commonly used in natural language processing to create social media posts and news articles. Various natural language processing techniques are used to generate the text for such posts. These techniques includes transforming raw characters like words, letters, and punctuation into sentences. The resulting entities and actions are represented in the form of vectors through multiple encoding mechanisms.
Image Synthesis
Today’s businesses want to represent their products and services with realistic graphics and lifelike images. Here, AI algorithms assist businesses with accurate image synthesis. You can eliminate misleading aspects from your images and fill in the missing elements to portray your products in the best way.
Medical Imaging
That’s not all - AI tools can also convert low-resolution images into high-resolution images, making them look like they were captured by professional photographers. With generative AI by your side, your images are transformed into clear and detailed masterpieces. This is particularly useful in medical imaging analysis, where accuracy is of utmost importance.
Generative AI is used in medical imaging for specific tasks like image segmentation, anomaly detection, and prediction of patient outcomes.
Anomaly Detection
Business owners need to go through large amounts of data to understand patterns in order to make informed decisions. In this case, generative AI helps detect anomalies in a series of data. Assuming a certain range of data based on historical trends, AI can point out values that fall outside that threshold and happen less frequently.
How Generative AI is Changing Industries
The world knows generative AI as a buzzword because of its impact on different industries. It has quickly transformed business operations and is creating new opportunities across the board. Apart from creating novel, unique content and making way for innovations, generative AI is changing the way processes are carried out.
Here are some examples of how generative AI is transforming various sectors:
Entertainment and Media
Two of the many benefits of generative AI are democratized content creation and simplified management. In the entertainment industry, AI helps accelerate research tasks and allows filmmakers to improve the efficiency of their post-production operations. The data processing power of AI makes video libraries more searchable, making life easier for broadcasters. For this reason, the market size of generative AI is expected to grow to $12,077 Million in 2032 at a CAGR of 26.7%
Healthcare
Whether it is medical diagnosis, imaging, or analysis of a large number of patient records, AI is always good news for the healthcare industry. You can now leverage AI-based disease prediction and diagnosis to improve healthcare operations. Generative AI monitors various factors like lifestyle risk factors, medical imaging, patient health data, environmental factors, and genetic data to provide accurate results. Moreover, statistics show that 27.5 percent of health systems currently use conversational AI, with a further 72.5 percent considering it for future use.
Marketing and Advertising
Until a few years ago, marketing and advertising agencies required graphic designers and content creators to create their campaigns. But now, AI is being used by 53% of IT companies for marketing and advertising. With generative AI, this process is a matter of a few prompts. You can prompt AI tools to create unique and original graphics for blog posts, social media posts, and more.
Gaming
Game development is a complex and time-consuming process that involves various tasks. Like other industries, generative AI becomes useful in game development by automating complex tasks. These include creating assets, levels, and generating dialogues. By utilizing generative AI to handle these tasks, game developers are able to unlock creativity and use the extra time to work on the strategic aspects of their development cycle. Generative AI in the gaming sector is expected to achieve the highest market value with a valuation of $7,105 Million by 2032.
Manufacturing and Design
Now here’s an industry that focuses on optimized production and quality controls along with great customer service. By processing raw data and helping in the production of valuable products, generative AI significantly improves manufacture and design operations. According to Gartner, by 2025, generative AI will account for 10% of all data produced, up from less than 1% today. It helps enhance customer service, streamline production processes, and also improves quality control procedures.
The Right Tech Stack to Build a Generative AI Solution
Before getting into the process of building your own generative AI solution, you need to know the right tools and technologies to use. The following is a comprehensive list of the tech stack you will need to use:
7 Steps to Build a Generative AI Solution from Scratch
In order to build a generative AI solution, you need to develop a comprehensive understanding of its purpose and the problem it will solve. The process involves creating and training AI models to generate unique outputs depending on varying inputs.
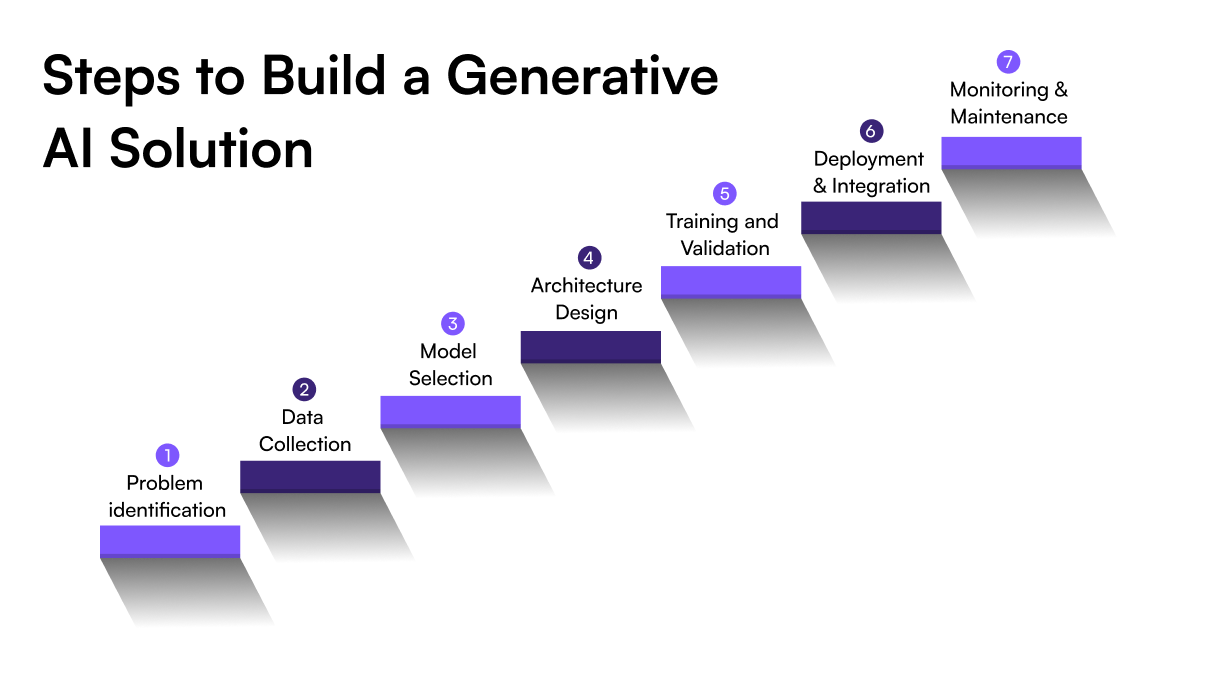
As today’s generative AI solutions are capable of optimizing and improving operations, building a satisfactory solution requires you to follow a series of steps.
Problem Identification
Since you are dealing with a lot of computing power in generative AI, you need to be sure that they are designed to solve the right problem. The first step in building your generative AI solution from scratch is to identify a set of problems to solve. For instance, marketing agencies need various types of content for their blog and social media platforms. In this case, your AI solution needs to be built around natural language processing, neural networks, and generative pre-trained transformers. In other cases such as video and audio generation, you will need to adopt a different approach.
Data Collection
Data collection is one of the important steps in the prototyping phase of generative AI development. Since the training of your generative AI solution depends on data, you need to consider certain technical details. These include:
- Identifying the right data sources
- Ensuring that high quality, relevant, and diverse data is collected
- Labeling the data if needed using crowdsourcing, semi-supervised learning, or active learning
- Preprocessing data through data augmentation, tokenization, or normalization before it is fed to the AI model
- Splitting the data into separate categories for training, validation, and testing
- Storing the data in data warehouses, distributed file systems, or cloud storage depending on the scenario.
Model Selection
Based on the defined problem that the AI solution is intended to solve, you need to select the right model and tech stack to be used in the development. To be able to choose the right model, you need to categorize the problem and determine the right algorithms to solve it.
For instance, if you’re working with input categorization, you need to select the supervised learning model. For imaging systems that can differentiate objects from images, you can work with OpenCV. On the other hand, you can also use Jupyter Notebook to allow seamless collaborations between machine learning engineers, developers, and data scientists.
Architecture Design
In order to ensure that your generative AI solution can process large amounts of data and handle high traffic, you need to base it on a robust and scalable architecture. The common purpose of all generative AI solutions is to maintain high performance, reliability, and availability in all situations. The best practices to build the right architecture are as follows:
- Break down the AI solution into small, manageable components through microservices architecture
- Adopt a modular design where each module performs its specific function
- Incorporate message queues to efficiently manage communication between different components of the AI solution
- Implement load balancing to ensure equal traffic distribution across multiple servers and get peak performance
- Implement caching to reduce backend requests by storing frequently accessed data
- Configure hyperparameters of the generative AI model to set number of layers, learning rate, batch size, regularization techniques, dropout rate, etc.
Training and Validation
In the training phase, you feed the AI model with datasets you previously collected and categorized. With these datasets, the generative AI model learns patterns that are later used to solve problems and create content. In order to train generative AI models, you need to use self-supervised learning and semi-supervised machine learning methods. Although the process is machine dominant, you need to add the human touch to fine-tune and set the AI model’s accuracy and align it with your business objectives.
Deployment and Integration
While deploying your AI solution, you need to be sure of its reliability, scalability, and maintainability. This is particularly important to eliminate errors and failures and ensure the smooth functioning of your generative AI solution. Here’s what you need to consider in the deployment and integration phase:
- Choose the right deployment environment by setting up on-premise infrastructure, cloud computing systems, or hybrid solutions depending on the requirements.
- Implement the right hardware and software for installing operating systems, setting up databases, and configuring servers.
- Set up dependencies like libraries, frameworks, and packages required to run your AI solution.
- Use tools like Jenkins, Travis CI, or GitLab to set up continuous integration and deployment (CI/CD) pipelines.
Monitoring and Maintenance
The last step of the process holds equal significance. Upon deployment, you need to constantly provide support and maintenance while evaluating how the AI solution performs in real-life use cases. The key is to identify bottlenecks and failures in order to fix and improve the solution. Upon further testing, you can refine your AI solution by studying user feedback and performance benchmarks.
Pro Tips for Crafting Effective Generative AI Solutions
Even when you follow the best practices to build your AI solution, there are always going to be some unexpected issues and challenges along the way. Based on research in the field of generative AI, here are a few pro tips that can be helpful.
- Apart from identifying a problem to solve, you should also identify specific business processes that can be enhanced by using generative AI. Focus on small changes with meaningful outcomes.
- Ensure data protection to provide users the confidence to use your generative AI solution and stay compliant with international regulations. Use data encryption to reduce risks of data leaks.
- Instead of spending big bucks on training AI software like ChatGPT, you should build smaller, more targeted AI models. These can be trained to solve business problems unlike ChatGPT.
- Since the quality of data matters, your focus should be on preparing the data for training the AI model. Try to eliminate under-generalization and bias.
- Regardless of how well you train generative AI, it can never be perfect. Therefore, you need to inform your users about the limitations of your AI solution.
Costs of Generative AI Solution Development
Depending on the type, complexity, and size of the application you are trying to build, generative AI development becomes quite a costly process. This means you can expect a bill between $10,000 and $500,000. The most notable costs include training the AI model and deploying it. The software cost itself is between $35,000 to $120,000, while maintenance costs can go beyond $320,000 per year.
That’s not all, you also need to invest in specialized hardware and cloud storage to get the right computing power and storage capacity. Depending on the complexity of your solution, this can cost you between $100 and $15,000 a month.
FAQs
Can I make my own generative AI?
You can create your own generative AI by adopting, modifying, and building the models you require. However, the process is quite complex and you need an increasing number of resources as you continue building your generative AI. You also need to have technical expertise in the fields of natural language processing, machine learning, and computer vision.
How do I start generative AI?
First of all, you need to understand the different generative AI models available in the market. You should consider the unique features and applications of GANs, autoregressive models, and VAEs. You need to gather and prepare the data you want to work with and choose the type of AI architecture to use. Then you need to train your AI model and evaluate, optimize, and test it in a real-world environment.
Does generative AI use NLP?
Yes, generative AI leverages natural language processing to create new outputs or content for each input. The use of NLP means that you can also generate all types of content including text, images, audio, and video.
Is generative AI free to use?
There are free versions of generative AI tools like ChatGPT 3.0 and DALL-E 2. These are great for beginners who want to try generating content using AI. AI tools by OpenAI allow you to generate content by simply creating an account on their website and entering your prompts in the search bar.
Key Takeaways
In the era of artificial intelligence, businesses are being driven by generative tools that enhance operational efficiency and also help target the right customers. With businesses adopting generative AI solutions, there really is no limit to what can be achieved in the near future. We can expect unprecedented business growth with the help of efficient processes, ease of use, and the automation of complex operations. Based on the steps discussed in this blog, you can build your generative AI solution and embrace its transformative power in your business use cases.
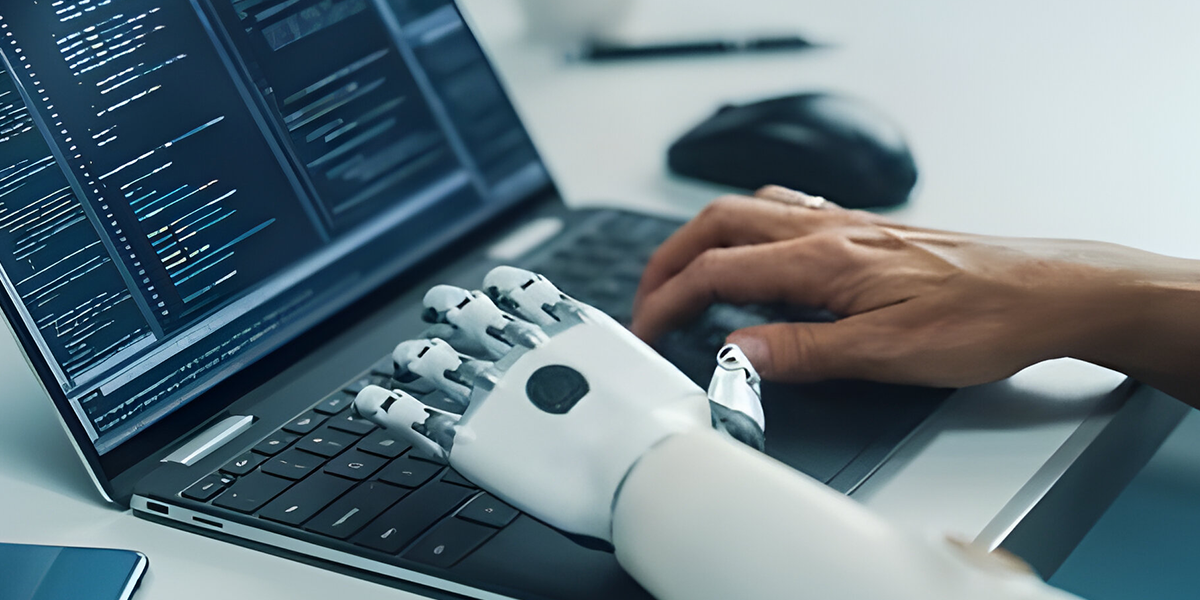
The Ultimate Guide to AI App Development Using Generative AI
App development has come a long way in recent years. As AI pushes the boundaries of innovation in software development, generative AI app development has become one of today's biggest buzzwords. In fact, it has the potential to boost business profits by up to $4.4 trillion a year.
AI changes how we conceive and build our apps by unlocking unimaginable possibilities. Using large language models, developers can create intuitive in-app experiences. You also get to personalize user experiences with enhanced creativity.
Read on to find out how the ground-breaking AI technology enhances app development.
How Generative AI differs from other AI technologies
AI has been here for quite some time in the form of machine learning and computer vision. However, these technologies are limited to analyzing and classifying datasets. Generative AI is capable of creating new content based on existing data. This can be in code, text, images, video, music, and more.
Generative AI is currently being used for the development of innovative apps in different industries.
Generative AI can be used to generate code for mobile apps, which can save developers a lot of time and effort. For example, DhiWise has introduced the new feature “WiseGPT” which analyzes the entire codebase to produce personalized production-ready code without writing prompts. The other is the “GitHub Copilot” tool that can generate code snippets and even entire functions based on natural language prompts.
The Role of Generative AI in App Development
Generative AI offers an unparalleled ability to test apps for bugs and errors. AI-based systems not only identify security risks but they can also be used to write code for a wide range of applications.
Enhancing user experience with Generative AI
When it comes to online websites and applications, user experience is crucial. You need to have seamless transitions between different responsive, user-friendly pages. Here, generative AI can automate repetitive tasks in app development.
As a result, it can solve problems without human input. You get an exceptional user experience with a bug-free application that doesn’t require any expert developer insights. That said, the effectiveness of generative AI apps depends on how well you train them.
Personalization and customization through AI
Personalized content is the way to go in the modern world. And nothing creates personalized and customized content like generative AI. By analyzing user history including search and preferences, AI creates personalized recommendations. This enhances the user experience by creating more opportunities for customer engagement.
Generating content and creative elements automatically
When humans work in cross-functional teams, the output is great. Imagine AI doing the same, except with much higher efficiency. Generative AI raises the bar of creativity and innovation. It produces content that would take us too much time to create. Plus, we would need to use various methods and tools to come close.
Automated content generation takes app development to a whole new level. You can now uncover new concepts before even creating the application. Using novel patterns, styles, and combinations, AI allows you to create perfection.
Improving app development with predictive models
Predictive analytics is a field of AI that allows developers to optimize app functionalities. By factoring in the time, effort, and cost of app development, it provides a clear pathway. You can easily identify potential risks and hurdles. It also allows you to eliminate bottlenecks to enhance the functionality of your application. These measures reduce the likelihood of issues arising during the development life cycle.
Getting Started with Generative AI App Development
Before getting started with generative AI app development, you need to know its basics. The best practices allow you to be more productive in the process by making it smooth. Whether it's your first time or you’ve been working with AI, here are the steps for app development with generative AI:
Gather high-quality data
No matter how powerful an AI tool is, it still needs to be trained using existing data. The quality of this data determines how well generative AI will perform. Therefore, the first step is to gather error-free and well-structured data. Make sure it does not have any inconsistencies or biases. And of course, it should be relevant.
Training your AI model using high-quality data yields accurate outputs. This makes app development with generative AI worth your while. And your app performs the intended functions with maximum effectiveness.
Choose the right tools and frameworks
Choosing the right algorithms and AI models is among the critical aspects of using generative AI. You need to ensure that you choose the right one based on your specific use case. For example, if you want to generate natural language texts, you can work with algorithms like GPT-4. But if you want images as output, you need a deep learning model like GANs. If you make the right decision, your generative AI app will perform effectively and yield quality outputs.
Understand the data requirements for training AI models
Moving on, you need to ensure that your AI model operates at maximum efficiency. For this, technical know-how of data requirements is crucial. You can adjust the AI’s learning rate, batch size, and epochs, and even regularize it. Here,
- Batch size means how much data it can process at a time.
- Epochs mean the number of times it learns from the data.
- Regularizing means you can prevent it from overthinking or overprocessing data.
Fine-tuning your AI models results in high efficiency of the application.
Building Your First Generative AI App
Building a generative AI app is a complex process that requires you to follow a unique set of steps. Here’s how you can get started with your first app:

Selecting and preparing your dataset
The process of developing an AI app starts with data. You need to identify the right sources to extract the datasets you’ll be using. This can be done using databases, web scraping, sensor outputs, and APIs depending on your requirements. This is a crucial step as the quality of the data directly affects the performance of your AI model.
Apart from data sourcing, you also need to pay attention to data diversity. The more diverse your data, the better the AI will perform. Its outputs will be more unique if you train it with different scenarios, modalities, and environments.
Training your Generative AI model
This is the step where your efforts shine through. Using the data you’ve gathered and cleaned, you need to train your generative AI model. You’ll be using deep learning and neural networks to feed the prepared data to the AI. It then learns to identify and emulate data patterns.
When the initial model is sufficiently trained, you can proceed to fine-tune the AI. Here, you can go deeper into specific tasks to generate the desired outputs. For instance, you can fine-tune your AI model to create poetry. You’ll have to train it on an extensive dataset of poetic content. Here, you can also deploy techniques like differential learning rates. This process takes different layers of the AI through different learning rates.
Integrate the AI model into your app
Once your AI model is ready to go, the next step is to integrate it into your app. For this, you need to develop APIs or connectors. APIs act as a bridge that fills the gap between your app and the generative AI model. Then, you need to design workflows to ensure that the data generated by AI is integrated into your existing processes. Frameworks like FastAPI and Flask are commonly used to deploy APIs with AI models.
Testing and optimizing app performance
Using real-world conditions and comprehensive testing techniques, you need to test the AI app performance. This step helps you determine whether your training in the AI model has been fruitful.
Testing an AI model for performance requires validation and data metrics. With metrics like the ROC curve, AUC score, and error analysis, you need to assess the quality of the AI model's outputs. Moreover, you can compare the outputs with benchmark models to see where your AI model falls behind in terms of accuracy and performance.
Advanced Techniques in Generative AI App Development
Improving Model Accuracy and Efficiency
An AI model is like a work in progress. You can keep making changes and updates to tweak its performance. In the case of app development, you can improve your AI model with the following techniques:
- Retrain the model on better data: You can use newer, more accurate data to retrain your AI. This helps improve performance as the outputs are based on the data being fed into the model.
- Change the way you deploy the model: Sometimes an AI model can struggle to perform well if it isn’t hosted on the right platform or connected to the right hardware.
- Source code enhancement: Some applications are just poorly designed and implemented right from the start. You need to review the source code to make the necessary improvements.
Scale your Generative AI app for a larger audience
Like any other tech solution, AI applications also need to be scalable for future use. Scalability challenges are common in AI app development. For instance, the size of datasets used to train the AI model, the sources used, and the deployment strategy need to be considered.
To make your generative AI app scalable, you need to:
- Ensure that you standardize the development and deployment processes
- Dedicate team efforts to tasks that they know best
- Utilize tools that support creativity, efficiency, and security
- Use development methods that allow updates for the future data
Ethical Considerations and Best Practices
For quite some time now, ethical concerns have been raised against generative AI. The most notable concerns like bias and racism were seen when OpenAI’s ChatGPT was first released. For you generative AI app development, you need to consider the following best practices:
- Bias check: Your AI application needs to be checked for unwanted biases that it may have picked up while training on different datasets.
- Fairness: Your AI model must not discriminate or generate biased outputs that negatively impact different user groups
- Anonymization: You need to take care of data privacy when user data is involved. So its important to anonymize your inputs and outputs at all times
Ensure privacy and security in AI-powered apps
Speaking of privacy, there are also reports of concerns related to data encryption. You need to monitor your app’s performance to identify vulnerabilities. Cyber attackers try to take advantage of the same AI capabilities in a negative way. For this reason, you must never include credentials and tokens in the code.
Apart from data encryption and authentication mechanisms, you must implement ongoing vigilance systems to avoid security breaches.
- Create ethical usage guidelines for your AI app.
- Use techniques like adversarial training to restrict bias
- Routinely monitor your AI app’s performance
- Identify and remove fake content, news, and images.
Best practices for transparent and responsible AI usage
Once the ethical and privacy concerns are addressed, you also need to consider transparency in AI usage. For this purpose, you need to implement the following best practices:
- Documentation: Create detailed descriptions of the AI models’s capabilities, its limitations, and how it is expected to perform.
- Feedback loops: You need to have open channels to discuss and mitigate any concerns and questions arising from stakeholders.
- Anomaly detection: Create systems to identify and take care of unintended behaviours or harmful outputs of the AI app.
- Guidelines and policies: The guidelines and policies for AI use must be updated each time a new feature is added or whenever the AI is retrained.
Navigate legal considerations in AI app development
In today’s day and age, the last thing you would want is a non-compliant AI solution. When it comes to legal considerations, you need to look at the following factors:
Copyrights: Businesses have started to use AI applications to create copyright content. As copyrights only protect content created by humans, this causes legal concerns. You need to ensure that your generative AI app cannot be used to create copyright content.
Confidentiality: Similarly, users and businesses are using AI tools for processes that involve personal information. To avoid legal consequences, you need to ensure that the personal data is either anonymized or used safely.
GDPR compliance: According to the GDPR, you need to define the data subjects and the purpose for gathering data. If you are unable to define the purpose, you cannot proceed with the training of your AI models.
The Future of Generative AI in App Development
AI technologies are developing at an alarming rate. Here is what the future holds for AI-based app development:
Emerging trends and technologies in Generative AI
Based on OpenAI’s latest update called GPT-4 Turbo, the system has two core improvements. One is its ability to ‘pull from a newer database of information’. And the second is its promise ‘to follow instructions better’. This gives us an idea of how fast AI is growing and improving.
AI is already working with features we expected it to perform yesterday. So it’s safe to say that the future is already here. AI can now analyze extensive documents and provide you with a summary. This is because it can now support up to 128,000 tokens of context.
That’s not all, as GPT4 Turbo now also has new API assistants. These are aimed at simplifying the creation of AI-driven apps. You will get capabilities like a Code Interpreter, Retrieval, and improved function calling. For app developers, these features are absolute game-changers.
Predictions for the future impact of Generative AI on app development
According to Forrester, ‘generative AI is accelerating the pace of innovation’. If there is going to be a challenge, its going to be navigating the risks of AI. This is because of the exponential rate at which AI is learning. That said, there is good news too. AI will unlock higher levels of productivity in the years to come.
- By 2026, generative AI design will automate 60% of the design effort for developing new websites and mobile apps.
- By 2027, 15% of applications will be automatically generated by AI without human input.
The same research suggests that by next year,
- ‘40% of enterprise applications will have embedded conversational AI, up from less than 5% in 2020’.
- By 2025 ‘30% of enterprises will have implemented an AI-augmented development and testing strategy, up from 5% in 2021.’
Key Takeaways
In summary, generative AI has tremendous potential to accelerate application development. It suggests code, identifies bugs, and even refactors lines of code. You can command it to write simple applications to speed up app development. Not only that but you can also perform quality checks of your existing code.
Combined with the innovative ideas brought forth by generative AI, you can achieve higher customer satisfaction. The best part is that generative AI applications are built without ever gathering any requirements.
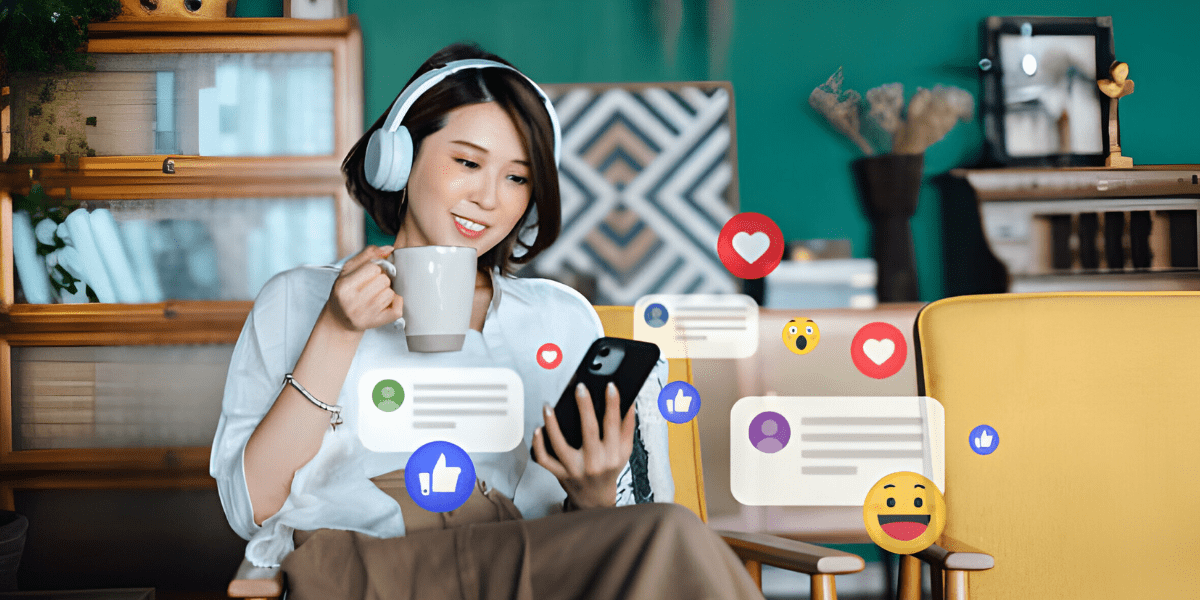
AI in Digital Marketing: Effective Use Cases
Marketing is becoming a vital aspect of any company's business domain. It allows the company to thrive and expand. Without the right marketing strategy and roadmap, it would be almost impossible to attract and engage customers, which can cause a notable impact on the growth and even decline the revenue streams. Therefore, it is crucial to stick with the contemporary marketing trends to stay ahead of the rivals and perform better.
Modern times have brought incredible evolution to the marketing industry. The Internet has opened up many new opportunity corridors, and people are now focusing more on digital marketing than conventional marketing practices. In fact, digital marketing occupies almost half of the total marketing shares. One of the most notable advancements in digital marketing is integrating artificial intelligence and machine learning in marketing practices to ensure rational decision-making and smart effort in the right direction.
Use Cases of AI in Digital Marketing
AI for Automated Processes
Human insight is still crucial despite the skyrocketing trend of adopting AI technology. AI is becoming a primary aspect of a modern paid media strategy. By automating the iterative tasks, you can spare a lot of time and money that can be utilized elsewhere in the core business operations. Investing less time on iterative tasks can also boost productivity and performance.
AI for Content Creation and Curation
Producing quality content is a primary aspect of establishing authority within your industry or niche. Ensuring authority improves organic rankings and transparency in the Search Engine Results Pages - SERPs. Conventional Marketers may be reluctant to let AI take the controls and generate content autonomously, but this technology is closer than one may think. The New York Times, Reuters, and Washington Post already use AI to generate content. The Press Association, for instance, can now produce 30,000 local news articles a month using AI. You might think that these are formulaic like who, what, where and when stories, and you are right; some of them certainly are. But now, AI-produced content has expanded beyond formulaic production to more creative writing domains such as poetry and novels.
The methodology that automatically produces a written narrative from data is called natural language generation (NLG), which is already used for different content generation needs from business intelligence dashboards, business data reports, personalized email, and in-app messaging communication to client financial portfolio updates, etc. Now digital marketers will have more time to work on strategic growth plans, face-to-face meetings, and other core business areas where human assistance is more valuable than AI.
AI to Predict Customer Behavior
Predictive analytics is another of the most exciting use cases of AI for digital marketers. Many predictive analytics companies encourage users to visualize and analyze data in a secure environment, such as Microsoft Power BI, a self-service platform introduced for non-technical business users. Now you don't need to be an expert in big data to adapt to the new technology.
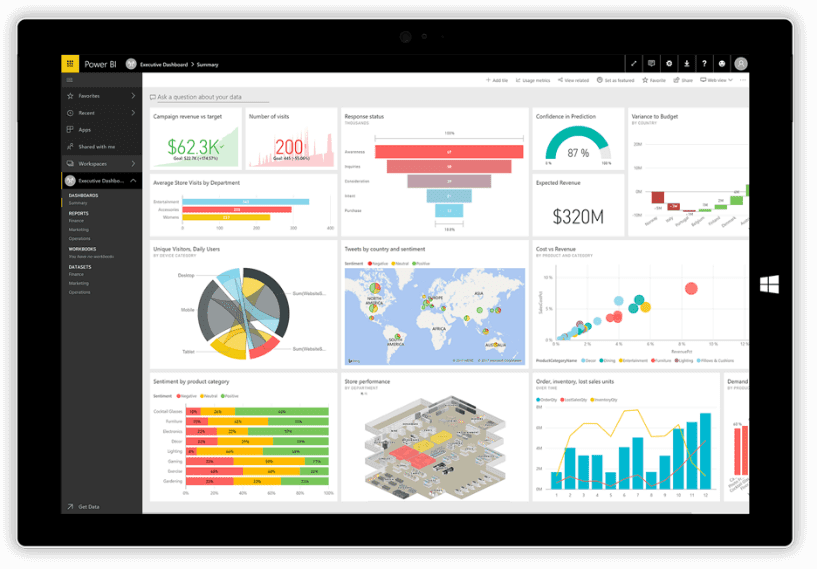
Microsoft Power BI
The accuracy promised by the AI tool is so overwhelming that you can predict customers' future behaviour based on past behaviour, data, and statistical models. In this aspect, AI is not only saving time but ensuring precision. When customers' actions can be predicted accurately, you can showcase them with highly personalized ads and notifications that will lead them through a buying funnel that improves engagement and boosts sales. In the same way, AI-based digital marketing can let you acknowledge your target market segment. Outlining your Ideal Customer Profile - ICP is crucial to extract the most out of your marketing budget because spending time on leads that aren't going to buy is a waste of time and money. As far as self-evaluation is concerned, Modern AI tools can analyze your sales history and quickly determine the firmographics and demographics to let you know where you can focus your efforts.
AI for Better Personalization
Personalization is no longer just a trendy buzzword as personalized messaging, as it is quickly becoming a norm. Most online consumers expect brands to personalize their messages, whether personalized based on matching their location, demographics, or certain interest. Many consumers won't even give attention to messages that are not personalized. By analyzing consumer data and behaviour, AI algorithms can actively personalize CX's customer experience to ensure consistent engagement and interest. Recommendation engines are one of the most prominent examples of AI technology by virtue of which you can easily adapt a more fruitful marketing strategy by knowing your customers' buying habits and interests. You can most commonly witness this technology used on eCommerce platforms and OTPs. Consider an example of Netflix. Their goal is to keep you interested in their subscription service. Before and after you finish your favourite season or movie, Netflix pushes recommendations and Top Picks for you to keep you engaged and refrain from cancelling.
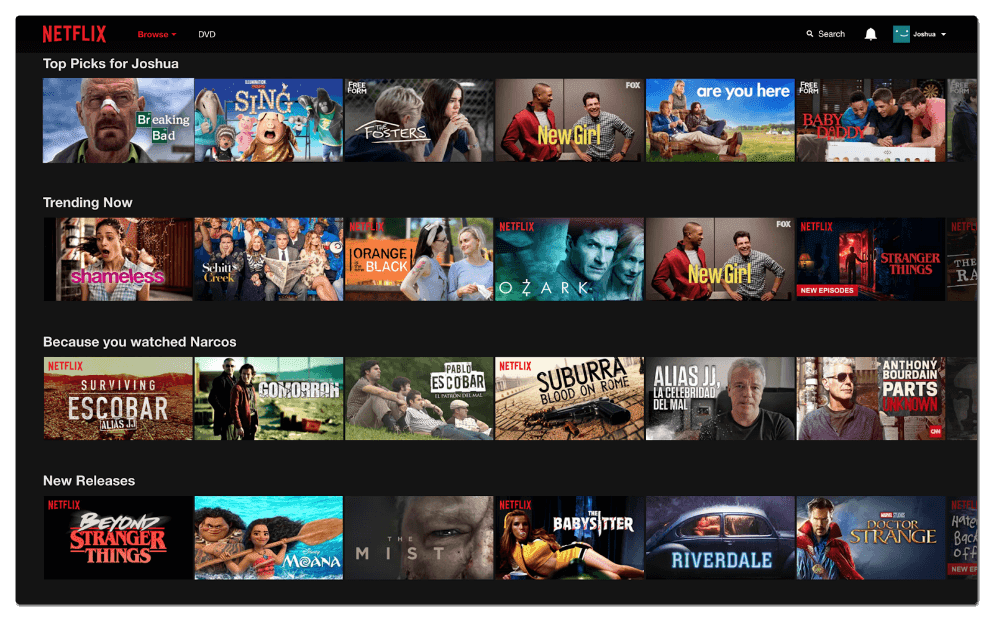
The average user doesn't know about Netflix using AI to learn their watch history – they'll just be excited to start watching Dark as soon as they finish Stranger Things.
Amazon uses AI greatly by providing personalized recommendations based on buying history, items you have viewed or liked and items in your cart, etc. These recommendations are more useful once a user is already in the final stage of the purchasing funnel.
AI chatbots and Digital Marketing
Different AI methodologies such as semantic recognition and natural language processing are intended to improve customer experience and deliver a better response. Compared with manual customer service, AI chatbots have many perks. First of all, AI chatbots allow businesses to go one step further than the conventional "one-to-one" form of customer service. Instead, chatbots can promise "one-to-many" support by interacting with several customers simultaneously and in different time zones. Secondly, AI chatbots don't need to rest as humans do to answer customer queries 24/7 and in real-time with the same accuracy and speed. Not only does this mean they are highly efficient, but they can also deliver services outside of office hours. Last but not the least, customers can opt for the language used by AI customer service as per their needs and feasibility, allowing a brand to deliver a personalized customer experience. Many brands have begun to interact with their customers using messenger applications like WhatsApp, Facebook Messenger, and Slack, all of which can benefit from AI chatbots in order to automate the process and improve customer experience without involving human effort.
Chatbots for Customer Service
Chatbots, powered by AI, can communicate with customers in real-time, providing instant responses to inquiries and support requests. These virtual assistants are capable of handling a wide range of tasks, from answering FAQs to guiding users through a purchase process, enhancing the customer experience while reducing the workload on human customer service representatives.
Predictive Analytics
Predictive analytics leverages AI to forecast future customer behaviors based on their past actions. By analyzing data patterns, AI can identify potential future purchases, allowing marketers to tailor their strategies to nudge the customer towards a sale. This proactive approach in marketing ensures that customers are presented with products or services they are likely to need, improving conversion rates.
Content Creation
AI is now able to generate basic content, such as news articles, social media posts, and even some types of image content. While it may not replace human creativity, AI can significantly speed up content production, providing marketers with a tool to create more content in less time. This is particularly useful for maintaining a consistent online presence and engaging with audiences regularly.
SEO and Content Strategy
AI tools can analyze search trends and user queries to inform SEO and content strategies. By understanding what users are searching for, marketers can tailor their content to meet these needs, improving their visibility in search engine results. AI can also suggest topics that are likely to attract attention, helping content creators to stay ahead of trends.
Social Media Insights
AI's ability to process and analyze large datasets extends to social media platforms, where it can monitor mentions, sentiments, and trends related to a brand. This provides marketers with valuable insights into public perception, allowing them to adjust their strategies in real-time to capitalize on positive trends or mitigate negative feedback.
Email Marketing
In email marketing, AI is used to personalize email content for individual recipients, test different subject lines, and determine the optimal send times to increase open and click-through rates. By continuously learning from user interactions, AI can enhance the effectiveness of email campaigns, ensuring that messages are more relevant and engaging to each recipient.
Voice Search Optimization
As voice search becomes more popular, AI is helping marketers optimize their content for voice queries. This involves understanding the natural language patterns used in voice searches and ensuring that content provides clear, concise answers to these queries. Optimizing for voice search can improve a brand's visibility in voice search results, a growing segment of online searches.
FAQs on AI in Digital Marketing
Q1: What is AI in digital marketing?
AI in digital marketing refers to the use of artificial intelligence technologies to improve marketing strategies and efforts. This includes personalizing content, optimizing ad campaigns, automating customer service with chatbots, and much more, all aimed at enhancing efficiency and engagement.
Q2: How does AI personalize marketing content?
AI personalizes marketing content by analyzing user data like browsing history, purchase behavior, and preferences. It uses this information to predict what content or products a user might find interesting, creating personalized experiences for each individual.
Q3: Can AI write blog posts or create content on its own?
Yes, AI can generate basic content like blog posts, social media updates, and simple images. However, it's best used as a tool to assist content creators by providing suggestions or drafting content, rather than replacing human creativity entirely.
Q4: How do chatbots improve customer service?
Chatbots handle inquiries and support tasks 24/7 without human intervention, providing instant responses to customers. They can answer FAQs, guide users through processes, and even handle basic transactions, improving the overall customer experience.
Q5: Is AI in digital marketing expensive to implement?
The cost varies depending on the scale and tools used. Some AI tools and platforms offer cost-effective solutions for small to medium-sized businesses, while more advanced implementations might require a significant investment. However, the return on investment can be substantial due to increased efficiency and engagement.
Q6: How does AI help with SEO and content strategy?
AI tools analyze search trends and user behavior to suggest content topics and optimize for search engines. They can identify what users are searching for and help create content that matches these queries, improving visibility and engagement.
Q7: What is predictive analytics in marketing?
Predictive analytics uses AI to analyze data and predict future customer behaviors, such as potential purchases. Marketers use these insights to tailor their strategies, targeting users with content and offers that match their predicted needs.
Q8: Can AI manage social media accounts?
While AI can't fully manage social media accounts, it can help by scheduling posts, analyzing engagement data, and providing insights into trends and sentiment. This aids in creating more effective social media strategies.
Q9: How does AI optimize email marketing campaigns?
AI optimizes email marketing by personalizing emails for each recipient, testing different subject lines, and determining the best times to send emails. This increases the chances of emails being opened and acted upon.
Conclusion
AI is revolutionizing digital marketing by providing tools and technologies that enhance the efficiency and effectiveness of marketing strategies. From personalization to predictive analytics, AI is enabling marketers to engage with their audiences in more meaningful ways. As AI technology continues to evolve, its impact on digital marketing is expected to grow, offering even more opportunities for innovation and engagement. Marketers should stay informed about AI developments to leverage these technologies fully, ensuring they remain competitive in the ever-changing digital landscape.
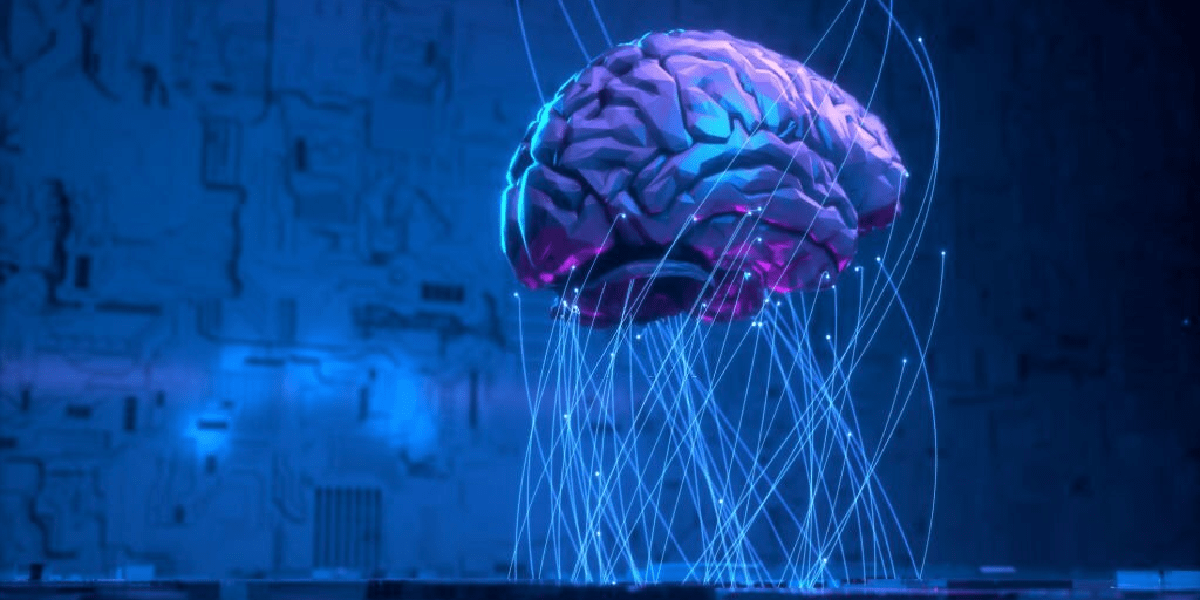
How Generative AI is Redefining Product Development Strategies
Are you a product owner or a business analyst?
Then you know that keeping up with the time requires a combination of modern strategies and ground-breaking technology.
It’s no surprise that 60% of businesses are already implementing generative AI to accelerate and enhance every step of their product engineering process. Overnight, transformative AI technology has broadened the scope of opportunities and opened up new avenues for product development.
This post explores how businesses are using generative AI for product development and how it helps them create lasting value.
How Generative AI is Fueling Product Development
Whether you consider improving your time-to-market, making data-driven decisions, or improving overall efficiency, generative AI has got it all covered. Traditional methods of product development were time-consuming and resource-intensive, which meant that businesses had to deal with a higher upfront investment.
Assisting the Development of Market-fit Products
Since the traditional product development approach does not involve a deeper analysis of user feedback, you are unable to develop products according to their needs. As a result, there is a higher risk of developing a product that is not a market fit.
On the other hand, training generative AI for product development allows you to not only accelerate but also improve the accuracy of complex tasks. Instead of relying on guesswork, design teams can leverage user feedback and approach their projects with data-driven insights. Generative AI replaces assumptions with real information, which is why 92% of product managers see a lasting impact of AI on product management.
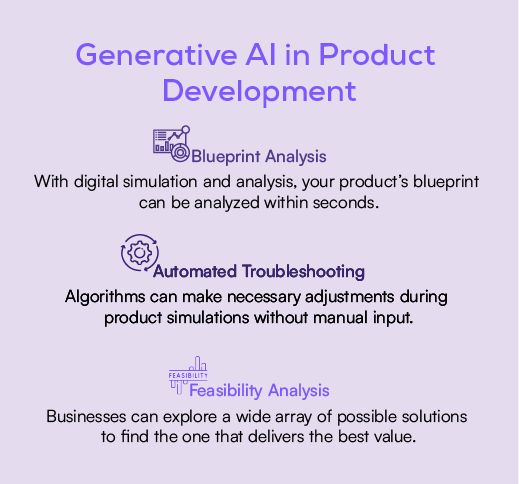
Broadening the Scope of Product Design
When it comes to product development, time-to-market is key to success. This is where the capabilities of generative AI really shine through. Businesses are using it to develop product ideas by analyzing what competitors are doing and what customers are expecting. Compared to manual brainstorming, the ideation process involving generative AI is much more comprehensive and creative.
For instance, you can create more design variations while staying true to a given criteria. When you feed AI with your design preferences, target audience, and product specifications, it can help you validate your ideas and refine your product. That’s not all - generative AI also helps you achieve digital shelf success by helping you understand the shifting demands of customers.
Finesse - An AI-driven Fashion Retailer
Finesse takes the on-demand fashion trend to the next level with the help of AI. Also known as the first “AI-driven fashion house”, it uses a combination of an AI-based voting system and its proprietary AI algorithms to determine the quantity of each item to be manufactured.
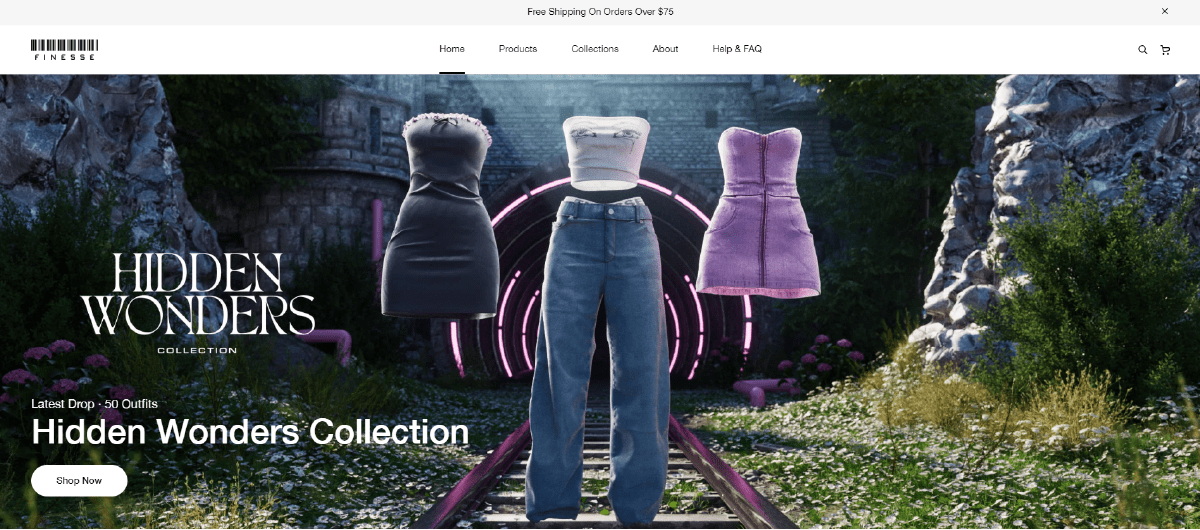
Since the whole process is based on customer demand, it helps the brand in preventing overproduction to optimize costs. In the meantime, the customers can get their hands on the products that they love, and the brand does not have to go through the traditional post-launch user acceptance testing process.
Benefits of Incorporating Generative AI in Product Development
The power of generative AI is not limited to gathering customer feedback and utilizing it for product ideation. It also allows businesses to get the latest insights and base their decisions on market research and trend analysis. Here are some of the benefits of using generative AI for product development:
Enhanced Creativity and Innovation
Normally, product development involves bringing together a group of individuals for brainstorming and ideation. The goal here is to get different perspectives and make room for innovation. But what if we replace the individuals with AI?
With generative AI, you get a significant boost in data processing power, which means you can analyze a wide range of data points related to emerging trends and new product ideas.
AI enhances your team’s creativity by shedding light on areas that you may be ignoring. It factors in data points from different channels, product ratings, focus groups, and social platforms to put you on the right track.
Speeding up Iterations and Prototyping
In the early days, this kind of processing power wasn’t associated with quick results. However, that is not the case anymore. Generative AI analyzes vast amounts of data to identify patterns and delivers the output within seconds. Unlike traditional product prototyping where you need a lot of time and resources, AI-based prototyping essentially automates the whole process.
It speeds up your prototyping process by leveraging machine learning algorithms to create new features and designs. Basically what you are getting is better quality and diversity of ideas in less time than it would take a team of individuals. You can innovate and create differentiated products while reducing operational costs.
Product Personalization
Since every other brand is using personalization to engage customers, you can’t skip this part either. According to a 2022 Salsify research, 70% of U.S. shoppers are more likely to buy an item if there are “personally relevant” images, text, and reviews for it.
Thankfully, you can leverage generative AI to tailor your products to the needs of your customers. As seen in the Finesse example earlier on, you can allow your customers to choose how the product should look like. This agile approach to product design allows your team to try out multiple concepts, curate their ideas, and have better chances of engaging the right audience. Numerous eCommerce businesses are now using the customer-first approach as a way to get positive reviews and make their way to the top.
Cost-efficiency and Resource Optimization
While AI is doing its magic and accelerating your operations, it also helps diagnose problems and eliminate risks. No matter how well you plan your product development process, there are always going to be some hurdles. Generative AI automates repetitive, time-consuming, and resource-intensive tasks to help you save time and manage costs efficiently.
Generative AI can enhance your designs and provide the most suitable options based on manufacturing costs. This involves analysis of the costs associated with materials, assembly, and manufacture. Then, it helps you minimize material waste, optimize the supply chain, and opt for cost-effective options. Generative AI is also capable of suggesting efficient assembly flows and procurement timelines.
Challenges and Considerations
With so many benefits, you might think that generative AI also has some drawbacks. And you’re right. Here are some of the challenges involved in implementing generative AI for product development:
- Complexity of Training Generative AI: In order to implement AI for product engineering, you need specialized knowledge and expertise. Apart from ensuring data security, you need to deal with the complexity of training and maintaining the AI models.
- Ethical Concerns: This is where the difference between humans and machines shines through. Generative AI can raise ethical concerns when it is used to collect and process personal information or intellectual property. The outputs can also be subject to copyright issues and malicious uses.
- Data Quality and Bias: Based on the quality and diversity of the data they are trained with, generative AI models can sometimes deliver biased outputs. As this mainly depends on the datasets used to train the AI, businesses need to be careful about data quality and bias.
- Lack of Control: While the scope of data processing is to be appreciated, you cannot always maintain control of content generated using generative AI. These AI models can even produce undesirable and unexpected outputs that need to be verified and amended manually.
How to Overcome the Challenges of Generative AI
Accuracy
First and foremost, businesses need to ensure that they can train AI models effectively using their own data. This not only allows them to get verifiable outputs but also achieve maximum accuracy. You need to be sure that the generative AI model can identify and process the unique datasets provided by your business and produce the right outputs.
The best way to ensure that your AI models are properly trained is to have a check and balance. Team members should communicate errors and uncertainties in the outputs they get from generative AI. This may also require team members to cite the sources where the generative AI has picked the data, and then explain how it is justified.
Security
While you have assigned tasks to AI experts for training a particular AI model, you need to conduct regular tests to mitigate potential risks. For instance, you need to ensure that there is no bias in the data being used to train the models and whether the outputs being generated contain any harmful content. These security checks also give your business the opportunity to identify vulnerabilities or the involvement of bad actors in the form of potential cyber-attacks.
Transparency
So how do we deal with the issue of bad data quality? By ensuring transparency.
You need to have measures in place to ensure that the data collected to train AI models is evaluated. Your business must respect provenance and have the consent to use the data.
To ensure transparency, your business can use open-source or user-provided data to train AI models. When you are using AI outputs to carry out a task, you need to be transparent and clearly state that the content is generated using AI. Watermarks on the content or in-app messages can be used to differentiate between human-written and AI-generated content.
Future Trends and Implications
AI will Determine Whether or Not a Product is Market-fit
According to statistics, a shocking 35% of SMBs and startups fail because their products are simply not in demand. Soon, AI will help solve this problem. Generative AI-based data analysis will allow businesses to gather accurate data and establish a better understanding of what is required in the market. This includes targeting the right audience and developing the right products within a given category.
AI is already helping businesses understand their customers better with the help of extensive data analysis. It digs deeper into their preferences and searches to find hidden problems in existing products. Up until yesterday, we didn’t have any tools to analyze such information and provide suggestions for product designs and features.
AI will Enhance Human Capabilities for Product Development
Although machine learning has been around for quite some time, the capabilities of AI are now increasing at a drastic rate. While the previously used machine learning algorithm learned patterns and produced outputs using the data provided, the future will see the generation of unseen data. AI will soon be able to apply the ‘learned’ patterns to new and unique data and generate new outputs.
In fact, generative AI models are already advanced enough to take things further. They can not only use unseen data but also develop an understanding of the thought process and ideation behind the datasets provided.
Key Takeaways
From what we have seen in recent times, it is safe to say that generative AI is transforming product development and giving a new angle to innovation. Apart from streamlining processes, improving customer experience, and empowering startups, generative AI is assisting product owners with new design ideas and development processes. As we see the technology evolve, the use cases of generative AI in product engineering and management are set to expand further.
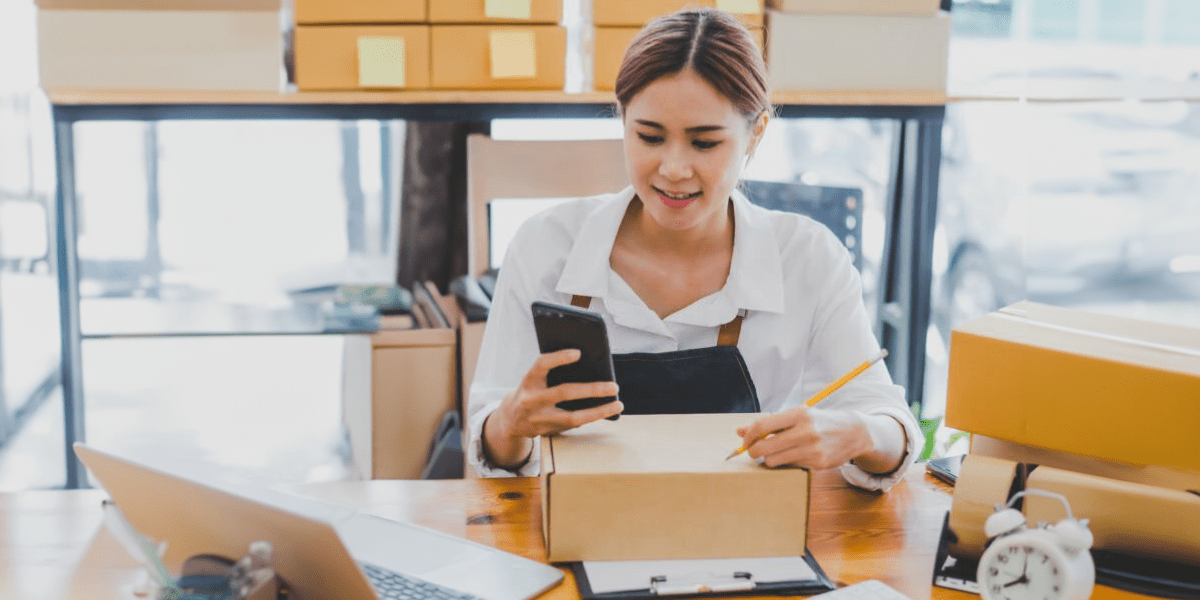
How Generative AI Enhances Inventory Management in Retail
The retail sector is accustomed to razor-thin margins and serious competition, but there is no bigger concern than inventory management. Overstocking, stockouts, and inventory shrinkage cost businesses around the world approximately $1.8 trillion. The impact of manual inventory tracking can be seen in the form of delayed orders and inconvenience for customers, which comes as a big blow to retailers.
In order to meet customer demands, you always need to have enough stock in your inventory, and this is only possible with a comprehensive inventory management system.
By providing additional capabilities to monitor and control stock levels, the buzzworthy generative AI technology is now allowing retailers to offer enhanced customer experiences.
This post covers everything you need to know about the application of generative AI to manage stock levels in retail.
Why Retailers Should Embrace Generative AI
Even though poor inventory management directly affects profitability, a shocking 43% of small businesses still do not manage their inventory even today. This means that when an order comes in, 34% of businesses end up selling products that aren’t available in their inventory. As a result, customers face significant delays in shipment, and the business loses its chances of getting any positive reviews.
Utilizing generative AI for supply chain optimization means you can solve longstanding inventory problems like stockouts, overstocking, and shrinkage. A typical retail supply chain comprises the following processes:
- Procurement
- Production
- Distribution
- Customer Service
The capabilities of AI, which extend beyond data processing, become particularly useful in retail as it can predict future trends and analyze large amounts of data in real-time. By incorporating generative AI in retail, you can track the quantity, location, and status of raw materials, products being manufactured, and finished goods.
Sharper Decision-Making
Utilizing generative AI for inventory management, you can get real-time insights into your stock levels in different locations and manage them effectively. Unlike manual inventory management, AI can factor in numerous variables like lead times, demand patterns, seasonality, carrying costs, and the risk of obsolete inventory. It also allows you to optimize your inventory to make products available when they are needed, and avoid overstocking at the same time.
Boost Customer Satisfaction
When your customers are able to find the right product in your stock, you get a hundred percent satisfaction rate. But when they don’t, it means you have simply lost a valuable lead. With the help of generative AI, you can create exceptional shopping experiences for your customers and provide them with more than just what they are looking for.
By analyzing their searches and preferences along with supply and demand metrics, generative AI enables your business to exceed customer expectations. Not only that, but the availability of hot-selling products in your stock means you have a higher chance of gaining customer loyalty.
Cost Savings and Efficiency Gains
Before AI, inventory management was based on hit and trial. You had to rely on guesswork for ordering inventory items. Sometimes it worked, and sometimes you ended up having extra items on your shelves. However, generative AI has now enhanced the accuracy of inventory management by a significant margin. You can order just the right quantity of items at the right time to save both time and money.
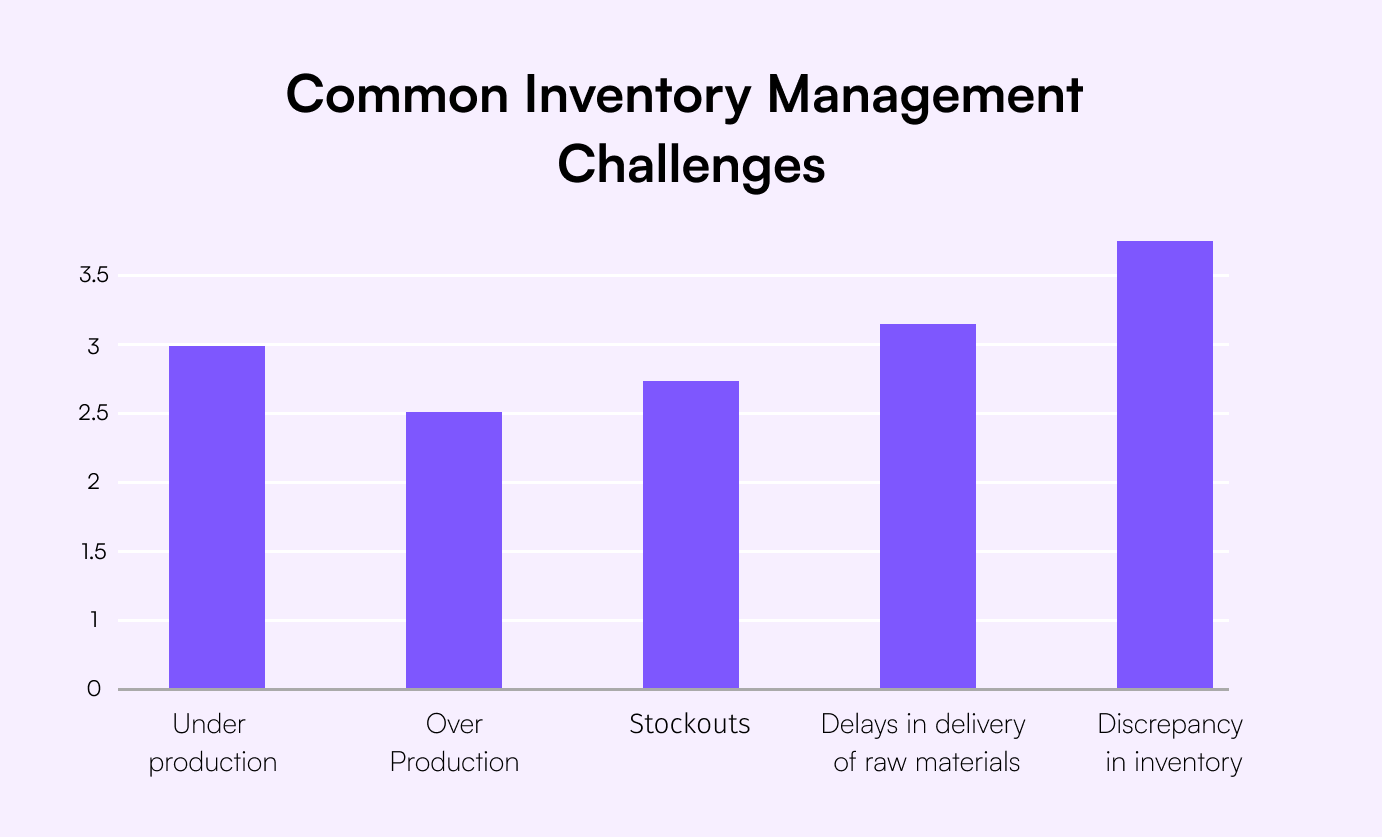
Considering the high efficiency of AI, you can cut down on costs in terms of storage, wastage, and higher sales. All this is possible because generative AI allows you to forecast how much is needed, and sell faster so you do not have to store items in your warehouses for longer periods of time. Looking at the small margins retailers have to play with, this kind of cost-effectiveness is what everyone needs. Instead of wasting resources on overstocking, your business can spend more on innovation and growth.
Real-world Applications of Generative AI in Supply Chain Management
While AI offers so many benefits, it’s no surprise that brands around the world have incorporated generative AI into their supply chain. The following are some notable examples of real-world applications of generative AI:
Walmart
The secret behind the popularity of the retail giant Walmart is its use of generative AI for selecting suppliers and managing risks. By employing generative AI, Walmart is able to analyze different factors like geographical locations, financial standing, and supplier performance to make the best business decisions. The aim is to partner with an enhanced supplier base and eliminate loopholes in the supply chain.
Procter & Gamble (P&G)
The case is quite similar at Procter & Gamble. The renowned brand utilized generative AI for demand forecasting, whereby historical data and variables such as market trends, weather, and more are considered to predict the demand for each product. With the help of AI, P&G is able to enhance demand forecasting, reduce costs, and improve its overall inventory performance.
DHL
When it comes to logistics, you need to have your delivery routes planned out to extract maximum business value. The global logistics service provider, DHL, understands this and utilizes generative AI for resource allocation and route optimization. With AI working its magic, DHL can adjust its delivery routes dynamically. In this case, generative AI factors in real-time data such as weather, traffic conditions, transit times, and fuel requirements.
Flexport
Similarly, Flexport has also integrated generative AI to benefit from real-time supply chain visibility and optimization. Techniques like predictive analytics and demand forecasting allow the logistics platform to identify potential disruptions in freight routes and find the best possible solutions well before time.
Llamasoft
Apart from the key players that have an active role in supply chains, there are also analytical entities that benefit from generative AI. One such example is Llamasoft, which has incorporated generative AI to dry-run different supply chain scenarios and allow companies to make better decisions. Using generative AI analytics, businesses are able to identify loopholes and optimize their supply chain effectively.
How Generative AI Transforms Planning in Supply Chains
With all its analytical power, it’s not hard to guess that AI helps improve the planning and scheduling processes of supply chains. This is made possible by considering variables like production capacity, resource availability, order priority, and the customers themselves.
Considering these factors, generative AI can lay out complete production plans that include schedules, sequences, and resources. The ability of AI to analyze multiple variables simultaneously means that production plans are free of loopholes and ensure maximum production efficiency.
Example
One example of applying generative AI to improve demand forecasting can be seen in biotech companies, which use generative AI tools to get useful insights for production planning. For instance, the company can simulate scenarios where they use specific chemicals to develop their products and assess the impact it has on the global market. In fact, generative AI is now advanced enough to recommend courses of action in case the company’s own ideas go wrong. When it comes to risk management, AI is second to none.
Empower Sourcing Strategies with Generative AI
Once you have your supply chain planned out, the next step is to select the right suppliers. Fortunately for modern retailers, generative AI can assist and improve the supplier selection process with its analytical power. It can analyze supplier performance, pricing, associated risks, capabilities, and rankings to help you make informed decisions.
That’s not all, a leading US retailer even developed their own generative AI-based bots to negotiate purchasing terms and costs with vendors. Not only does it allow them to get the job done in a shorter amount of time, but it also helps reduce costs. If you think about it, generative AI has taken all the complexity out of the supplier selection process. It’s no surprise that 75% of the vendors prefer to negotiate with the bots instead of humans at the retail company.
Accelerate Production with Generative AI
The good news does not stop there. Generative AI can also assist retailers in speeding up their production process. Whether you are considering designing new parts for your production line or making your products more durable, generative AI can analyze numerous designs to suggest the ideal way forward.
Accelerating and improving production has obvious advantages in the retail environment. For this reason, businesses are training generative AI models on their datasets to achieve higher levels of productivity and efficiency. Preventive maintenance, another technique of generative AI, helps identify areas that are likely to fail or malfunction in your production line, hence allowing you to improve overall equipment effectiveness (OEE).
A leading manufacturer in Europe has incorporated generative AI to automate its production line. With the help of AI-based factory automation and product lifecycle management, the company is able to achieve more in less time. It has even improved its production cycle by employing automated inspection with AI.
Logistics Revolution: How Generative AI Optimizes Movement
After manufacturing your products, the next question is how to move the stocks to different warehouses and outlets. But you can rest assured that generative AI can help you in this step as well. Generative AI can optimize your logistics network by processing data points like transport links, demand patterns, and warehouse locations. This allows AI to create the most efficient logistics configuration with lower delivery times and costs.
Speaking of logistics and route selection, it's worth mentioning that one of the biggest logistics service providers in the US utilizes a proprietary AI platform for optimizing its supply chain. This includes optimizing delivery routes, warehouse selection, and improving productivity by 30%. Moreover, generative AI also allows you to cut down on operational costs while allowing you to customize your logistics process like never before.
Strategies for Implementing Generative AI in Supply Chains
With all its powerful applications and benefits, generative AI is still not tailor-made for your specific business needs. As the implementation varies from one use case to another, you need to ensure that your AI solution integrates seamlessly with your existing applications. In some cases, companies have to re-engineer some of their existing processes to enable the AI solution to fit in.
Here’s how you can implement generative AI in your supply chain:
- Define what you want to achieve by implementing generative AI in your supply chain. This will allow you to select the right AI models to work with.
- Ensure that your data collection process is automated so that the AI algorithms can access the latest information
- Process the data to be fed to the AI and machine learning algorithms
- Identify high-impact use cases to create a synchronized ecosystem of traditional and modern business processes
- Implement generative AI to improve internal collaboration and coordination in your organization
- Identify the functionality and skill required for specific tasks and improve productivity
- Incorporate pilot initiatives to reduce risks and develop scalable processes
Overcome Challenges and Maximize Generative AI’s Potential
Now that we have discussed the benefits of generative AI for optimizing supply chains, let’s address some of the implementation challenges.
- Generative AI models work best with high-quality data inputs. So the most obvious challenge is data availability and quality.
- Training generative AI models is a time-consuming and costly process as it requires expertise and computational power.
- Supply chain decisions require transparency. It’s often difficult to interpret and explain to stakeholders how generative AI has reached the said results.
- Generating synthetic data based on assumptions to simulate supply chain scenarios can lead to legal and ethical challenges.
With that being said, the retail industry continuously faces challenges in one form or another. While the implementation of generative AI inventory management may cost you more than manual inventory management, the results are also that much better. At the end of the day, your best bet is to have AI and human intelligence working together in perfect harmony.
Key Takeaways
With technological advancements transforming inventory management, the competitive retail industry is looking for the best AI solutions. Leveraging generative AI for supply chain optimization allows retailers to achieve higher efficiency and also makes room for innovation. With accelerated operations and enhanced decision-making, business owners are able to identify and work on hidden weaknesses that have a significant impact on their customer base.
Book your free 40-minute
consultation with us.
Let's have a call and discuss your product.