Table of content
Generative AI is a revolutionary advancement in the tech world that has taken businesses and decision-makers by storm. With everyone using AI models like ChatGPT and Stable Diffusion to speed up development processes, it's safe to say that generative AI is here to stay.
Jasper, one of the successful generative AI tools, has raised $125 million at a valuation of $1.5 billion by assisting businesses with copywriting. Similarly, Stability AI has made $101 million with a valuation of $1 billion. But the most significant achievement is that of OpenAI, which generated over $1 billion from Microsoft at a valuation of $25 billion in 2019.
Clearly, the scope for generative AI solutions is broadening. Read on to learn everything you need to know about how to build a generative AI solution from scratch.
What is Generative AI?
Back in the 1950s, researchers first explored the basic principles of artificial intelligence and generative AI. The initial focus was on simple neural networks and rule-based models to mimic human decision-making.
On the surface, generative AI is an exciting new development where deep learning algorithms are used to create content, graphics, music, and more. Large datasets are used as input for deep learning algorithms that can generate unique content. What makes generative AI special is its ability to generate entirely new and unique outputs, unlike traditional AI which only delivers pre-programmed outputs.
When trained, the AI algorithms learn patterns from given inputs, store them, and utilize them to create unique content for similar inputs. Even though it looks easy, generative AI becomes quite complicated when you dig deeper. For instance, there are various components like transformers, generative adversarial networks (GANs), and variational auto-encoders behind the functionality of generative AI.
Examples of transformers include ChatGPT, LaMDA, Wu-Dao, and GPT-3. Transformers are trained to process natural language and images, as well as learn classification tasks and generate texts. These techniques are designed to mimic cognitive attention and understand the difference between varying inputs.
What Can Generative AI Do?
Generative AI is helping businesses worldwide by generating content in the form of text, images, and videos. This means voice-over and background music in videos are not a problem anymore. Generative AI is also used in data augmentation to boost the accuracy of predictive machine-learning models. As a result, retailers can offer highly personalized experiences to customers.
The following are some of the diverse application areas of generative AI across various industries:
Art and Creativity
The new, state-of-the-art algorithms of generative AI enable you to convert any ordinary picture into an art piece. You can guide the AI tool to add your unique style, features, and other favorite items. Even when you provide the generative AI tool with a rough sketch of the image you want to create, it generates photorealistic masterpieces that go beyond your expectations. Not only that, but the AI can also imitate specific styles of a given human artist, which allows you to create art like never before.
Content Generation
When it comes to automating content creation, you don’t need to look further than generative AI. It has provided marketers with the ideal solution to save time and resources. With automated content generation, you can achieve faster time-to-market and create a variety of campaigns. For instance, you can create social media posts and blog articles, and even design email campaigns.
AI tools like ChatGPT, Jasper, Copy.ai, and Writesonic have accelerated the process of content generation. In some cases, marketers can personalize and refine drafts generated by AI models. Not only that but generative AI can also be used to update existing content with valuable inputs and improvements.
Natural Language Processing
Generative AI is commonly used in natural language processing to create social media posts and news articles. Various natural language processing techniques are used to generate the text for such posts. These techniques includes transforming raw characters like words, letters, and punctuation into sentences. The resulting entities and actions are represented in the form of vectors through multiple encoding mechanisms.
Image Synthesis
Today’s businesses want to represent their products and services with realistic graphics and lifelike images. Here, AI algorithms assist businesses with accurate image synthesis. You can eliminate misleading aspects from your images and fill in the missing elements to portray your products in the best way.
Medical Imaging
That’s not all - AI tools can also convert low-resolution images into high-resolution images, making them look like they were captured by professional photographers. With generative AI by your side, your images are transformed into clear and detailed masterpieces. This is particularly useful in medical imaging analysis, where accuracy is of utmost importance.
Generative AI is used in medical imaging for specific tasks like image segmentation, anomaly detection, and prediction of patient outcomes.
Anomaly Detection
Business owners need to go through large amounts of data to understand patterns in order to make informed decisions. In this case, generative AI helps detect anomalies in a series of data. Assuming a certain range of data based on historical trends, AI can point out values that fall outside that threshold and happen less frequently.
How Generative AI is Changing Industries
The world knows generative AI as a buzzword because of its impact on different industries. It has quickly transformed business operations and is creating new opportunities across the board. Apart from creating novel, unique content and making way for innovations, generative AI is changing the way processes are carried out.
Here are some examples of how generative AI is transforming various sectors:
Entertainment and Media
Two of the many benefits of generative AI are democratized content creation and simplified management. In the entertainment industry, AI helps accelerate research tasks and allows filmmakers to improve the efficiency of their post-production operations. The data processing power of AI makes video libraries more searchable, making life easier for broadcasters. For this reason, the market size of generative AI is expected to grow to $12,077 Million in 2032 at a CAGR of 26.7%
Healthcare
Whether it is medical diagnosis, imaging, or analysis of a large number of patient records, AI is always good news for the healthcare industry. You can now leverage AI-based disease prediction and diagnosis to improve healthcare operations. Generative AI monitors various factors like lifestyle risk factors, medical imaging, patient health data, environmental factors, and genetic data to provide accurate results. Moreover, statistics show that 27.5 percent of health systems currently use conversational AI, with a further 72.5 percent considering it for future use.
Marketing and Advertising
Until a few years ago, marketing and advertising agencies required graphic designers and content creators to create their campaigns. But now, AI is being used by 53% of IT companies for marketing and advertising. With generative AI, this process is a matter of a few prompts. You can prompt AI tools to create unique and original graphics for blog posts, social media posts, and more.
Gaming
Game development is a complex and time-consuming process that involves various tasks. Like other industries, generative AI becomes useful in game development by automating complex tasks. These include creating assets, levels, and generating dialogues. By utilizing generative AI to handle these tasks, game developers are able to unlock creativity and use the extra time to work on the strategic aspects of their development cycle. Generative AI in the gaming sector is expected to achieve the highest market value with a valuation of $7,105 Million by 2032.
Manufacturing and Design
Now here’s an industry that focuses on optimized production and quality controls along with great customer service. By processing raw data and helping in the production of valuable products, generative AI significantly improves manufacture and design operations. According to Gartner, by 2025, generative AI will account for 10% of all data produced, up from less than 1% today. It helps enhance customer service, streamline production processes, and also improves quality control procedures.
The Right Tech Stack to Build a Generative AI Solution
Before getting into the process of building your own generative AI solution, you need to know the right tools and technologies to use. The following is a comprehensive list of the tech stack you will need to use:
Deep learning framework | TensorFlow, PyTorch |
---|---|
Generative Model Architectures | GANs, VAEs |
GPU Acceleration | CUDA, cuDNN |
Data Processing | Pandas, NumPy |
Image Processing | OpenCV, PIL |
Cloud Resources | Amazon Web Services (AWS), Microsoft Azure, Google Cloud |
Model Deployment | Docker, Kubernetes, TensorFlow Serving, Flask, FastAPI |
Version Control | GitHub or GitLab |
Experiment Tracking | TensorBoard, MLflow |
Looking for a Custom Generative AI Solution?
Get in Touch with shopdev’s AI/ML Developers Today!
7 Steps to Build a Generative AI Solution from Scratch
In order to build a generative AI solution, you need to develop a comprehensive understanding of its purpose and the problem it will solve. The process involves creating and training AI models to generate unique outputs depending on varying inputs.
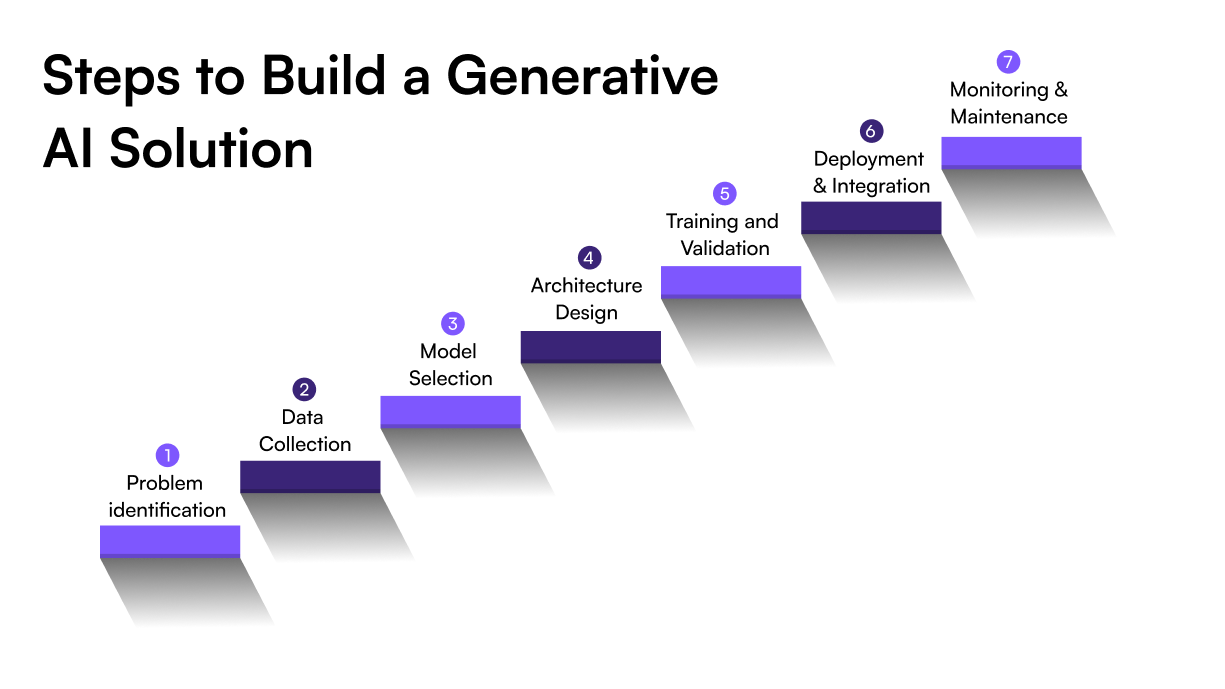
As today’s generative AI solutions are capable of optimizing and improving operations, building a satisfactory solution requires you to follow a series of steps.
Problem Identification
Since you are dealing with a lot of computing power in generative AI, you need to be sure that they are designed to solve the right problem. The first step in building your generative AI solution from scratch is to identify a set of problems to solve. For instance, marketing agencies need various types of content for their blog and social media platforms. In this case, your AI solution needs to be built around natural language processing, neural networks, and generative pre-trained transformers. In other cases such as video and audio generation, you will need to adopt a different approach.
Data Collection
Data collection is one of the important steps in the prototyping phase of generative AI development. Since the training of your generative AI solution depends on data, you need to consider certain technical details. These include:
- Identifying the right data sources
- Ensuring that high quality, relevant, and diverse data is collected
- Labeling the data if needed using crowdsourcing, semi-supervised learning, or active learning
- Preprocessing data through data augmentation, tokenization, or normalization before it is fed to the AI model
- Splitting the data into separate categories for training, validation, and testing
- Storing the data in data warehouses, distributed file systems, or cloud storage depending on the scenario.
Model Selection
Based on the defined problem that the AI solution is intended to solve, you need to select the right model and tech stack to be used in the development. To be able to choose the right model, you need to categorize the problem and determine the right algorithms to solve it.
For instance, if you’re working with input categorization, you need to select the supervised learning model. For imaging systems that can differentiate objects from images, you can work with OpenCV. On the other hand, you can also use Jupyter Notebook to allow seamless collaborations between machine learning engineers, developers, and data scientists.
Architecture Design
In order to ensure that your generative AI solution can process large amounts of data and handle high traffic, you need to base it on a robust and scalable architecture. The common purpose of all generative AI solutions is to maintain high performance, reliability, and availability in all situations. The best practices to build the right architecture are as follows:
- Break down the AI solution into small, manageable components through microservices architecture
- Adopt a modular design where each module performs its specific function
- Incorporate message queues to efficiently manage communication between different components of the AI solution
- Implement load balancing to ensure equal traffic distribution across multiple servers and get peak performance
- Implement caching to reduce backend requests by storing frequently accessed data
- Configure hyperparameters of the generative AI model to set number of layers, learning rate, batch size, regularization techniques, dropout rate, etc.
Training and Validation
In the training phase, you feed the AI model with datasets you previously collected and categorized. With these datasets, the generative AI model learns patterns that are later used to solve problems and create content. In order to train generative AI models, you need to use self-supervised learning and semi-supervised machine learning methods. Although the process is machine dominant, you need to add the human touch to fine-tune and set the AI model’s accuracy and align it with your business objectives.
Deployment and Integration
While deploying your AI solution, you need to be sure of its reliability, scalability, and maintainability. This is particularly important to eliminate errors and failures and ensure the smooth functioning of your generative AI solution. Here’s what you need to consider in the deployment and integration phase:
- Choose the right deployment environment by setting up on-premise infrastructure, cloud computing systems, or hybrid solutions depending on the requirements.
- Implement the right hardware and software for installing operating systems, setting up databases, and configuring servers.
- Set up dependencies like libraries, frameworks, and packages required to run your AI solution.
- Use tools like Jenkins, Travis CI, or GitLab to set up continuous integration and deployment (CI/CD) pipelines.
Monitoring and Maintenance
The last step of the process holds equal significance. Upon deployment, you need to constantly provide support and maintenance while evaluating how the AI solution performs in real-life use cases. The key is to identify bottlenecks and failures in order to fix and improve the solution. Upon further testing, you can refine your AI solution by studying user feedback and performance benchmarks.
Looking for a Custom Generative AI Solution?
Get Started with shopdev’s AI/ML Developers
Pro Tips for Crafting Effective Generative AI Solutions
Even when you follow the best practices to build your AI solution, there are always going to be some unexpected issues and challenges along the way. Based on research in the field of generative AI, here are a few pro tips that can be helpful.
- Apart from identifying a problem to solve, you should also identify specific business processes that can be enhanced by using generative AI. Focus on small changes with meaningful outcomes.
- Ensure data protection to provide users the confidence to use your generative AI solution and stay compliant with international regulations. Use data encryption to reduce risks of data leaks.
- Instead of spending big bucks on training AI software like ChatGPT, you should build smaller, more targeted AI models. These can be trained to solve business problems unlike ChatGPT.
- Since the quality of data matters, your focus should be on preparing the data for training the AI model. Try to eliminate under-generalization and bias.
- Regardless of how well you train generative AI, it can never be perfect. Therefore, you need to inform your users about the limitations of your AI solution.
Costs of Generative AI Solution Development
Depending on the type, complexity, and size of the application you are trying to build, generative AI development becomes quite a costly process. This means you can expect a bill between $10,000 and $500,000. The most notable costs include training the AI model and deploying it. The software cost itself is between $35,000 to $120,000, while maintenance costs can go beyond $320,000 per year.
That’s not all, you also need to invest in specialized hardware and cloud storage to get the right computing power and storage capacity. Depending on the complexity of your solution, this can cost you between $100 and $15,000 a month.
Looking for a Custom Generative AI Solution?
Explore shopdev’s AI/ML Software Development Services
FAQs
Can I make my own generative AI?
You can create your own generative AI by adopting, modifying, and building the models you require. However, the process is quite complex and you need an increasing number of resources as you continue building your generative AI. You also need to have technical expertise in the fields of natural language processing, machine learning, and computer vision.
How do I start generative AI?
First of all, you need to understand the different generative AI models available in the market. You should consider the unique features and applications of GANs, autoregressive models, and VAEs. You need to gather and prepare the data you want to work with and choose the type of AI architecture to use. Then you need to train your AI model and evaluate, optimize, and test it in a real-world environment.
Does generative AI use NLP?
Yes, generative AI leverages natural language processing to create new outputs or content for each input. The use of NLP means that you can also generate all types of content including text, images, audio, and video.
Is generative AI free to use?
There are free versions of generative AI tools like ChatGPT 3.0 and DALL-E 2. These are great for beginners who want to try generating content using AI. AI tools by OpenAI allow you to generate content by simply creating an account on their website and entering your prompts in the search bar.
Key Takeaways
In the era of artificial intelligence, businesses are being driven by generative tools that enhance operational efficiency and also help target the right customers. With businesses adopting generative AI solutions, there really is no limit to what can be achieved in the near future. We can expect unprecedented business growth with the help of efficient processes, ease of use, and the automation of complex operations. Based on the steps discussed in this blog, you can build your generative AI solution and embrace its transformative power in your business use cases.