Table of content
In the dynamic realm of data science, having the right tools at your disposal can make all the difference. Python, with its simplicity, versatility, and rich library ecosystem, has emerged as the go-to language for data scientists worldwide. In this blog post, we'll delve into the 21 must-know Python libraries for data science in 2024, each of which plays a crucial role in different facets of data analysis, visualization, and machine learning.
Benefits of Using Python For Data Science
Python's ascent to prominence in the field of data science is no coincidence. Its intuitive syntax, extensive community support, and powerful libraries have made it the preferred choice for data professionals. Let's explore some key advantages of using Python for your data science endeavors:
1. Simplicity and Readability
Python's clean and readable syntax allows data scientists to focus on solving problems rather than wrestling with code complexity. This simplicity not only accelerates development but also promotes collaboration within teams.
2. Vast Ecosystem of Libraries
One of Python's greatest strengths lies in its extensive library ecosystem. With specialized libraries for tasks ranging from numerical computing to natural language processing, Python provides a comprehensive toolkit for data scientists.
3. Large and Active Community
The Python community is a thriving hub of knowledge and expertise. Whether you're seeking advice on a specific library or encountering a coding challenge, chances are someone in the community has faced a similar situation and can offer guidance.
How To Choose The Right Python Libraries For Your Needs
Selecting the right Python libraries is a pivotal decision for any data science project. Each library brings its own set of capabilities and specialties to the table. To ensure you're making informed choices, consider the following factors:
1. Functionality and Use Case
Determine the specific tasks and analyses you need to perform. Some libraries excel in numerical computing, while others are tailored for natural language processing or machine learning.
2. Ease of Use and Documentation
Evaluate the user-friendliness of a library. Clear documentation and well-maintained resources can significantly reduce the learning curve.
3. Compatibility with Existing Tools
Ensure that the chosen libraries integrate smoothly with your existing tech stack. Compatibility with other tools and frameworks can streamline your workflow.
4. Community and Support
Consider the size and activity level of the library's community. A vibrant community can provide valuable insights, troubleshooting help, and contribute to the library's continued development.
5. Performance and Scalability
Depending on your project's requirements, assess the performance benchmarks of the libraries. Some libraries may be optimized for speed, while others focus on scalability.
6. License and Usage Policies
Verify that the library's license aligns with your project's requirements. Some libraries may have specific usage restrictions or licensing terms to be aware of.
By carefully weighing these factors, you can make informed decisions when selecting the right Python libraries for your specific data science needs.
Detailed Overview of Essential Python Libraries
1. NumPy

Role in Numerical Computing and Handling Arrays and Matrices
NumPy, short for Numerical Python, is a fundamental library for numerical computations in Python. It provides support for handling large, multi-dimensional arrays and matrices, along with a collection of high-level mathematical functions to operate on these arrays.
NumPy's primary contribution lies in its ability to perform array operations with a speed and efficiency that exceeds native Python lists. This makes it an essential tool for numerical tasks in data science, machine learning, and scientific computing.
Examples of Scenarios
Matrix Operations: NumPy simplifies complex matrix computations. For example, in linear algebra, you can use NumPy to perform operations like matrix multiplication, inverse calculations, and eigenvalue computations.
Statistical Calculations: NumPy is used extensively for statistical analysis. It allows for efficient computation of various statistical measures such as mean, median, standard deviation, variance, and more.
Signal Processing: In fields like digital signal processing, NumPy is crucial for tasks like filtering, Fourier transforms, and other frequency-domain operations.
Random Number Generation: NumPy includes functions for generating random numbers, which is essential in simulations and various statistical applications.
Data Manipulation and Cleaning: It's used for reshaping and cleaning datasets, especially when dealing with missing or incorrect data points.
Machine Learning: NumPy is the backbone of many machine learning libraries. It's used for implementing algorithms like support vector machines, principal component analysis, and more.
NumPy's efficiency in handling numerical operations and its wide array of mathematical functions make it an indispensable tool for any data scientist.
2. pandas
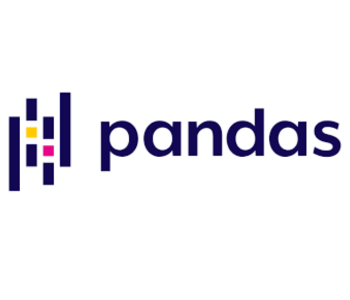
Facilitating Data Manipulation and Analysis
Pandas is a powerful library built on top of NumPy, designed specifically for data manipulation and analysis. It introduces two fundamental data structures: Series (1-dimensional) and DataFrame (2-dimensional), which provide a flexible and intuitive way to handle structured data.
Examples of Use Cases
Data Cleaning and Preparation: Pandas excels at handling missing data, data alignment, and data transformation. It allows for tasks like filling in missing values, dropping unnecessary columns, and transforming data into a format suitable for analysis.
Exploratory Data Analysis (EDA): With pandas, you can perform essential EDA tasks like summarizing data, calculating descriptive statistics, and visualizing distributions. This is crucial for understanding the underlying patterns and characteristics of a dataset.
Data Aggregation and Grouping: Pandas facilitates the process of grouping data based on specific criteria and performing aggregate operations. For instance, you can easily calculate sums, means, counts, etc., based on different groups within the dataset.
Merging and Joining Datasets: It provides powerful tools for combining datasets based on a shared key. This is essential for tasks like merging data from multiple sources or performing database-like operations.
Time Series Analysis: Pandas offers specialized functionalities for handling time series data, making it an ideal choice for financial and economic analysis, as well as other time-dependent datasets.
Handling Categorical Data: It provides robust support for categorical data, including the ability to perform operations like encoding and decoding categorical variables.
Data Input and Output: Pandas can read data from various file formats (CSV, Excel, SQL databases, etc.) and write data back to these formats after manipulation and analysis.
Integration with Visualization Libraries: It integrates seamlessly with visualization libraries like Matplotlib and Seaborn, enabling easy generation of informative plots and visualizations.
Overall, pandas' ease of use, extensive functionality, and compatibility with other libraries make it an indispensable tool for data wrangling and analysis in Python.
3. Matplotlib
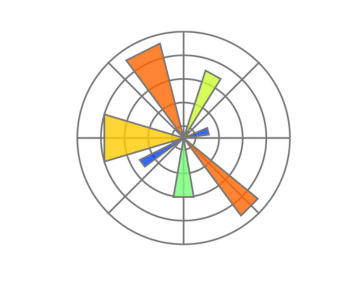
Role in Basic Data Visualization
Matplotlib is a comprehensive library for creating static, animated, and interactive visualizations in Python. It is particularly powerful for producing 2D and limited 3D plots, making it a cornerstone for data visualization in data science.
Capabilities and Use Cases
Line Plots: Matplotlib is adept at creating line plots, making it suitable for visualizing trends and time series data. This is crucial for tasks like tracking stock prices, temperature changes, or any other continuous data.
Scatter Plots: It allows for the creation of scatter plots, which are essential for understanding relationships between two variables. Scatter plots are useful for identifying correlations or clusters within a dataset.
Bar Charts and Histograms: Matplotlib is capable of generating bar charts and histograms, providing tools for visualizing distributions and comparing categorical data.
Pie Charts: It enables the creation of pie charts for displaying proportions or percentages within a dataset.
Error Bars and Confidence Intervals: Matplotlib supports the inclusion of error bars and confidence intervals in plots, aiding in the interpretation of uncertainty in data.
Subplots and Grids: It allows for the creation of multiple plots within a single figure, facilitating the comparison of different aspects of the data.
Annotations and Text: Matplotlib provides options for adding annotations, labels, and text to plots, enhancing their interpretability.
Customization and Styling: It offers a wide range of customization options, allowing users to modify colors, styles, and other visual aspects of plots to match specific preferences or requirements.
Exporting and Saving Plots: Matplotlib enables the export of plots in various formats such as PNG, PDF, SVG, etc., making it easy to incorporate visualizations into reports or presentations.
Matplotlib's versatility and extensive documentation make it a powerful tool for creating a wide variety of static visualizations, from simple line plots to complex, multi-panel figures.
4. Seaborn

Enhancing Statistical Data Visualization
Seaborn is a high-level data visualization library that builds on top of Matplotlib. It specializes in creating aesthetically pleasing and informative statistical graphics. Seaborn provides a high-level interface for producing visually appealing visualizations with minimal code.
Key Features and Applications
Statistical Plots: Seaborn offers a wide range of statistical plots such as scatter plots, bar plots, violin plots, and box plots. These plots incorporate statistical summaries directly into the visualization, providing insights into the underlying data distribution.
Categorical Data Visualization: Seaborn excels at visualizing categorical data through plots like categorical scatter plots, bar plots, and count plots. It's particularly useful for understanding the distribution of categorical variables and their relationships.
Multi-plot Grids: It provides the ability to create multi-plot grids, allowing for the simultaneous visualization of multiple aspects of the data. This is valuable for exploring complex relationships within a dataset.
Color Palettes and Themes: Seaborn includes a range of aesthetically pleasing color palettes and themes, making it easy to customize the appearance of visualizations.
Time Series Data Visualization: Seaborn can be used effectively for visualizing time series data, enabling data scientists to uncover trends and patterns over time.
Regression Plots: It provides specialized functions for visualizing relationships between variables, including regression plots with confidence intervals, which are valuable for understanding linear relationships.
Matrix Plots: Seaborn offers functions to create visually appealing matrix plots, which are useful for visualizing relationships between multiple variables in a dataset.
Facet Grids: It allows for the creation of multi-plot grids based on categorical variables, enabling a deeper exploration of relationships within subsets of the data.
Pair Plots: Seaborn can generate pair plots for visualizing pairwise relationships in a dataset. This is particularly valuable for understanding correlations and distributions across multiple variables.
Seaborn's focus on statistical visualization, combined with its user-friendly interface, makes it an invaluable tool for data scientists looking to create informative and visually appealing graphics.
5. Scikit-learn
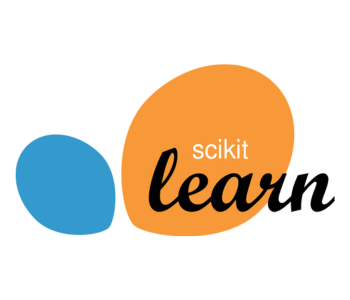
Comprehensive Machine Learning Library
Scikit-learn, often abbreviated as sklearn, is a versatile and comprehensive machine learning library in Python. It provides a wide range of machine learning algorithms, as well as tools for data preprocessing, model evaluation, and model selection.
Key Aspects and Applications
Classification and Regression: Scikit-learn offers a rich collection of algorithms for both classification and regression tasks. This includes popular techniques like Support Vector Machines, Random Forests, and Gradient Boosting.
Clustering: It provides a variety of clustering algorithms for unsupervised learning tasks. These algorithms are essential for tasks like customer segmentation, anomaly detection, and more.
Dimensionality Reduction: Scikit-learn includes methods for reducing the dimensionality of datasets, such as Principal Component Analysis (PCA) and t-distributed Stochastic Neighbor Embedding (t-SNE). This is crucial for visualizing high-dimensional data and speeding up computations.
Model Evaluation and Metrics: The library offers a range of metrics for evaluating model performance, including accuracy, precision, recall, F1-score, and many more. It also provides tools for cross-validation, enabling robust model evaluation.
Hyperparameter Tuning: Scikit-learn facilitates the process of hyperparameter tuning, which involves finding the best set of hyperparameters for a machine learning model. This is crucial for optimizing model performance.
Ensemble Methods: It supports ensemble methods like bagging, boosting, and stacking, allowing for the combination of multiple models to improve predictive performance.
Feature Selection and Engineering: Scikit-learn provides tools for feature selection and engineering, allowing data scientists to identify and use the most relevant features for modeling.
Preprocessing and Pipelines: The library includes various preprocessing techniques such as standardization, normalization, and one-hot encoding. These techniques are crucial for preparing data for modeling.
Outlier Detection: Scikit-learn offers algorithms for detecting outliers in datasets, which is important for ensuring the quality and reliability of the data used for modeling.
Imbalanced Data Handling: It provides techniques for handling imbalanced datasets, which is common in many real-world applications.
Scikit-learn's well-documented API, extensive set of algorithms, and consistent interface make it an indispensable library for both beginners and experienced practitioners in machine learning.
6. SciPy

Role in Advanced Scientific and Technical Computing
SciPy is a library built on top of NumPy, providing additional functionality for scientific and technical computing tasks. It is particularly valuable for tasks that go beyond basic numerical operations and require more specialized functions and algorithms.
Specific Functionalities
Optimization: SciPy offers a wide range of optimization algorithms for tasks like minimizing or maximizing objective functions. This is crucial for tasks like parameter tuning in machine learning models.
Integration: It provides functions for numerical integration, including methods like Simpson's rule and Gaussian quadrature. This is essential for solving problems in calculus and differential equations.
Interpolation: SciPy includes tools for performing data interpolation, allowing for the estimation of intermediate values within a dataset. This is valuable for tasks like curve fitting.
Linear Algebra: While NumPy covers basic linear algebra operations, SciPy extends this with additional functionalities like solving linear systems, computing eigenvalues, and performing sparse matrix operations.
Signal and Image Processing: SciPy includes a variety of functions for tasks like filtering, convolution, and image manipulation. This is crucial for applications in signal processing and computer vision.
Statistics and Probability: It provides a wide range of statistical functions, probability distributions, and hypothesis testing tools. This makes SciPy valuable for statistical analysis and hypothesis testing.
Ordinary Differential Equations (ODEs): SciPy offers solvers for initial value problems in ordinary differential equations. This is essential for simulating dynamic systems.
Sparse Matrices: SciPy provides specialized data structures and algorithms for handling sparse matrices, which are common in scientific and engineering applications.
Numerical Methods for Partial Differential Equations (PDEs): It includes tools for solving PDEs, which are prevalent in fields like physics and engineering.
Statistical Functions: SciPy extends the statistical capabilities of NumPy with additional functions for probability distributions, hypothesis testing, and more.
SciPy's rich collection of functions and algorithms for advanced scientific computing tasks makes it a vital library for researchers, engineers, and data scientists working on complex numerical problems.
7. Statsmodels

Estimating and Interpreting Statistical Models
Statsmodels is a Python library that focuses on estimating and interpreting models for statistical analysis. It provides a wide range of tools for conducting hypothesis tests, exploring relationships in data, and performing various types of statistical modeling.
Key Aspects and Applications
Regression Analysis: Statsmodels excels in performing regression analysis, including linear regression, logistic regression, and more. It provides detailed summaries of regression results, including coefficients, p-values, and confidence intervals.
Time Series Analysis: The library offers a variety of tools for analyzing time series data, including autoregressive integrated moving average (ARIMA) models, seasonal decomposition of time series (STL), and more.
Hypothesis Testing: Statsmodels provides a comprehensive suite of hypothesis tests for different types of statistical comparisons. This is crucial for validating assumptions and drawing meaningful conclusions from data.
Econometric Modeling: It is widely used in economics for estimating and interpreting models related to economic relationships, such as demand and supply, production functions, and more.
Nonparametric Methods: Statsmodels includes methods for nonparametric statistics, which are useful when assumptions about the underlying data distribution cannot be met.
Time Series Forecasting: The library provides tools for building and validating forecasting models, allowing for the prediction of future data points based on historical trends.
Generalized Linear Models (GLM): It supports GLM estimation, which is a flexible framework for modeling various types of relationships in data, including binary outcomes, count data, and more.
ANOVA and Experimental Design: Statsmodels offers tools for conducting analysis of variance (ANOVA) and experimental design, which are crucial for comparing groups and understanding treatment effects.
Multivariate Analysis: It provides capabilities for conducting multivariate analysis, including principal component analysis (PCA), factor analysis, and more.
Statistical Tests for Time Series: Statsmodels includes various tests for diagnosing properties of time series data, such as stationarity tests and tests for autocorrelation.
Statsmodels' emphasis on statistical modeling and hypothesis testing makes it an indispensable tool for researchers and data scientists conducting rigorous statistical analysis.
8. Jupyter Notebooks

Interactive Computing and Document Sharing
Jupyter Notebooks is an interactive computing environment that allows users to create and share documents that combine live code, visualizations, explanatory text, and more. It's a powerful tool for data scientists to perform data analysis, conduct experiments, and document their work in an interactive and reproducible manner.
Key Features and Applications
Live Code Execution: Jupyter Notebooks enable users to write and execute code in individual cells. This promotes an interactive and iterative approach to data analysis, as users can run code segments and immediately see the results.
Rich Output: In addition to code, Jupyter Notebooks support the display of rich outputs including text, images, plots, and even interactive widgets. This allows for comprehensive and informative documentation of the analysis process.
Markdown Support: Users can incorporate Markdown cells for adding formatted text, headings, lists, links, and more. This makes it easy to provide context, explanations, and documentation alongside code.
Data Visualization Integration: Jupyter Notebooks seamlessly integrate with data visualization libraries like Matplotlib, Seaborn, and Plotly, allowing for the creation of dynamic and interactive plots directly within the notebook.
Easy Experimentation: Data scientists can perform experiments and analyses in a controlled environment. They can modify code, rerun cells, and observe the impact on results, making it easy to fine-tune models and algorithms.
Collaborative Work: Jupyter Notebooks can be shared with colleagues or the wider community. This facilitates collaboration, knowledge sharing, and reproducibility of analyses.
Kernel Support: Jupyter supports multiple programming languages through the use of different kernels. While Python is the most commonly used language, kernels are available for languages like R, Julia, and more.
Version Control Integration: Notebooks can be tracked in version control systems like Git, allowing for easy management of changes and collaboration among team members.
Exporting and Converting: Jupyter Notebooks can be saved in various formats including HTML, PDF, and LaTeX. This enables users to share their work in different contexts or publish it as a report.
Interactive Widgets: Jupyter supports the creation of interactive widgets, allowing users to control parameters and visualize results in real time. This is particularly useful for exploring data interactively.
Jupyter Notebooks' combination of code execution, visualizations, and explanatory text makes it an indispensable tool for data scientists seeking an interactive and collaborative environment for their work.
9. TensorFlow or PyTorch
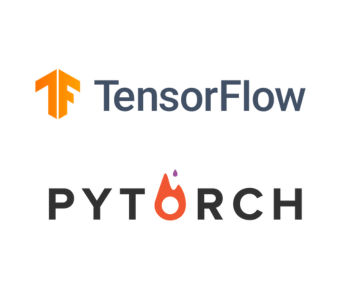
Frameworks for Deep Learning and Neural Networks
Both TensorFlow and PyTorch are powerful open-source libraries for building and training deep learning models. They provide a comprehensive set of tools and resources for constructing and training neural networks, making them essential for tasks like image recognition, natural language processing, and more.
Key Aspects and Applications
TensorFlow:
Graph-Based Computation: TensorFlow follows a computation graph paradigm, where computations are represented as a directed acyclic graph (DAG). This allows for efficient execution on GPUs and TPUs, making it suitable for large-scale deep learning tasks.
High-Level APIs: TensorFlow offers high-level APIs like Keras, which simplifies the process of building and training neural networks. Keras provides a user-friendly interface for designing models without the need to define computational graphs explicitly.
Wide Range of Pretrained Models: TensorFlow includes a vast collection of pre-trained models through the TensorFlow Hub, which allows data scientists to leverage state-of-the-art architectures for various tasks.
TensorBoard for Visualization: It integrates with TensorBoard, a powerful visualization tool, for tracking and visualizing metrics, model graphs, and more. This aids in monitoring and improving model performance.
Production Deployment: TensorFlow provides tools for deploying models in production environments, including TensorFlow Serving for serving models via APIs.
Support for Mobile and Embedded Devices: TensorFlow offers tools like TensorFlow Lite for deploying models on mobile and embedded devices, enabling applications with real-time processing requirements.
PyTorch:
Dynamic Computation Graphs: PyTorch adopts a dynamic computation graph approach, allowing for more flexible and intuitive model construction. This is advantageous for tasks that involve dynamic or variable-length inputs.
Easier Debugging and Experimentation: PyTorch's imperative programming style makes it easier to debug and experiment with different architectures and techniques. It follows a "Pythonic" way of writing code.
Research-Focused Community: PyTorch has gained popularity in the research community due to its flexibility and ease of use. This has led to a rich ecosystem of research papers, models, and pre-trained weights available in PyTorch.
Natural Integration with Python: Since PyTorch is closely integrated with Python, it aligns well with Python programming paradigms and is easy to learn for Python developers.
TorchScript for Production: PyTorch includes TorchScript, a domain-specific language, which can be used to serialize and optimize models for production deployment.
Libraries like Fastai: Fastai, a high-level deep learning library built on top of PyTorch, provides simplified APIs for common deep learning tasks and includes pre-built models and training techniques.
Choosing between TensorFlow and PyTorch often comes down to personal preference, specific project requirements, and the existing ecosystem of the team or community.
10. Keras

High-Level Neural Networks API
Keras is a high-level neural networks API that can run on top of either TensorFlow or Theano. It provides a user-friendly interface for designing, training, and deploying deep learning models, making it accessible to both beginners and experienced practitioners.
Key Aspects and Applications
Simplicity and Ease of Use: Keras is known for its straightforward and intuitive API, which allows users to quickly build and experiment with neural network architectures. It abstracts many of the complexities of lower-level libraries.
Modularity and Flexibility: Keras enables the construction of models through a series of high-level building blocks called "layers." This modular approach makes it easy to assemble and customize complex neural network architectures.
Support for Multiple Backends: Keras can be configured to run on top of different backends, including TensorFlow and Theano. This provides flexibility in choosing the underlying computational engine.
Wide Range of Pretrained Models: Keras includes access to a large collection of pre-trained models through the Keras Applications module. These models are trained on massive datasets and can be fine-tuned for specific tasks.
Multi-GPU and Distributed Training: Keras supports training on multiple GPUs and distributed computing, allowing for accelerated training of large-scale models.
Integration with Other Libraries: Keras seamlessly integrates with libraries like TensorFlow and SciPy, enabling users to leverage additional functionalities for tasks like data preprocessing and optimization.
Visualizations and Callbacks: It provides tools for visualizing model architectures, monitoring training progress, and applying callbacks during training (e.g., early stopping, model checkpointing).
Transfer Learning and Fine-Tuning: Keras facilitates transfer learning, where pre-trained models can be adapted for specific tasks with relatively small datasets. This is particularly useful when working with limited annotated data.
Community and Documentation: Keras has a vibrant community with extensive documentation, tutorials, and resources. This makes it easy for users to get started and find solutions to common problems.
Model Export and Deployment: Keras models can be exported in a variety of formats, including TensorFlow SavedModel and ONNX, making it compatible with various deployment environments.
Keras' combination of simplicity, flexibility, and powerful abstractions makes it an excellent choice for rapid prototyping and experimentation in deep learning projects.
11. NLTK (Natural Language Toolkit)

Working with Human Language Data
NLTK, short for Natural Language Toolkit, is a comprehensive library for working with human language data (text). It provides a suite of libraries and programs for tasks like tokenization, stemming, tagging, parsing, and semantic reasoning, making it a powerful tool for natural language processing (NLP) tasks.
Key Functionalities and Applications
Tokenization: NLTK offers tools for breaking text into individual words or tokens. This is a fundamental step in many NLP tasks, including text analysis, sentiment analysis, and machine translation.
Stemming and Lemmatization: It provides algorithms for reducing words to their base or root form (stemming) or converting them to their canonical form (lemmatization). This is essential for tasks like text classification and information retrieval.
Part-of-Speech Tagging: NLTK includes pre-trained models for assigning grammatical tags (noun, verb, adjective, etc.) to words in a sentence. This information is valuable for tasks like syntax analysis and semantic understanding.
Named Entity Recognition (NER): It includes tools for identifying and classifying named entities (names of people, organizations, locations, etc.) in text. This is crucial for tasks like information extraction.
Parsing and Syntax Analysis: NLTK provides tools for parsing sentences and determining their grammatical structure. This can be used for tasks like dependency parsing and sentence segmentation.
Sentiment Analysis: It includes resources and pre-trained models for sentiment analysis, allowing for the classification of text as positive, negative, or neutral.
Machine Translation: NLTK includes tools for building and evaluating machine translation models, enabling the translation of text from one language to another.
WordNet Integration: NLTK integrates with WordNet, a lexical database of the English language. This provides a rich source of semantic information for tasks like word sense disambiguation.
Corpus and Language Resources: NLTK includes a vast collection of text corpora, lexical resources, and language processing tools. These resources are invaluable for training models and conducting research in NLP.
Text Classification and Categorization: It provides tools for building and evaluating text classification models, allowing for tasks like sentiment analysis, topic modeling, and document categorization.
NLTK's extensive set of tools and resources for NLP tasks makes it a go-to library for researchers, linguists, and data scientists working with text data.
12. spaCy

Advanced Natural Language Processing (NLP)
spaCy is a popular library for advanced natural language processing (NLP) tasks. It is designed for efficiency and high performance, making it suitable for processing large volumes of text data. spaCy provides a wide range of functionalities for tasks like entity recognition, dependency parsing, and more.
Key Functionalities and Applications
Tokenization and Part-of-Speech Tagging: spaCy excels in tokenizing text into words or phrases and assigning grammatical tags to each token. This is essential for various NLP tasks, including syntactic and semantic analysis.
Named Entity Recognition (NER): It includes pre-trained models for recognizing and classifying named entities in text, such as names of people, organizations, locations, etc. This is crucial for information extraction and entity linking tasks.
Dependency Parsing: spaCy provides tools for analyzing the grammatical structure of sentences, including identifying the relationships between words. This is valuable for tasks like syntax analysis and semantic understanding.
Lemmatization: It offers a lemmatizer that converts words to their base or root form. This is important for tasks like text classification and information retrieval.
Entity Linking: spaCy includes functionality for linking recognized entities to knowledge bases or databases, providing additional context and information about those entities.
Sentence Segmentation: It can segment text into individual sentences, which is an important step for various NLP tasks, including machine translation and sentiment analysis.
Word Vector Representations: spaCy provides pre-trained word vectors (word embeddings) that capture semantic similarities between words. These embeddings can be used for tasks like word similarity, clustering, and classification.
Text Classification: It includes tools for building and training text classification models, allowing for tasks like sentiment analysis, topic modeling, and document categorization.
Customizable Pipelines: spaCy allows users to customize the NLP pipeline to include specific components or functionalities based on their requirements.
Multi-Language Support: It supports multiple languages and provides pre-trained models for various languages, making it a versatile choice for global NLP projects.
spaCy's emphasis on speed, efficiency, and accuracy makes it a valuable library for researchers, data scientists, and developers working on complex NLP tasks.
13. Gensim
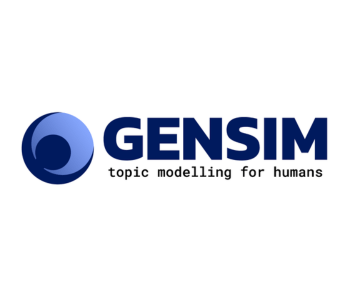
Topic Modeling and Document Similarity Analysis
Gensim is a powerful Python library for topic modeling and document similarity analysis. It is designed to work with textual data and is particularly valuable for tasks like extracting topics from a collection of documents or finding similar documents based on their content.
Key Functionalities and Applications
Topic Modeling: Gensim provides tools for performing topic modeling, which involves identifying topics in a collection of documents. This is valuable for tasks like content categorization and clustering.
Latent Semantic Analysis (LSA): It includes algorithms for performing LSA, a technique that uncovers the underlying structure in a set of documents. LSA is used for tasks like information retrieval and document summarization.
Latent Dirichlet Allocation (LDA): Gensim supports LDA, a probabilistic model that assigns topics to words and documents. LDA is widely used for uncovering themes or topics in large document collections.
Document Similarity Analysis: Gensim can calculate similarities between documents based on their content. This is useful for tasks like finding similar articles, clustering related documents, and recommending similar content.
Word Embeddings: Gensim includes tools for training word embeddings (word vectors) using techniques like Word2Vec. Word embeddings are essential for tasks like word similarity, document classification, and more.
Document-to-Vector (Doc2Vec): It supports Doc2Vec, an extension of Word2Vec that learns embeddings for entire documents. This allows for the representation of documents in a continuous vector space.
Text Summarization: Gensim can be used for extractive text summarization, where key sentences are selected from a document to create a concise summary.
Scalability and Efficiency: Gensim is designed to be memory-efficient and can handle large datasets and corpora. This makes it suitable for processing extensive collections of text documents.
Multi-Language Support: It supports multiple languages and can be used for topic modeling and similarity analysis in various linguistic contexts.
Integration with Other Libraries: Gensim can be seamlessly integrated with other NLP libraries like spaCy and NLTK, allowing for a more comprehensive analysis of text data.
Gensim's capabilities in topic modeling and document similarity analysis make it a valuable tool for researchers, content creators, and data scientists working with textual data.
14. NetworkX
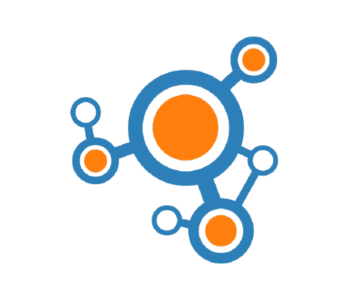
Creating, Manipulating, and Analyzing Complex Networks
NetworkX is a Python library designed for the creation, manipulation, and study of complex networks. It provides tools for modeling and analyzing the structure and dynamics of networks, making it invaluable for tasks like social network analysis, transportation networks, and more.
Key Functionalities and Applications
Graph Representation: NetworkX allows for the creation and manipulation of graphs, which consist of nodes (vertices) and edges (connections between nodes). This is essential for modeling various types of networks.
Directed and Undirected Graphs: It supports both directed graphs (where edges have a specific direction) and undirected graphs (where edges have no direction).
Graph Algorithms: NetworkX includes a wide range of algorithms for tasks like finding shortest paths, computing centrality measures, detecting communities, and more. These algorithms are crucial for analyzing network properties.
Centrality Measures: It provides tools for computing centrality measures, such as degree centrality, betweenness centrality, and eigenvector centrality. These measures help identify important nodes in a network.
Community Detection: NetworkX includes algorithms for detecting communities or clusters within a network. This is valuable for understanding the structure and organization of complex networks.
Graph Visualization: It offers basic tools for visualizing graphs, allowing users to create visual representations of network structures.
Network Properties and Metrics: NetworkX provides functions for computing various metrics and properties of networks, including diameter, clustering coefficient, and assortativity.
Graph Generators: It includes a collection of generators for creating standard graph types (e.g., complete graphs, random graphs) as well as more complex network models (e.g., small-world networks, scale-free networks).
Graph I/O: NetworkX supports reading and writing graphs in various file formats, allowing for easy integration with external data sources.
Multi-Graphs and Multi-Digraphs: It can handle graphs with multiple edges between nodes and directed graphs with multiple edges.
NetworkX's capabilities in network modeling and analysis make it a valuable tool for researchers, data scientists, and engineers working on a wide range of network-related problems.
15. Beautiful Soup
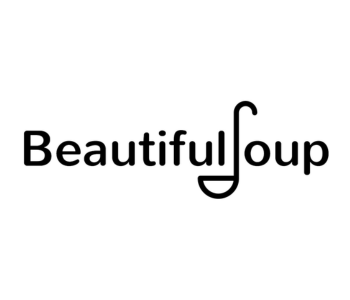
Web Scraping for Data Extraction
Beautiful Soup is a Python library used for web scraping purposes. It provides tools for parsing HTML and XML documents, navigating their structures, and extracting relevant information. This makes it a valuable tool for data scientists and researchers who need to gather data from websites.
Key Functionalities and Applications
HTML and XML Parsing: Beautiful Soup can parse HTML and XML documents, allowing users to navigate the document's structure and extract specific elements.
Tag and Attribute Selection: It provides methods for selecting specific HTML tags and their attributes, making it easy to target and extract the desired content.
Navigating the Document Tree: Beautiful Soup allows for navigation through the document's tree structure, including moving up and down the hierarchy of elements.
Searching and Filtering: It supports powerful searching and filtering operations based on CSS selectors, tag names, attributes, and more. This enables precise targeting of elements for extraction.
Extracting Text and Attributes: Beautiful Soup allows users to extract the text content of elements as well as their attributes, which can be valuable for data collection.
Handling Different Encodings: It automatically converts incoming documents to Unicode, ensuring compatibility with various encodings.
Robust Error Handling: Beautiful Soup handles poorly formatted or incomplete HTML gracefully, making it robust for real-world web scraping tasks.
Integration with Requests: It is commonly used in conjunction with the Requests library, allowing for seamless HTTP requests and subsequent parsing of the retrieved content.
Web Page Crawling: Beautiful Soup can be used in combination with other libraries to crawl multiple pages within a website and extract data from each page.
Data Extraction for Analysis: Once data is extracted, it can be further processed and analyzed using other Python libraries for tasks like data cleaning, transformation, and visualization.
Beautiful Soup's ability to parse and extract data from web pages makes it an essential tool for data scientists who need to collect information from the internet for analysis and research.
16. Requests
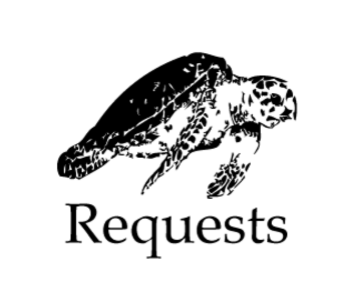
Sending HTTP Requests
Requests is a Python library used for sending HTTP requests to web servers. It provides a simple and intuitive interface for making various types of requests (e.g., GET, POST) and handling responses. This makes it a fundamental tool for data scientists and developers working with web-based APIs and services.
Key Functionalities and Applications
Making HTTP Requests: Requests allows users to send HTTP requests to web servers, enabling interactions with web-based resources, APIs, and services.
Support for Different HTTP Methods: It supports various HTTP methods, including GET (retrieve data), POST (submit data), PUT (update data), DELETE (remove data), and more. This versatility is essential for interacting with different types of resources.
Passing Parameters and Data: Requests enables users to include parameters and data in their requests, allowing for customization of the request payload.
Handling Headers and Cookies: It provides options for setting custom headers and sending cookies along with the request, which is crucial for authentication and session management.
Handling Authentication: Requests supports basic authentication, as well as handling more complex authentication mechanisms like OAuth
Handling Response Content: It allows for easy access to the content of the HTTP response, whether it's HTML, JSON, XML, or other formats.
File Downloads: Requests can be used to download files from the web, making it useful for tasks like data acquisition and scraping.
Session Management: It supports sessions, allowing users to persist certain parameters or settings across multiple requests. This is useful for scenarios that require maintaining a session state.
Timeouts and Error Handling: Requests provides options for setting timeouts on requests to prevent them from hanging indefinitely. It also includes mechanisms for handling errors and status codes.
SSL Certificate Verification: It supports SSL certificate verification for secure and encrypted connections.
Requests' simplicity and flexibility make it a go-to library for data scientists and developers who need to interact with web-based resources, APIs, and services as part of their workflow.
17. Flask or Django

Web Application Development (Optional but Useful for Deploying Data Science Models)
Flask and Django are both popular Python web frameworks used for building web applications. While not strictly necessary for data science, they can be immensely useful for deploying data science models and creating interactive web-based tools for data analysis.
Key Aspects and Applications
Flask:
Micro Framework: Flask is a micro web framework, which means it provides the essential components for building web applications without imposing too much structure. This allows for flexibility and customization.
Lightweight and Minimalistic: Flask is designed to be lightweight and follows a minimalistic approach, making it easy to get started and suitable for small to medium-sized projects.
Extensible with Extensions: It can be extended with various Flask extensions, allowing users to add functionalities like authentication, database integration, and more.
Jinja Templating: Flask integrates with the Jinja templating engine, which facilitates the rendering of dynamic content in HTML templates.
RESTful API Development: Flask is well-suited for building RESTful APIs, making it a good choice for creating API endpoints to serve data or model predictions.
Django:
Full-Featured Framework: Django is a high-level, full-featured web framework that provides a comprehensive set of tools and components for building robust web applications.
Built-in Admin Interface: Django includes a built-in admin interface that allows for easy management and administration of the application's data models.
ORM (Object-Relational Mapping): It comes with a powerful ORM system that simplifies database interactions by abstracting SQL queries into Python code.
Authentication and Authorization: Django provides built-in mechanisms for user authentication, authorization, and access control, making it well-suited for applications with user management.
Batteries Included: Django follows the "batteries included" philosophy, which means it comes with a wide range of built-in features and functionalities, reducing the need for external libraries.
Form Handling and Validation: Django includes a robust system for handling HTML forms, including form validation and processing.
Security Features: Django incorporates built-in security features like protection against common web vulnerabilities, making it a secure choice for web application development.
Scalability: While Django is feature-rich, it is designed to scale, allowing it to handle large and complex applications.
The choice between Flask and Django depends on the specific requirements of the project. Flask is well-suited for small to medium-sized projects and provides flexibility, while Django is ideal for larger, more complex applications with built-in features.
18. Bokeh or Plotly
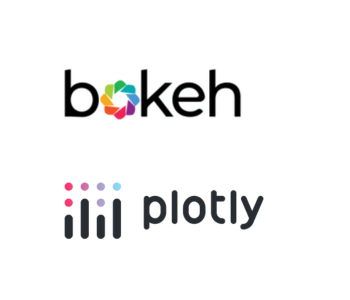
Interactive and Dynamic Data Visualization
Bokeh and Plotly are both Python libraries used for creating interactive and dynamic data visualizations. They provide tools for generating a wide range of visualizations, including plots, charts, and dashboards, making them valuable for conveying insights from data.
Key Aspects and Applications
Bokeh:
Interactive Web-Based Visualizations: Bokeh is designed for creating interactive and visually appealing plots that can be embedded in web applications.
High-Level and Low-Level Interfaces: It offers both high-level interfaces for creating common chart types (e.g., scatter plots, bar charts) and low-level interfaces for fine-grained control over visual elements.
Streaming Data: Bokeh includes features for handling streaming data, allowing for real-time updates in visualizations.
Server Integration: Bokeh can be used with the Bokeh server, which enables the creation of interactive, data-driven applications with server-side processing.
Integration with Jupyter Notebooks: It seamlessly integrates with Jupyter Notebooks, allowing for interactive data exploration and visualization within the notebook environment.
Plotly:
Wide Range of Chart Types: Plotly provides a comprehensive set of chart types, including line charts, bar charts, heatmaps, 3D plots, and more.
Interactive Dashboards: It excels in creating interactive dashboards with multiple linked visualizations, allowing for comprehensive data exploration.
Integration with Web Frameworks: Plotly can be integrated with web frameworks like Dash, which enables the creation of full-fledged web applications with interactive data visualizations.
Exportable and Shareable: Plotly visualizations can be easily exported as standalone HTML files or embedded in web pages, making them shareable across platforms.
3D and Geographic Visualizations: Plotly offers robust support for 3D visualizations and geographic maps, making it suitable for applications that require spatial or three-dimensional representation.
Customizable Themes and Styles: It provides options for customizing the appearance of visualizations, including themes, colors, and styles.
Both Bokeh and Plotly are powerful tools for creating interactive visualizations. The choice between them may come down to personal preference, specific project requirements, and the desired level of interactivity.
19. Scrapy

Web Crawling and Scraping
Scrapy is a powerful Python framework used for web crawling and scraping. It provides a structured way to extract data from websites, making it a valuable tool for data scientists and researchers who need to gather information from the web for analysis.
Key Functionalities and Applications
Crawling and Spidering: Scrapy allows users to define "spiders" that navigate websites and extract specific information from the pages. This enables automated data collection from multiple pages or websites.
XPath and CSS Selectors: It supports XPath and CSS selectors for targeting specific elements on web pages, making it easy to locate and extract desired content.
Item Pipelines: Scrapy includes item pipelines for processing the extracted data. This allows for tasks like data cleaning, validation, and transformation before saving the data.
Asynchronous Requests: Scrapy is designed to handle multiple requests simultaneously, making it efficient for scraping large volumes of data from multiple sources.
Robust Error Handling: It includes mechanisms for handling common web scraping challenges, such as handling timeouts, retries, and avoiding getting banned by websites.
HTTP Cache: Scrapy supports caching, which can help reduce the load on target websites and speed up the scraping process for recurrent visits.
Exporting Data: It provides built-in support for exporting scraped data in various formats, including JSON, CSV, and XML.
Middleware Support: Scrapy allows for the customization of request/response handling through middleware, enabling users to add custom functionality to the scraping process.
Distributed Crawling: It can be used in conjunction with tools like Scrapyd or Scrapy Cloud for distributed crawling across multiple machines or cloud environments.
Respectful Scraping: Scrapy encourages ethical scraping practices by allowing users to set crawl delays, respect robots.txt files, and avoid overloading servers.
Scrapy's structured approach to web scraping and its powerful features make it a preferred choice for projects that require systematic data extraction from websites.
20. LightGBM

Gradient Boosting Framework for Machine Learning
LightGBM is an efficient and distributed gradient boosting framework designed for training large-scale machine learning models. It is particularly well-suited for tasks involving large datasets and complex models, making it a valuable tool for data scientists and machine learning practitioners.
Key Aspects and Applications
Gradient Boosting Algorithm: LightGBM is based on the gradient boosting algorithm, which sequentially builds an ensemble of weak learners (usually decision trees) to improve predictive performance.
Efficient and Fast: It is optimized for speed and efficiency, making it capable of handling large datasets with millions of samples and features. LightGBM is known for its high training speed and low memory usage.
Leaf-Wise Growth Strategy: LightGBM uses a leaf-wise growth strategy instead of a level-wise strategy. This leads to a reduction in the number of splits, resulting in faster training times.
Categorical Feature Support: It provides native support for categorical features without the need for one-hot encoding, reducing memory consumption and speeding up training.
Gradient-Based One-Side Sampling: LightGBM uses gradient-based one-side sampling, which focuses on the data points that contribute more to the gradients during the training process. This further improves efficiency.
Distributed and GPU Training: It supports distributed training across multiple machines and can leverage GPUs for even faster training times.
Regularization and Control Parameters: LightGBM offers a range of parameters for controlling the model's complexity, including L1 and L2 regularization. This helps prevent overfitting.
Hyperparameter Tuning: LightGBM provides tools for hyperparameter optimization, allowing users to find the best set of parameters for their specific task.
Interpretability and Feature Importance: It includes features for interpreting the model's predictions and assessing the importance of different features in the model.
Wide Range of Applications: LightGBM can be used for various machine learning tasks, including classification, regression, ranking, and more.
LightGBM's efficiency and effectiveness in handling large datasets and complex models make it a powerful choice for machine learning projects, especially those where speed and scalability are critical.
21. XGBoost

Popular Gradient Boosting Library
XGBoost (eXtreme Gradient Boosting) is a widely used open-source library for gradient boosting. It is known for its high performance and accuracy in a wide range of machine learning tasks. XGBoost is a versatile tool that can be applied to both regression and classification problems.
Key Aspects and Applications
Gradient Boosting Algorithm: XGBoost employs the gradient boosting algorithm, which sequentially builds an ensemble of weak learners (typically decision trees) to improve predictive accuracy.
Regularization and Control Parameters: It includes a range of parameters for controlling the model's complexity, including L1 (Lasso) and L2 (Ridge) regularization. This helps prevent overfitting.
Handling Missing Values: XGBoost has built-in support for handling missing values in the dataset, reducing the need for data preprocessing.
Flexibility in Tree Construction: It offers flexibility in tree construction, allowing users to specify different criteria for making splits (e.g., gain, coverage).
Cross-Validation: XGBoost provides built-in support for cross-validation, allowing users to assess the model's performance and tune hyperparameters.
Ensemble Learning Techniques: It can perform both bagging and boosting, allowing for the combination of multiple models to improve predictive accuracy.
Parallel and Distributed Computing: XGBoost is designed for efficiency and can take advantage of multiple cores on a single machine. It also supports distributed computing for training on large datasets.
Support for Custom Loss Functions: It allows users to define and use custom loss functions, providing flexibility in model training.
Feature Importance Analysis: XGBoost provides tools for assessing the importance of different features in the model, helping to identify the most influential variables.
Wide Range of Applications: XGBoost can be applied to various machine learning tasks, including classification, regression, ranking, and more.
Integration with Python and Other Languages: XGBoost can be seamlessly integrated with Python, as well as other programming languages like R, Java, and Julia.
XGBoost's combination of accuracy, speed, and flexibility has made it a popular choice among data scientists and machine learning practitioners for a wide range of applications.
Future of Python For Data Science
As we step into 2024, Python's influence on the field of data science shows no signs of waning. Several trends and advancements are expected to shape the landscape of data science in the coming year:
1. Enhancements in Deep Learning Frameworks
TensorFlow, PyTorch, and related deep learning libraries are anticipated to undergo significant updates, further empowering researchers and practitioners in the realm of neural networks.
2. Rise of Explainable AI
Libraries like ELI5 (Explain Like I'm 5) are gaining traction, providing interpretable explanations for machine learning models. This trend is crucial for building trust and understanding in AI-driven solutions.
3. Advancements in Natural Language Processing (NLP)
With the increasing demand for language understanding applications, libraries like spaCy and NLTK are expected to introduce new features and models for NLP tasks.
4. Continued Growth of Data Visualization Libraries
Tools like Bokeh, Plotly, and Matplotlib are likely to evolve with enhanced features for interactive and dynamic data visualization, catering to the growing need for compelling data storytelling.
5. Expansion of AutoML Capabilities
Libraries and platforms facilitating Automated Machine Learning (AutoML) are projected to become more sophisticated, allowing for even easier implementation of machine learning models by non-experts.
6. Integration of Quantum Computing Libraries
With advancements in quantum computing, Python libraries like Qiskit and Forest are expected to play a significant role in quantum machine learning and optimization tasks.
These anticipated trends underscore Python's pivotal role in driving innovation and progress within the field of data science.
Conclusion
In the ever-evolving landscape of data science, having a solid grasp of the essential Python libraries is paramount. Python's simplicity, extensive library ecosystem, and supportive community make it the linchpin of data science in 2024.
From NumPy's numerical computing prowess to the advanced statistical analysis capabilities of Statsmodels, each library plays a unique role in empowering data scientists to tackle complex challenges. Whether you're delving into machine learning with Scikit-learn or unraveling the mysteries of natural language with NLTK, Python has a library tailored to your needs.
As we look ahead, the future of Python in data science promises even greater advancements. Deep learning frameworks like TensorFlow and PyTorch are set to reach new heights, while the demand for explainable AI solutions continues to grow. With Python libraries at the forefront, the possibilities for innovation are boundless.
So, as you embark on your data science journey in 2024, remember to harness the power of these 21 must-know Python libraries. They are the building blocks of groundbreaking discoveries and transformative insights that will shape the future of data science.