Explore the Boundless World of Insights and Ideas
Latest blogs
How to Start an eCommerce Business with Low Budget in 2024
The eCommerce business industry has expanded faster than ever in the recent COVID-19 crisis. Due to lockdown measures, online shopping was once a convenience, and luxury has become necessary. Check out the following statistics;
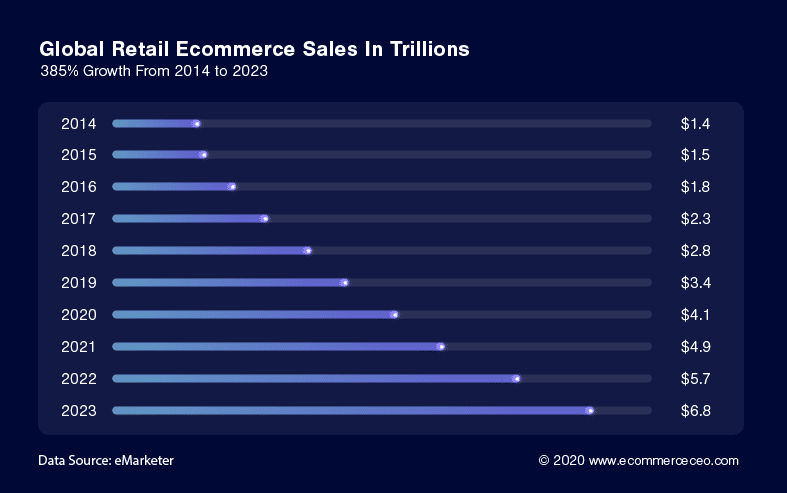
A lot of people want to explore this area and show overwhelming interest in starting an eCommerce store. If your objective is to start earning a bit, the goal should be a scalable & profitable strategy having a long-term impact. If you want to start an e-commerce business that offers a notable returns over minimal investment, this blog post will guide you along the way.
How to Start an Ecommerce Business out of Scratch
There's nothing more appealing than starting a business from scratch and watching it sustain. You build it up once, and no one can snatch it from you. eCommerce is a skyrocketing business. But we can see too many eCommerce startups fail to get proper traction.
Looking for eCommerce Consultancy? Talk to an eCommerce Expert
Building an eCommerce platform requires more than just choosing a brand name, writing product listings, and selling products online. It's a proper recipe, and with wrong ingredients and choices, even the best business ideas can crash. Now let's discuss in detail and discover the roadmap to start an eCommerce business from scratch.
Step 1: Choose From Different Ecommerce Business Models
Starting your research is the first vital step. Keep in mind that growing any eCommerce business is an investment in time, money, and energy. So treat it accordingly, and no need to hustle.There isn't an absolute business plan that works for everyone. As we hear today, the types of businesses, like Service-based businesses, software products, and numerous physical products, are just the tip of the iceberg.Before deciding what to sell on your eCommerce platform, you need to learn how to differentiate between the different business models available as it impacts your business structure.If you want to start getting a profit without investing heavily, dropshipping or print on demand is the best choice ever.If you are gearing up to set up your warehouse full of stuff, you're investing too much upfront and working with a wholesaling or warehousing (retail) model.
Want to launch your brand? Must look deeper into what white labeling and manufacturing may offer you.And then, there is the subscription option, where you can custom build a category of products or a single product to be delivered regularly to your customers.The eCommerce business model that suits newcomers is a single product category that you supplement with affiliate marketing. You can focus your content marketing and branding efforts on a single product and utilize the rest of your energy and time on driving sales by capitalizing on the traffic.
Step 2: Perform eCommerce Niche Research
It's disappointing to see that people have eCommerce platforms filled with hundreds of products, dozens of categories, and no real focus.Unless you have an overwhelming investment, you can't be the next Amazon. You have to niche down your product choices to boost your return on investment (RoI).
Choosing your niche is the most crucial step in starting your eCommerce business. Start this practice by identifying successful businesses already working in this domain.Must ensure that the domain is highly competitive because an absence of potential rivals usually reveals no market.Refrain from pushing yourself in an overly crowded niche, and dodge anything dominated by major brands. If you're facing problems with this, dig down deeper and find what you want to do – the more relative you are, the less competition you are likely to experience.
Niche-ing down also gives you the perks of having numerous partnership options. Related to what you do, but not identical, you can collaborate with other businesses in those niches, turning your rivals into partners and expanding your customer base.Don't forget to choose a product category with at least 1000 plausible keywords and focus on a niche that shows positive social media platforms.
Step 3: Validate Market Niche and Product Choice
Now that you've chosen a niche and business model, you might be eager to start acquiring products to sell. Don't hustle. Before you think about product ideas, ponder over your targeted audience. You can't expect people to buy your product if you don't know who you're selling to. Similarly, self-consciousness is equally essential. Ask yourself these questions.
- Who are you?
- What does the store represent?
- Who are your ideal customers?
- What are the demographics of your customers?
You need to maintain a consistent brand image that can promise you a long-lasting profit. Luckily, Facebook allows us to find our target audience online. Specifically, determining how many customers, you can engage. You can dig deeper to get very dedicated numbers and detailed demographics. You'd be surprised to know that most entrepreneurs have no idea how many people are in their target audience. Once you've chosen the brand outlook you want to showcase and the customer you will engage, it's the right time to think about product ideas. Don't put everything at risk. You can use affiliate marketing to validate your idea. On getting a reasonable response, you can consider making your brand of that product.Before investing in a certain product, perform a SWAT analysis carefully. Even if you opt for a dropshipping business model, you must test it carefully and get a feel for that certain item yourself so you can foresee any possible issues and prepare a customer service response to address frequent questions.The segment related to the validation of the idea is about determining its viability. Again, SWAT analysis comes into action here. Can you find the right suppliers that sync with your price model? What happens if your supplier leaves the table in between? Is there any backup plan?
Step 4: Register Your eCommerce Business & Brand Name
If you want to make a grand launch in the eCommerce market, you need a brand that showcases your identity. Acknowledging your persona makes it easier to set up an eCommerce brand. You might refrain from choosing multiple and contrasting colors and images if you sell products to corporate customers interested in living a minimalist life.But before you set up your eCommerce store and get into the trouble of building a brand, you should follow a recommended roadmap. Let's discuss it step-by-step.
- Choose a brand/business name and get your company registered, as some legal protections and tax benefits are involved.
- The domain of your eCommerce site and the legal name of your business doesn't need to be the same, but keeping identical is a good practice.
- You'll need to have an Employer Identification Number (EIN) to open a business bank account, even if you don't plan or have any employees. Your EIN serves as your business's social security number that identifies your business and helps you handle necessary paperwork or legal activities.
- Operating an online business does not give you a green channel to walk away from acquiring specific business licenses and permits. Determine and sort it out according to the rules and regulations of your country, state, or region.
- You'll have many rivals ready to give you competition on every aspect from aggressive pricing to quality and service, so it must be your priority to explore the best quality products at the best prices. Keep exploring until you find the right vendor.
- Ensure that your logo doesn't resemble any other company specifically in your niche.
- Must rethink and reconsider the colors of your brand, the images you'll use, and the font type you'll choose. If you've got a little more budget, don't hesitate to hire a marketing firm to create a design for your company. If not, you can create your own on different online logo makers.
Step 5: Evaluate and Finalize Your eCommerce Business Plan
For now, you have got a tremendously rich idea about the overall appearance of your business. You have your business model, a target market, a product niche, and your very own brand name.It's high time now to put your business plan on paper and finalize your plan by considering your startup budget, loan requirements (if any), and monthly expenditure. The financial question is the essential aspect of any business.
Determine your break-even point, both in unit sales and duration (in months). Any real-time business practice is fundamentally an investment of resources and capitalization over these resources. You are in deep trouble if you fail to determine your profit margin.The business planning phase must include the details of your staff (if any), product sourcing, logistics layout, and marketing budget. Ensure that you acknowledge all the financial resources available at the time.
Step 6: Create Your eCommerce Store
Once you're officially an eCommerce business owner, you must register your relevant domain name. You have to choose the appropriate design for your eCommerce store.There are numerous eCommerce shopping cart platforms. Choosing the right eCommerce platform is not easy. You need to consciously evaluate the essential factors like loading speed, features, compatibility with various payment gateways, your business structure, SEO features being offered, and more. Some of the essential eCommerce platforms available include:
- Magento
- WooCommerce
- Shopify
- OpenCart
- BigCommerce
You can explore more about these platforms and the drop-shipping options in our similar blog post: How to Launch Your Dropshipping Store.Once you opt for your eCommerce platform, You can select many themes offered by the aforementioned platforms.If you don't want to indulge in credit and debit payments issues, you can sell products online on an existing marketplace like Amazon. Keep in mind, setting up your eCommerce store requires more than just adding your products and content. It would be best to prepare your email marketing and automation setup.
For a smooth order fulfillment process, consider using dedicated shipping software to automate shipping label creation, tracking, and delivery notifications. This ensures a seamless experience for your customers and helps streamline your operations as your business grows.
Step 7: Driving Traffic to Your eCommerce Store
Want to skyrocket your reach and engagement? SEO is the key to unlocking this potential. You need to keep keywords and search terms in mind on each page of your eCommerce site, in your URLs, and your marketing campaigns. You also need to consider driving traffic to your site.The best eCommerce sites are eye candy because of heavy investment in digital marketing.
If you don't have enough budget, you can still avail the perks of utilizing the true potential and proper use of SEO, not as instantaneous as digital marketing. Still, it has significant potential in the long run. Subscribe to digital marketing newsletters, join community pages, follow relevant threads and listen to digital marketing podcasts to stay updated about updates, trends, and recent breakthroughs in the digital marketing industry.
Your eCommerce platform isn't the only thing you need to attract traffic to but the products you choose also need space in your marketing budget.You are here to sell products, not just drive traffic. You have to think outside the box and find hidden dimensions to expand your approach to selling products.
Despite the way you choose to sell, the first step is to set up an email list. Place an option for free sign-up on your eCommerce site, run a social media campaign to attract subscribers, or arrange a giveaway session where the entry 'fee' is your customer's email address. Providing consumers with discount coupons and content via email helps keep your brand active in their minds, upsurge in sales, and promise credibility. Keep your emails interesting – ask for your customers' input often, including reviews. Evaluate your site operations and insights by looking at how and where traffic flows.
Are your product pages relevant to your persona? Are you losing potential customers? If you're driving traffic to your store but experiencing empty carts and low sales output, improve everything you think needs improvement in your sales channel by carefully optimizing each page and evaluating your product listings. Use Google Analytics to keep track of vital operations and insights. Some tools can help you observe and optimize every segment of the sales process.
Don't hesitate to use these tools and methodologies.Look for a partner and collaborate to boost your brand presence by using affiliate marketing options.You can also offer influencers and Instagrammers a gift sample of your product in exchange for reviews and marketing. It can bring you overwhelming traffic and boost sales.
End Words
Learning how to start an eCommerce business isn't that much easy as you think — but by carefully taking one step at a time in the right direction, as we've discussed, you can make the process more organized, take control of things and mitigate possible risks.
Additionally, there are numerous perks of starting an e-commerce business, including the much lower investment, small start, and feasibility to utilize 24 hours a day, seven days a week while offering services to all around the world if you're willing to ship that far.
It's also much easier and budget-friendly to expand operations if needed. Refrain from getting things done in a hustle. Choose a business model and your market niche carefully. It is more important than the development and operation phase.
Everything will start aligning automatically if you take the initial two steps seriously. There is, however, a specific roadmap to follow and investments to make if you want your eCommerce business to provide you with appealing ROI.
You should deal with your eCommerce site like any other business, follow the regulations and laws related to tax and registration, don't forget to obtain necessary permits as per your country or state's policy. Don't hesitate to invest in customer retention and communication. Last but not the least, don't forget about building a mobile-friendly platform as more than 60 percent of the purchases are being made through mobile devices, according to recent statistics.
Snowflake vs AWS Athena - Best Data Query Warehouse in 2022
Are you looking for the best data warehouse solution for your business or organization but confused about choosing the best option? Don't worry! We've got your back, as we have compared the two leading data warehouse giants, Snowflake vs AWS Athena, in our blog.Data warehouse solutions have seen tremendous popularity in the past few years thanks to their ability to get deep data insights. Big data and analytics have now become a driving force behind companies' progress.The volume of data generated every moment has increased significantly over time. As a result, cloud data warehouse technology has emerged as a solution and is extremely efficient at handling data analysis tasks.[lwptoc skipHeadingLevel="h1,h4,h5,h6"]Speaking of data warehouse solutions, both Snowflake and AWS Athena have proven themselves as the front runners and provide profound big data analysis. So we decided to dig deep into their comparison to help you make the right decision. Keep reading to unlock the better choice for your business.
Understanding Snowflake and AWS Athena
Before we had cloud data warehouse solutions, the idea of setting up a data warehouse meant hefty amounts, labor, and complicated processes. However, warehouse solutions like Snowflake and AWS Athena have simplified the process and are providing affordable solutions.
Related: Snowflake vs Redshift – Complete Comparison Guide
We need to define AWS Athena and Snowflake before we compare them so that our readers who are not acquainted with them can get a clear idea about them. If you already know about both leading data warehouse solutions, then you may skip this heading and move on to the next one.
What is Snowflake?
Snowflake is a cloud data warehouse solution that is made on either the AWS or Azure cloud infrastructure. It is perfect for businesses and organizations that don't look forward to hiring special resources to handle their server's maintenance, support, and installation.Since Snowflake is a cloud-based warehouse solution, it does not involve any hardware or software, which means you don't have to worry about the management, installation, configuration, selection, or any such things.The revolutionary architecture and the trailblazing data sharing abilities are the reason why Snowflake is a renowned name in the market.As a result of the Snowflake architecture's ability to expand storage and carry the computation process separately, you have the complete freedom to pay for storage only when they need it. Additionally, the sharing feature makes it quite handy for companies to share authorized and secure data instantly.
What is AWS Athena?
Using Athena is quite simple as you have to define the schema after pointing to your data in Amazon Simple Storage Service and proceed with querying via SQL. It is a fast service and delivers output within a couple of seconds.You don't have to worry about scaling with Athena, as it is serverless and is designed to scale automatically. By being serverless, Athena cuts down the work required to manage the infrastructure.Thanks to Athena's auto-scaling feature, you don't have to worry about running queries concurrently when working with big data sets or complicated queries.AWS Athena is a cloud storage service that is used by data analysts worldwide to manage massive amounts of data. This interactive query service uses SQL to analyze data that is stored in Amazon S3.Athena eliminates the need for time-consuming ETL operations by conducting the data analysis. This simplifies large-scale dataset analysis for anybody with a basic understanding of SQL.
Snowflake vs AWS Athena: Comparison
Now that you know about Snowflake and AWS Athena, we can juxtapose both cloud warehouse solutions to conclude the better solution. We will compare Snowflake vs AWS Athena in six different departments and then choose our final winner with the most wins in all departments.
Performance
The most important aspect while choosing a data warehouse solution is the performance. Noone fancies a system that lacks performance. Thankfully, in terms of performance, both Snowflake and Athena are strong contenders.Snowflake allows you to scale a virtual warehouse or add more warehouse instances and lets you operate as many sessions as you want simultaneously.Athena also permits an infinite number of processes to run at the same time. However, in reality, AWS only allows up to 20 SQL queries to run in parallel.AWS Athena is a serverless service that scales, manages, and builds data sets without requiring extra infrastructure. However, Athena depends a lot on how the data is structured in S3.Even though Snowflake isn't serverless, you won't have to worry about setting up storage and computing since Snowflake works on them independently and handles them using the Snowflake Data Cloud.All in all, Athena is a bit conflicting in performance, while Snowflake is a lot more consistent. AWS Athena helps with data discovery but isn't an excellent query engine.Winner: Snowflake
Management
Maintenance costs should be calculated in the deciding phase of selecting a data warehouse solution. In addition to price, the effort should also be counted in, as you should always prioritize automated systems that do not require much manual intervention.Both Athena and Snowflake are self-managed services and don't require any human help for their optimization and maintenance.You don't have to worry about maintaining or tuning any variables with Snowflake and don't have to deal with backups as well since everything is automated.Like Snowflake, Athena looks after the upgrades, backups, etc. Athena even goes a step further by generating schemas for you automatically, thanks to AWS Glue. Although Snowflake does not offer any such feature, you can use third-party tools to get somewhat similar functionality on Snowflake as well.Winner: Athena
Caching
Snowflake uses SSDs on the compute nodes to store cached copies of the data you query. If your virtual warehouse is suspended, the data will remain temporarily on the compute nodes. When you subsequently start your virtual warehouse, it's possible that the cached data will still be there.Sadly, Athena does not have a data caching feature, which is a big turn-off. Athena, however, supports result caching. Result caching works as the result of each query is physically stored, and subsequent execution of the identical query is fulfilled using the cached version of the result.Snowflake also supports result caching and does all processes automatically. While, on the other hand, Athena provides a manual solution which is quite underwhelming.Athena stores the query results in S3. To reuse the results from one query in another, you will have to create DDL on the underlying table and direct the query as well. All this hard work makes Athena's version of result caching quite ineffective.Winner: Snowflake
Scalability
Snowflake's storage and computation architecture are separated, resulting in excellent scalability. The only method to acquire bigger nodes is through larger clusters. Thus it is ineffective at scaling for specific queries that need more nodes.Snowflake requires rewriting whole micro-partitions for each write, it is best suited for batch writes. In addition, Snowflake sends whole micro-partitions across the network, causing a barrier as the system grows in size.Since Athena works as a shared platform and is used by multiple accounts, the performance of each account is restricted to prevent data loss. Athena has a default concurrent user limit of 20. As far as scalability goes, Snowflake is a better option than Athena.Winner: Snowflake
Security
You cannot afford to compromise on security when picking up the right cloud data warehouse solution for your business. Snowflake and Athena offer the essential required features like robust AES 256-bit encryption, authentication, and authorization.Snowflake can secure data at the column and row level, thanks to its built-in security feature.Snowflake allows you to use its multi-tenant environment, limit data to your users, and only let specific users access the confidential data. Snowflake's data sharing feature also allows you to use secure views. Unlike Snowflake, Athena does not offer such features but enables the user to secure their objects.Athena's data security utilizes the AWS shared responsibility framework. AWS is in charge of safeguarding the global infrastructure on which the AWS Cloud runs. If your material is hosted on their infrastructure, they allow you to be in charge of keeping that content under your control.Winner: Athena
Pricing
The last domain for the comparison of Snowflake vs. Athena is the pricing plans. When looking for a perfect data warehouse solution, you cannot ignore the pricing factor. In fact, some people even start looking for solutions based on this very factor.Considering the compute pricing, Snowflake charges somewhere more than around $2 to $4 per node. Keep in mind that faster analytics need bigger clusters that may cost you from $15 to $30 per hour or even more. On the other hand, Athena does not charge you a dime for computing. Isn't it cool?Snowflake charges you from $23 to $40 per TB on a monthly basis on-demand/upfront and does not charge you on transferred data. Meanwhile, Athena charges you $5 per TB scanned (10MB per query)Check out the detailed pricing plans of both cloud data warehouse solutions on their official website:
Know your budget, and then explore the options accordingly. In terms of pricing, Athena is much cheaper than Snowflake. It comes at the cost of limited benefits.If your business or organization has a solid budget and prioritizes performance over anything, then you should go with Athena. Conversely, if you are on a tight budget and are ready to compromise on the functionalities and features, then Athena is your friend.Winner: Athena
Final Decision: Snowflake vs AWS Athena?
We have compared Snowflake and AWS Athena on six different departments and concluded a separate winner for each department. As per our research, experience, Snowflake has emerged victorious and has the edge over AWS Athena on most factors.After reading the blog, you must have chosen your winner as well. We'd love to know your choice as well, so leave the name of your preferred cloud warehousing solution in the comment below.
10 Must-Have Shopify Plugins for Better Conversions
Shopify is the 3rd biggest eCommerce platform as more than 800,000 stores are powered by Shopify.
Apart from its user-friendly interface and state-of-the-art backend services, one of the primary reasons people prefer to choose this platform is its utility tools, plugins, and extensions that allow users to have full control over the workflow and outlook of their store by adding personalized and highly customizable features.[lwptoc numeration="none" numerationSuffix="none" title="Contents" skipHeadingLevel="h1,h4,h5,h6"]The Shopify plugins are known for boosting customer engagement, driving sales, and generating more profit margins.
Related: How to Launch Your e-Commerce Store on Shopify
This blog post will discuss 10 of the best Shopify plugins to optimize your e-commerce experience and boost conversions.
Best Shopify Plugins for Better Conversions
- Free Shipping Bar by Hextom
- Returnly (for Returns & Exchanges)
- Wishlist Plus
- One Click Social Login
- Upsell and Cross-Sell Products
- PageSpeed Monitor
- Plugin SEO
- Free Trust Badge
- Social photos
- Swell Loyalty & Rewards
1. Free Shipping Bar by Hextom
Free shipping is one of the primary reasons that motivate online customers to buy from you. Statistically, around 90 percent of online customers prefer free shipping as the top reason to shop online.As an eCommerce business owner, bearing overwhelming shipping costs on your own is not profitable. It only works if you offer free shipping to customers who reach a certain threshold. Still, it comes amidst a lot of manual effort.But the Free Shipping Bar by Hextom deals with this issue for you on its own.This amazing plugin enables you to showcase your customizable bar and adjust notifications as customers add more products to the cart.
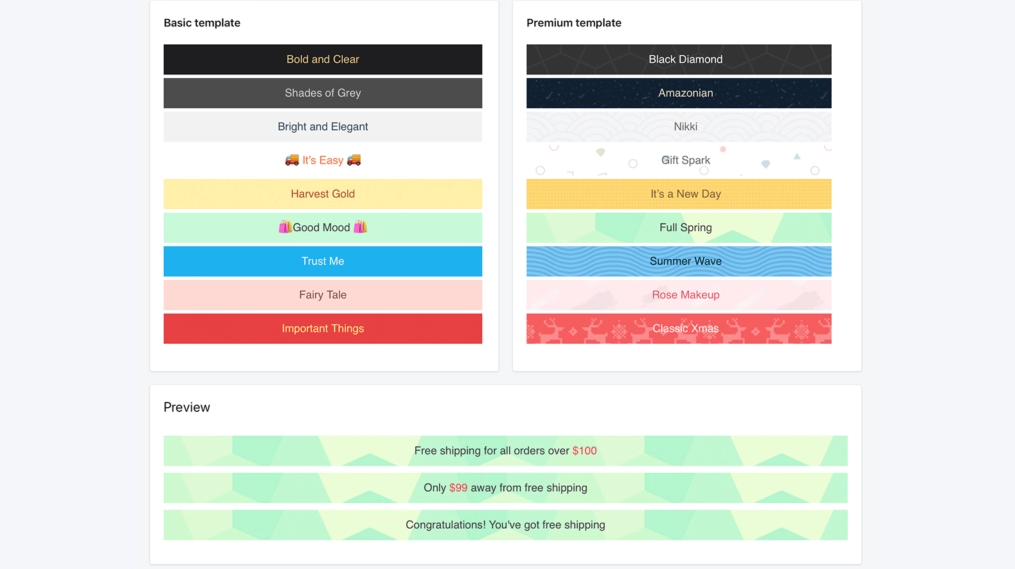
Moreover, it also offers integrated metrics to determine which product results in the most sales. Below is the screenshot representing how this plugin looks before anything has been added to the cart.
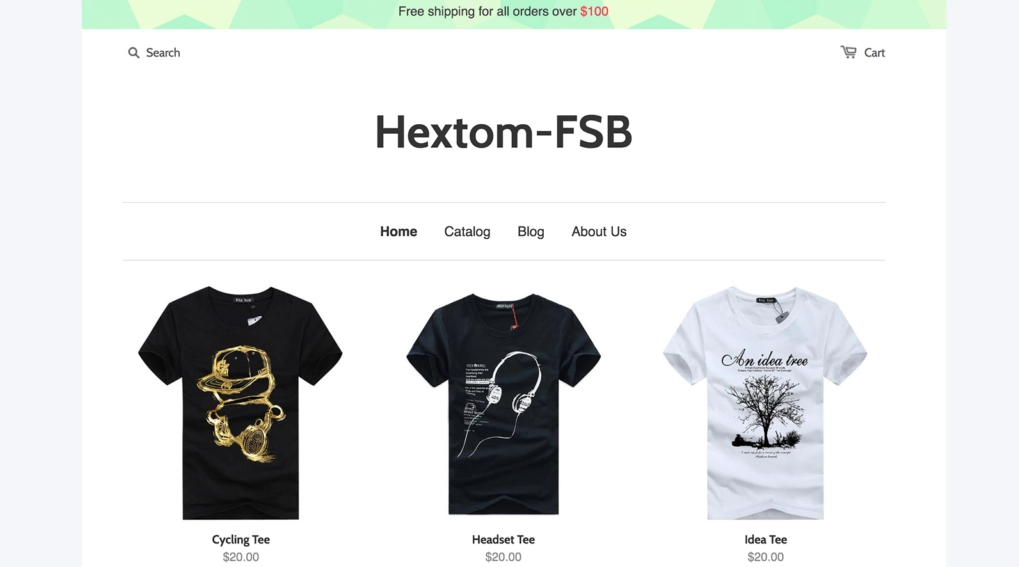
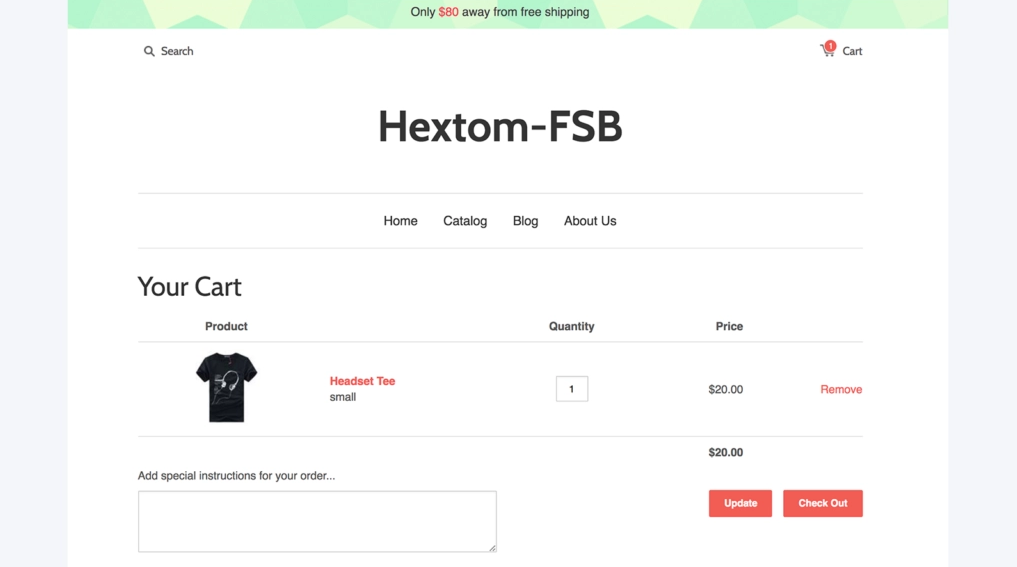

Here’s how it appears once a customer adds a product and moves one step closer to free shipping.And here’s how it looks once they’ve attained the certain threshold required for free shipping.It’s very simple to use and has a notable impact on your eCommerce business to boost sales.
2. Returnly: Returns & Exchanges
The only reason that puts the eCommerce business at a disadvantage compared to brick-and-mortar is the possibility of return and exchange.Around 30 to 40 percent of products purchased online end up being returned compared to only 8 to 9 returns’ percentage in the case of brick-and-mortar stores. Multiple factors drive this phenomenon, among which a few of the main reasons are the product appears different than expected or showcased in pictures, wearable products either outfit or underfit, and receiving a damaged product. As eCommerce owners have to deal with huge returns, acquiring a simple return process can sort out this overwhelming workload. More than 90 percent of shoppers prefer to buy again if they have an easy return policy and procedure. That’s where Returnly comes into the frame. It reduces all the plausible hurdles in the returns process. It allows customers to purchase another item using store credit before retrieving the first item run last to boost sales and ensure loyalty.
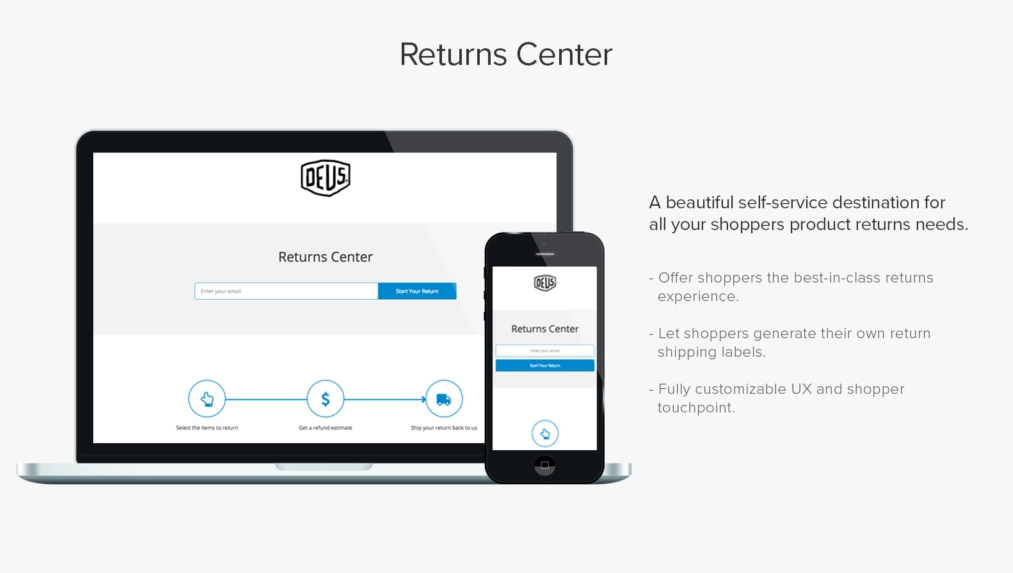
You can use it to set up a highly customized Returns Center and streamline your product returns process.
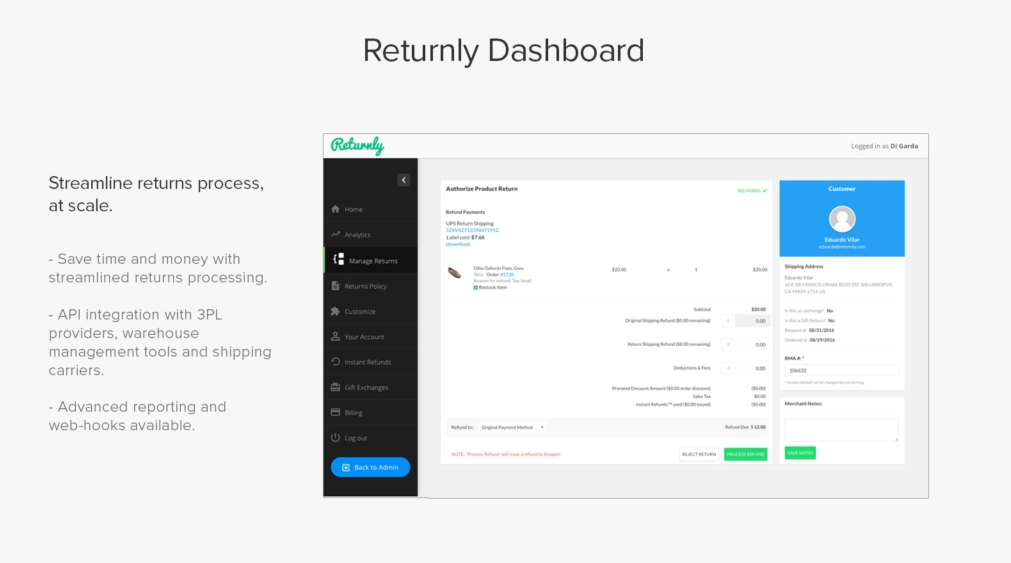
It also offers robust analytics to extract fruitful insights related to customer behavior, which can promise a substantial profit in the long run, last but not least. Hence,
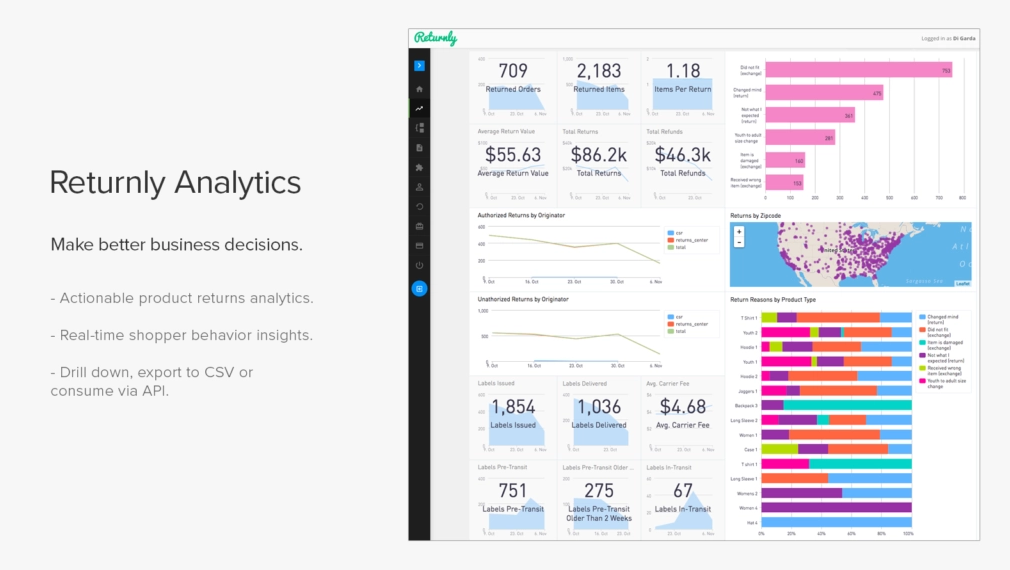
So if order returns are disturbing your routine workload and ruining your peace of mind, this is a plugin you must consider.
3. Wishlist Plus
Everyone loves to maintain wishlists, and creating one is a fun activity to keep a record of the products you’re interested in so that you can go back and purchase the ones you love the most later on.It allows your customers to bookmark their desired items and pick up where they left off whenever they want.Once they return to your eCommerce site, they can quickly jump to their bookmarked items and purchase them.
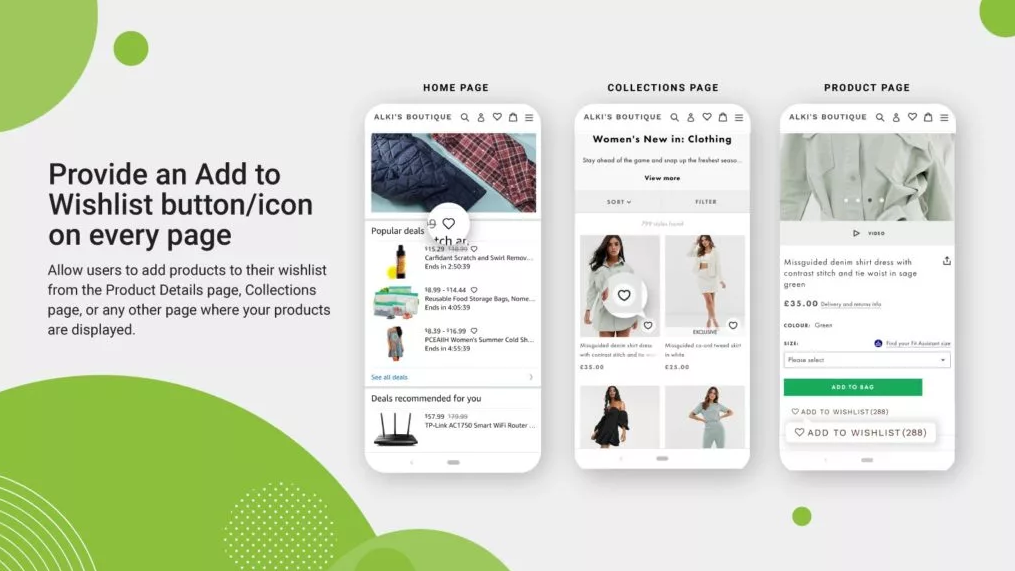
It’s very easy and interactive, and customers don’t need to sign in to leverage Wishlist Plus.Moreover, they can synchronize their wishlist across multiple gadgets for their feasibility of access from anywhere and anytime. The impact of integrating this plugin is notable as it boosts conversion rates by 20-30 percent and shoppers started buying 30-50 percent more on average. Last but not least, it reveals the number of times each product has been added to the wishlist, which serves as a value-added feature that can persuade customers to shop more.

4. One Click Social Login
Online customers abandon their cart because of extra charges like shipping, tax, and other fees, followed by creating an account.The need to sign-up is the reason why around 30-35 percent of customers abandon their shopping drive without making a purchase and leave your site never to come back.
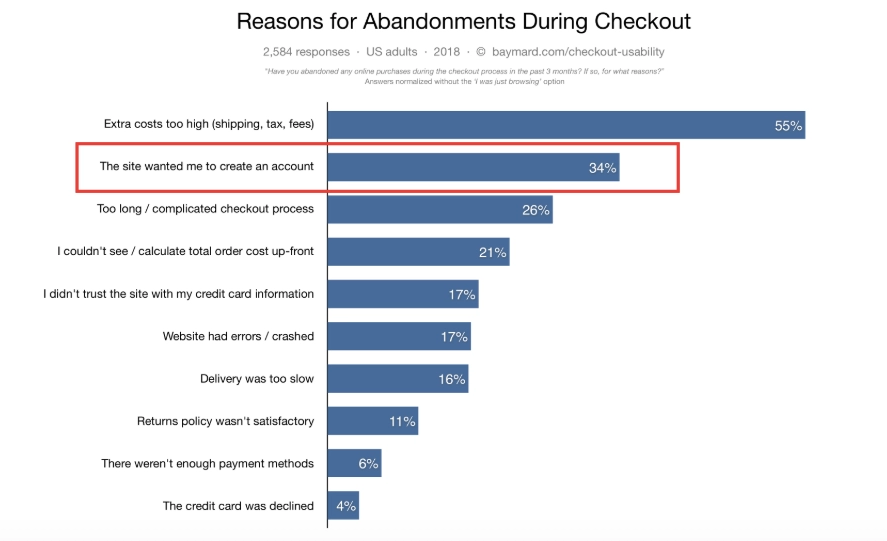
If customers are prompted to register themselves by making an account, it's demotivating them to continue shopping, especially if they plan to buy a single product. But the One-Click Social Login plugin is the ultimate solution to this problem.Apart from asking customers to create an account or sign-up by typing every detail, you can ask them to sign up using renowned social media networks like Facebook, Twitter, Google, Instagram, or LinkedIn.
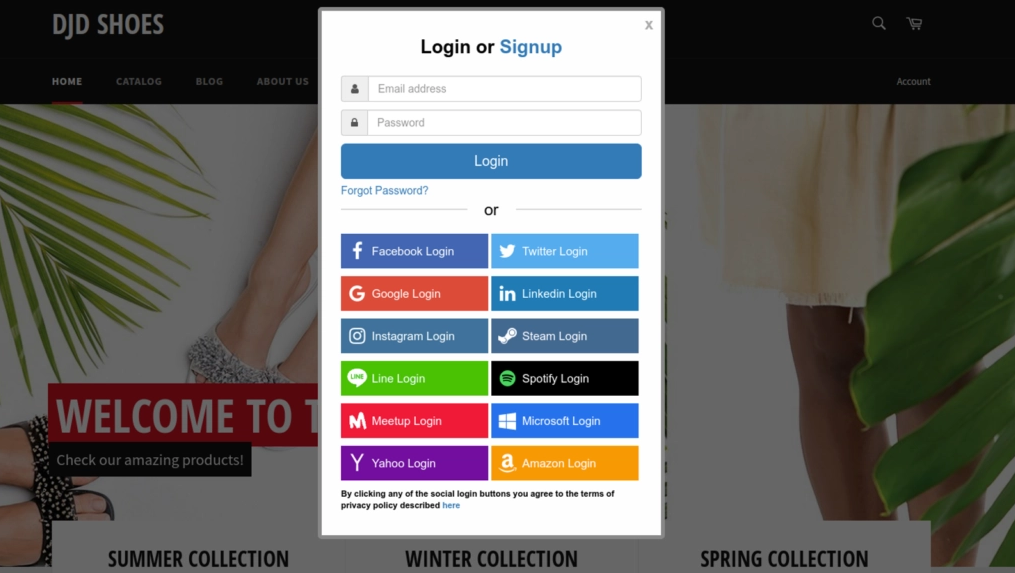
They handle all the fundamental input necessities and enable customers to sign up without trouble. You can even customize it as per your requirements and feasibility.

You can also track the record of how many users are signing up.
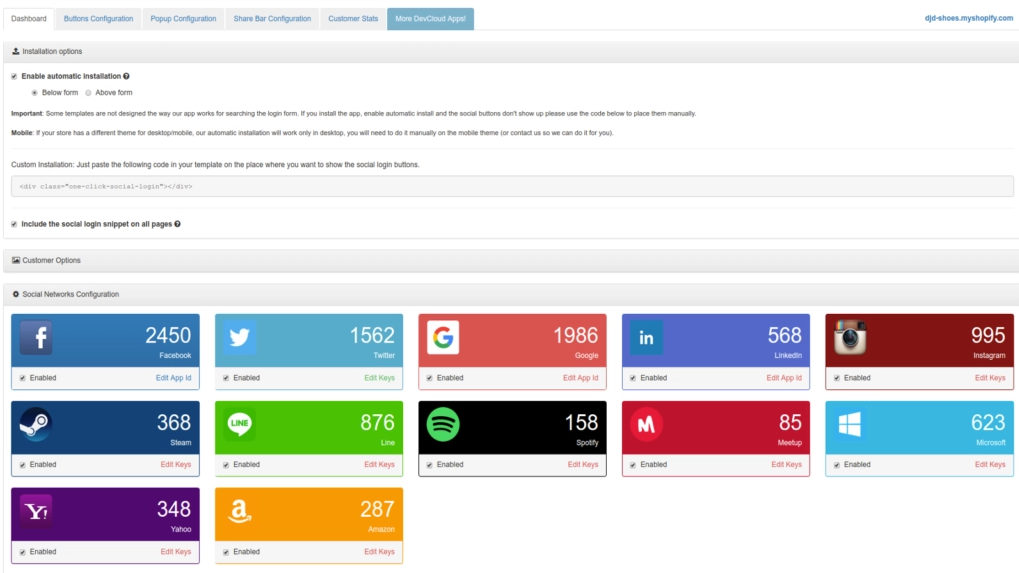
By allowing your shoppers to refrain from investing their time and effort in making accounts, it can cast a notable impact on your sales.
5. Upsell and Cross-Sell Products
One of the traditional business strategies is that it’s much more feasible and profitable to engage an existing customer than acquire a new one, and yes, it’s true. Increasing your average order value is one of the efficient ways to increase your profit margin. Quantitatively, You’re 60 to 70 percent likely to sell to an existing customer compared to that of 5 to 20 percent in case of selling to a new customer.So what’s holding you back from considering an option of upselling and cross-selling?This Shopify plugin by Exto is here to help you.You create relations between relevant products based on a simple mechanism of a rule-based system.
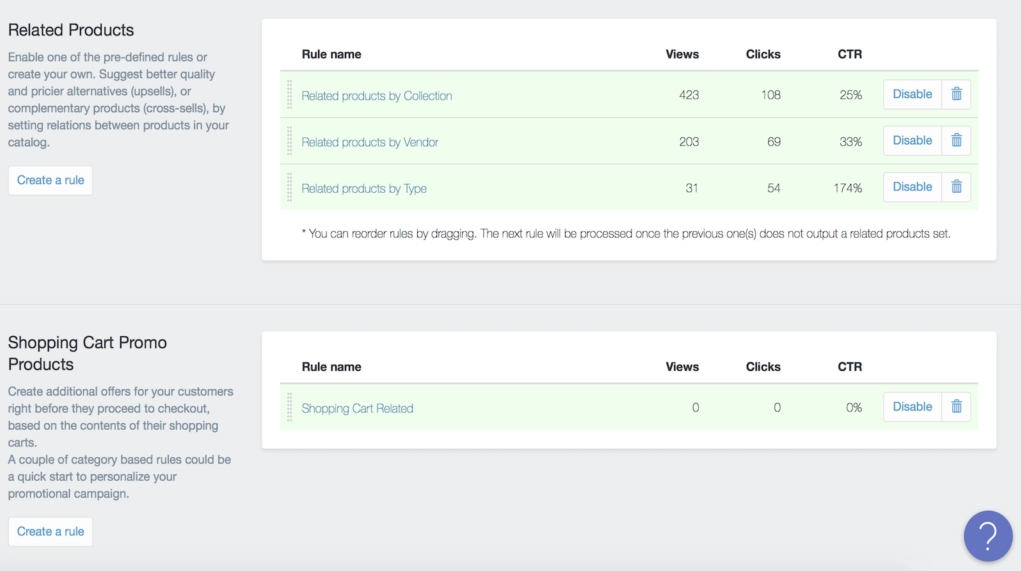
By doing so, customers will be automatically provided with recommended products based on their behavior.

And if they try to add a currently out-of-stock product, they’ll be recommended similar options that hold their interest. It also provides a Recently Viewed feed so shoppers can quickly return to the products by making a single click. It is undoubtedly one of the top-notch Shopify plugins that drive profit by maximizing average order value.
6. PageSpeed Monitor
Everyone knows how crucial it is for an eCommerce site to load quickly. If your page takes longer than 2-3 seconds, it will negatively impact customers’ motivation and leave them in masses. That's why it is highly recommended to use PageSpeed Monitor.It’s a very simple and easy-to-use extension that offers a track record of how fast your eCommerce site is loading and recommendations & tips to increase loading speed.PageSpeed performs by checking your Google PageSpeed Insights on an hourly basis and alerts you whenever any issue pops up so you can resolve it on time.
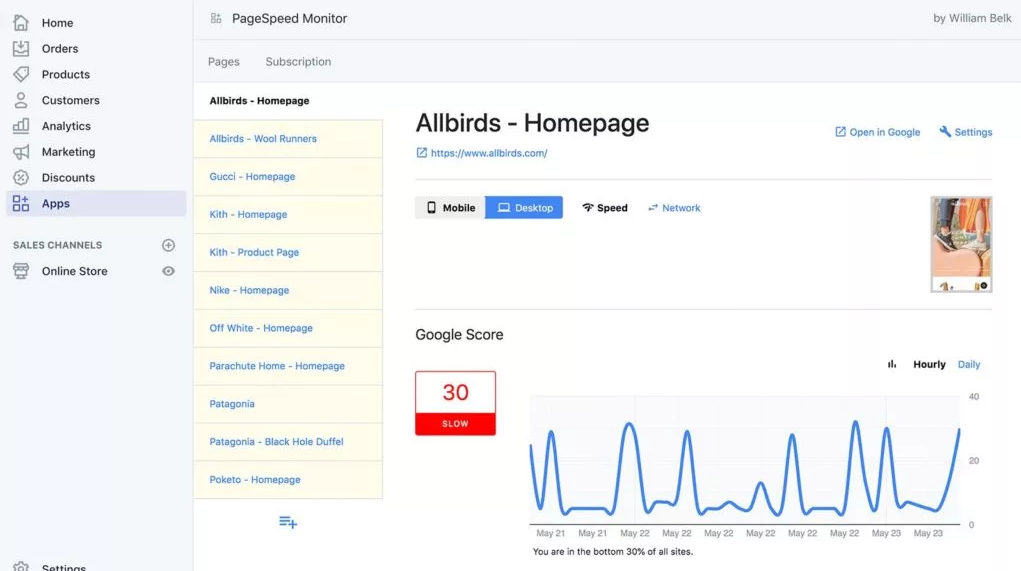
You can also evaluate your rivals' site loading performance, giving you a nice edge.
7. Plug In SEO
Another primary reason for eCommerce success is to secure your place in the top search results in all search engines. Seventy-five percent of online surfers don’t even scroll past the first page of the search results, among which 35 percent of the clicks are made to the top three results. So your business needs to rank in the top position. One of the best Shopify extensions for helping with Search Engine Optimization (SEO) is Plugin SEO. It helps you to find simple ways to boost the ranking of your Shopify platform and eliminate any hurdles that may be pushing you back. Some notable pieces of content it evaluates include:
- Meta titles;
- Headlines;
- Meta descriptions;
- Keywords; and
- Broken links

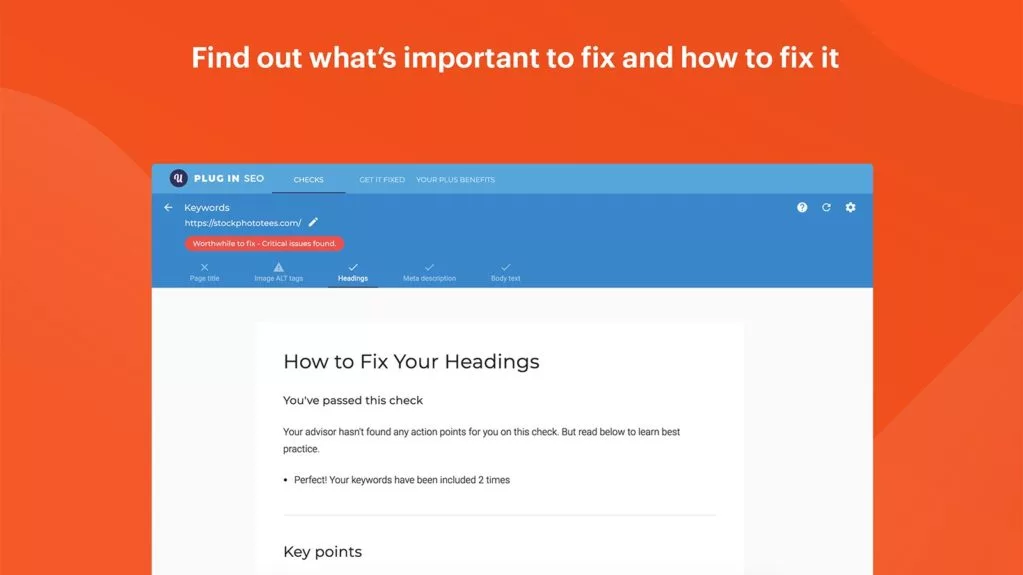
And if you have rich content on your website, it can help you make it SEO-friendly.
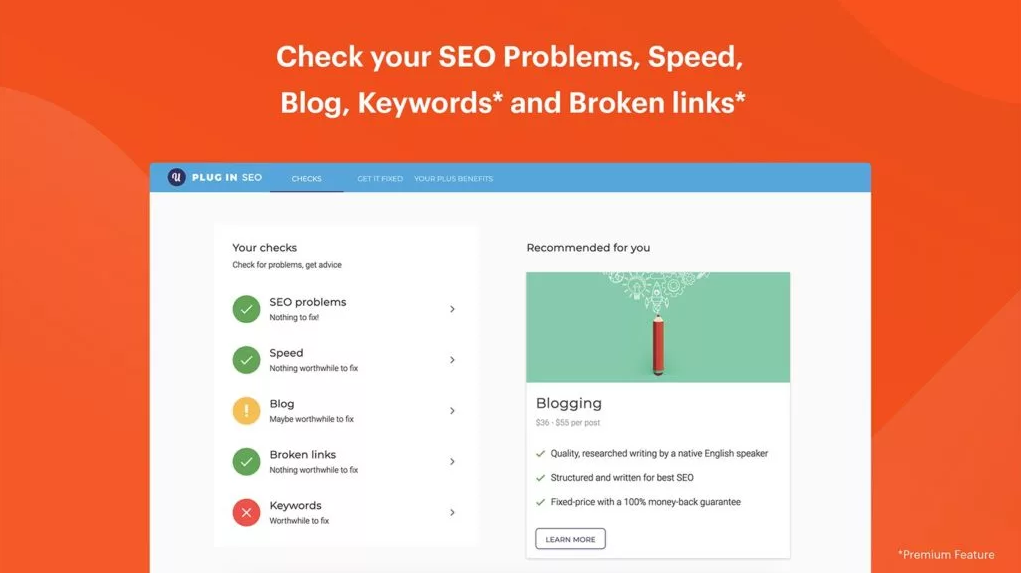
By doing so, you can attain the place among top search engine searches by using relevant keywords and notably boost your website traffic. And this tool does not require you to be an SEO expert. Plugin SEO handles all complex tasks for you and helps you develop knowledge about SEO over time.
8. Free Trust Badge
Another reason for cart abandonment is that customers do not trust the site with their credit or debit card information.

So many customers worry about sharing their sensitive credit and debit card information. That’s why e-commerce stores need to eliminate this owner-customer barrier and trust issue.One way to tackle this issue is to add trust badges on areas of primary focus, such as your product and checkout pages. Free Trust Badge extension should be your top choice. It provides you access to a library with a multitude of basic badges.

Moreover, it also offers a premium version with full-colored badges from renowned companies.
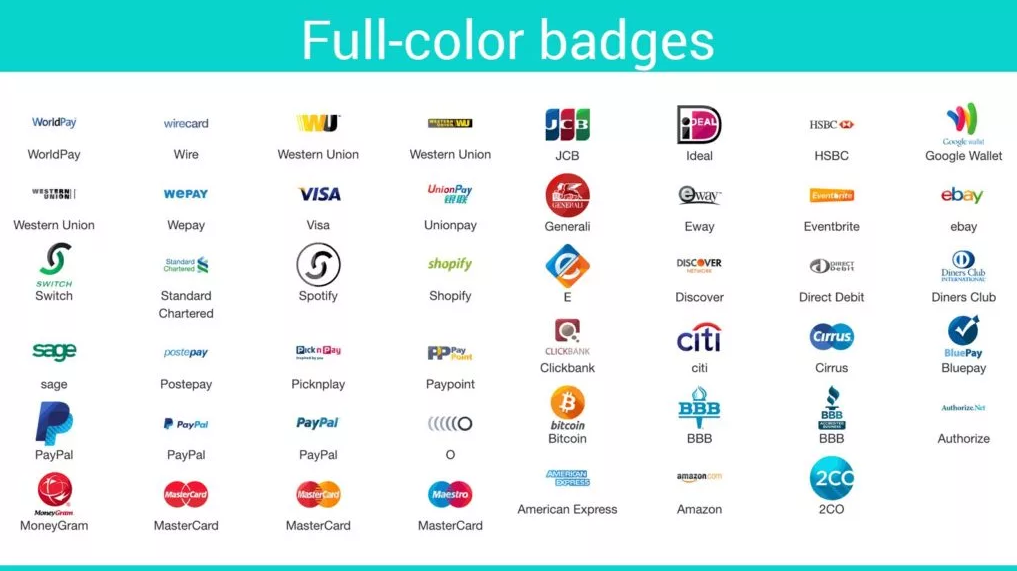
It sets up very quickly and easily as it takes only a couple of minutes to deploy, and everything is highly customizable. Hence, trust badges adjust effortlessly with your brand theme.Consider an example below.

And if you choose the premium variant, you can enjoy the perks of having more than 250 premium badges with various designs to opt from.
9. Socialphotos
If you have a strong social media presence with stills of your customers using your products, you can use Socialphotos to showcase those pictures to online customers.Socialphotos gathers some of your top customer product photos from well-known. Hence, purchasing social media sites such as Instagram and Facebook adds an album on your eCommerce site using a widget tool.Consider an example below.


It’s a perfect way to unleash the magic of visuals and social media posts, win new customers, and ensure trust by making them feel comfortable purchasing from your eCommerce platform. The widget is highly customizable, and you can select the photos you want to showcase on your eCommerce site.
10. Swell Loyalty & Rewards
Most online consumers love loyalty and rewards campaigns. Statistically, around 55 to 60 percent of online consumers around the globe regard earning rewards and loyalty points as one of the most interesting aspects of the eCommerce experience.”You can also capitalize on your e-commerce business using such tactics as an effective way to motivate first-time and consistent shoppers. Swell Loyalty & Rewards is one of the renowned Shopify plugins to serve this purpose. Below are some of the scenarios in which you can reward your customers:
- Referring friends to your store
- Ordering Frequently
- Leaving positive reviews
- Reading, watching, or sharing your valuable content
- Connect with your brand on social media platforms
For instance, you can use this tool to promote your reward campaign and let shoppers know how many points they’ll earn for completing a certain course.

And shoppers will be able to check their reward points easily.

Like previous tools, this extension is highly customizable, and you can choose from a variety of themes and color choices to match your branding.
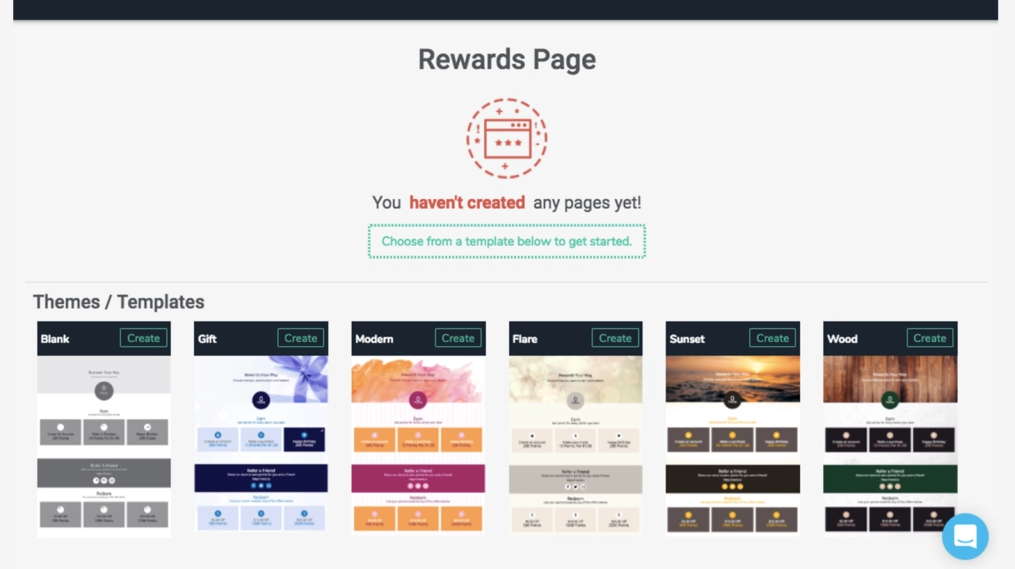
It also showcases detailed metrics to let you know your campaign's overall impact and outcomes.
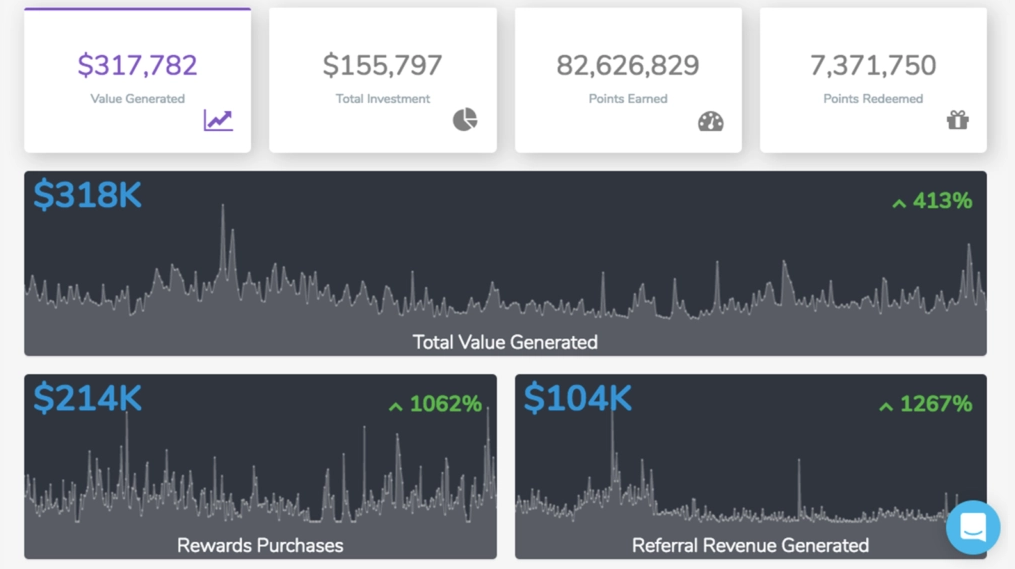
Conclusion
As an eCommerce business owner, there are many ways you can optimize your shop and boost conversions.Around 3000 plus Shopify plugins & extensions exist, and choosing a few out of all these is an overwhelming task.The aforementioned Shopify plugins can reform every aspect of your store and boost your productivity as well as profit.No matter if you’re gearing up to upsell and cross-sell products, boost average order value or establish customer integrity, you can achieve your goals using these Shopify plugins. Last but not least, these tools and utilities are highly customizable so that you can use them as per your feasibility and need.
How to Choose a Firm for Data Science Consulting
In today’s time, data science and artificial intelligence are trending topics in the business arena. An expert talking about the capabilities of predictive analytics for business on a morning TV show is far from unusual. Articles covering AI or data science in Facebook and LinkedIn appear regularly, if not daily.[lwptoc numeration="none" skipHeadingLevel="h1,h4,h5,h6"]Due to a surfeit of information about AI and big data on the Internet, companies can assume that data analysis is the solution for most of their data-related issues.But keep in mind, before planning to use data science or AI for your business, find out whether it’s the technology you need. You can answer this initial question by isolating and specifying your problem.This blog post will discuss what factors to consider and steps to take to team up with competent data science or AI vendor.
Reasons to Hire a Consulting Company
There are lots of reasons to hire a consulting company (outsider), and some common ones are:
- Internal staffs lack/with a gap in the skills and knowledge gap with a product or technology to build up their data science solutions.
- Internal conflicts and politics between different departments are the barriers to carrying data science implementations.
- It is too risky for the project to be 100% handled internally. It is expected to have some valuable advice provided by the consultants.
- Staff within the organization should have the best knowledge in the business, but they may not start their first analytics without proper skills and tools.
Steps involved In Choosing a Firm for Data Science Consulting
1. Match your problem with possible solutions
Data science and AI solutions generally entail gaining valuable insights using available data. Some companies approach data science vendors to build products whose key functionality is centered around machine learning. For instance, this may be an application that transforms speech into text. Other organizations may want to develop a custom analytical and visualization platform to control their operations and make strategic decisions based on the insights.In the broadest sense, you can apply data science to gain insights about your business and improve your operational efficiency, or you may want to deliver AI-based applications to your end customers. In the former case, the end-consumer would be your company; in the latter – your customers.
Customer-facing apps and fraud detection
Customer-facing applications powered by machine learning algorithms solve your customers’ problems. People may use these products for their daily activities or to do work tasks faster and easier.These are examples of customer-facing solutions that need data science engagement:
- Virtual text and voice assistants (e.g., Mezi travel assistant or Expedia chatbot)
- Recommendation engines for eCommerce and over-the-top media service providers (e.g., Amazon and Netflix recommendation systems)
- Price prediction engines (e.g., Fareboom or Kayak fare predictions)
- Apps for conversion of speech into text (e.g., the IBM Watson Speech to Text or Voice Assistant by QuanticApps)
- Sound recognition and analysis applications (e.g., Do I Grind healthcare app)
- Image editing applications (e.g., Prisma app)
- Image recognition apps and features (e.g., credit card recognition with the Uber app)
- Real-time visual and voice translators (e.g., Google Translate app or iTranslate Voice)
- Document classification apps (e.g., Knowmail)
There’s also a group of fraud detection products that employ both data science and traditional programming techniques to build.
Business analytics: business intelligence and statistical analytics
Business analytics (BA) explores data through statistical and operations analysis. BA aims to monitor business processes and use insights from data for making informed decisions.Business analytics techniques can be divided into business intelligence and statistical analysis. Companies with business intelligence (BI) expertise analyze and report historical data. Insights into past events allow companies to make strategic decisions regarding current operations and development options. Statistical analytics (SA) allows for digging deeper while exploring a problem. For instance, you can find out why customers prefer booking from OTAs rather than from your hotel site this week or whether or not a particular user buys products after reading an email about current deals.Business analytics can be used for:
- Data management
- Dashboards and scorecards development
- Big data analysis
- Price, sales, or demand forecasting
- Client analysis
- Sentiment analysis on social media
- Risk analysis
- Market and customer segmentation
- Customer lifetime value prediction
- Upsell opportunity analysis, etc.
Business analytics allows for solving problems of various complexity, from simple reporting to advice on measures for risk mitigation or operations improvement. And to address these problems, you may use different types of analytics. There are four analytics types:
- Descriptive
- Diagnostic
- Predictive
- Prescriptive
Let’s suppose you own a car dealership. According to analysis, sales of new models are lower compared to the same period last year. This is an example of descriptive analytics, and its purpose is to provide a performance overview. Descriptive analytics allows for answering the question, what happened?Please note that you don’t need data scientists for most cases of descriptive analysis. You can do it by yourself with BI tools.You can try to dig deeper and understand why people are purchasing fewer cars in your stores. One of the ways is to measure sales records against external data like industry trends or market data. In other words, to perform diagnostic analytics. This analytics type allows for figuring out why something happened.If you want to estimate how many automobiles will be purchased during the next three months or whether they will be purchased or not, you need predictive analytics. This more complex analytics type uses descriptive and diagnostic analytics insights to forecast the probability of future events and outcomes. In addition, you can evaluate a moment at which an event might happen. So, predictive analytics allows for determining what is likely to happen next to optimize ongoing operations.Predictive analytics usually requires machine learning that you can read more about in our article.If you want to know what measures to take to increase sales, embark on prescriptive analytics. This analytics type entails actions that include evaluation of insights about past events, their cause, and various forecasts on upcoming events. Entrepreneurs get recommendations on actions needed to eliminate future issues or benefit from emerging trends with prescriptive analytics. Prescriptive analytics uses machine learning as well.Consider every event in your business, be it a transaction, a website visit, or staffing changes, was filmed and became a part of an episode in a long series. Business analytics is the tool you can use to rewind the video, spot some facts, analyze them, and draw conclusions. But that’s not all you can do. BA allows you to understand the cause of events, predict what can happen next, and make an optimal plan to ensure that yours is a success story only.
2. Consider off-the-shelf products
Make sure you’ve analyzed off-the-shelf solutions before you start looking for a team. Such websites as KDnuggets and PCMag have listings of analytics solutions and SaaS companies. Some of them (e.g., Capterra) even specialize in software research. We’ll talk more about sources you can check out to find vendors or products below. Also, if you look for customer insights and use common CRM software to collect and maintain customer interaction data, find out whether your vendor provides modules that would address your problem.However, off-the-shelf solutions may not support the functionality you need. Let’s assume, for example, to adjust the marketing campaign; you need to analyze reviews that customers share on social media. As your data analytics platform doesn’t support this feature, you need the one that’s customized to analyze feedback in your domain. These are sentences, words, and word combinations customers may frequently use when discussing your services. For instance, reservation, room service, cancellation, room key, or breakfast included if you run a hotel business. In this case, you may hire a team of data science specialists to build tailor-made software.
3. Study company listings
Now it’s time to find a consultant who will develop a solution specifically for you. We’ve picked several websites that may help you fill the workforce gap with qualified data scientists.
KDnuggets: listings of DS consulting, product companies, and analytics solutions
Besides news and articles, KDnuggets has publications that may be useful for businesses that want to leverage DS. The website lists data science consulting companies with brief descriptions of their expertise and office location. You can also find listings with product companies and analytics solutions clustered by technology and industries on the same page.

Experfy: a marketplace to find experts for big data, analytics, and BI projects
Experfy is a Harvard-based data science consulting marketplace where clients from various industries can partner with experts for short- and long-term projects. The platform allows clients to hire external teams or augment existing ones. While most experts are freelancers, clients can negotiate with them to work on-site if needed.Users post their projects according to their practice area or technology, specifying goals and requirements. Experfy then solicits bids on clients’ behalf, narrows down potential contractors, and clients start getting expert proposals. Collaboration with freelance specialists is done in the Experfy Project Room – a private work environment. The platform deals with contacts and charges a 20 percent fee on each transaction.

This is how Experfy works. Source: Experfy
Clutch: verified reviews to make data-driven hiring decisions
Clutch is an independent B2B research company based in Washington DC. Its goal is to assist businesses in making informed hiring decisions and allow service providers to advertise what they do. Clutch surveys clients about their collaboration experience with companies registered on the website. Reviews are located on a company profile and include some information about the commentator and the project and a feedback summary. A full review form is also available. Clients score providers on schedule, quality, cost, and willingness to refer, helping potential clients know the overall service quality.Clutch has a list of the best big data analytics companies. The list includes more than 600 companies and constantly updates. You can filter providers by Clutch rank, the number of reviews, company name, and review rating.
4. Look at the vendor portfolio: case studies and references
Once you have a vendor shortlist, start learning about the expertise of each of the potential consultants. Industries for which a company builds solutions must be the first thing to look at when researching its website. A data science consultancy company with domain knowledge goes beyond delivering a solution: Specialists can consult you regarding product development and usually spend less time studying your problem.Case studies. Each company strives to prove it has sufficient expertise in its field. However, nicely written testimonials and client logos on the main site page don’t add as much credibility to a company as related case studies. A case study allows for learning about a client’s background, the technology and methods a consultancy company applied to solve a specific problem, and of course, the outcomes. The level of analytics used in case studies is another source of guidance for a consultant search and selection.
Bayer Case Study – Digital transformation to enhance Sales Effectiveness
Besides reading case studies, consider contacting previous clients or visiting their websites to evaluate a vendor’s competency. You can ask companies from your shortlist to provide client contacts for references.Other marketing materials. News, press releases, and blog articles can tell much about a specialists’ proficiency, a company’s business strategy, and reputation. What events did a company participate in or hold? How often have media or other industry players mentioned this company in their publications, and in what context? Also, check corporate pages on social media to get a fuller picture of a potential partner’s expertise.
5. Interview a data science consulting firm: evaluation phase
Finally, you have some companies on the list you want to contact. That means that the time has come for the essential part of the consultant search journey – an evaluation phase. Ideally, this phase is all about a meaningful dialog between a consultant and a potential client.
Problem exploration
First things first, data science experts must define whether it’s feasible to solve your problem with data science or AI. You must be ready to describe your issue in detail and provide experts with operational data. The quality and amount of data are also crucial because an algorithm’s performance depends directly on them.49.4 percent of data scientists who took part in the 2017 State of Data Science and Machine Learning survey by Kaggle called dirty data one of the pain points they face at work. Lack of a clear question to answer (30.4 percent) and data either unavailable or difficult to access (30.2 percent) are other difficulties that experts wished they didn’t know about.
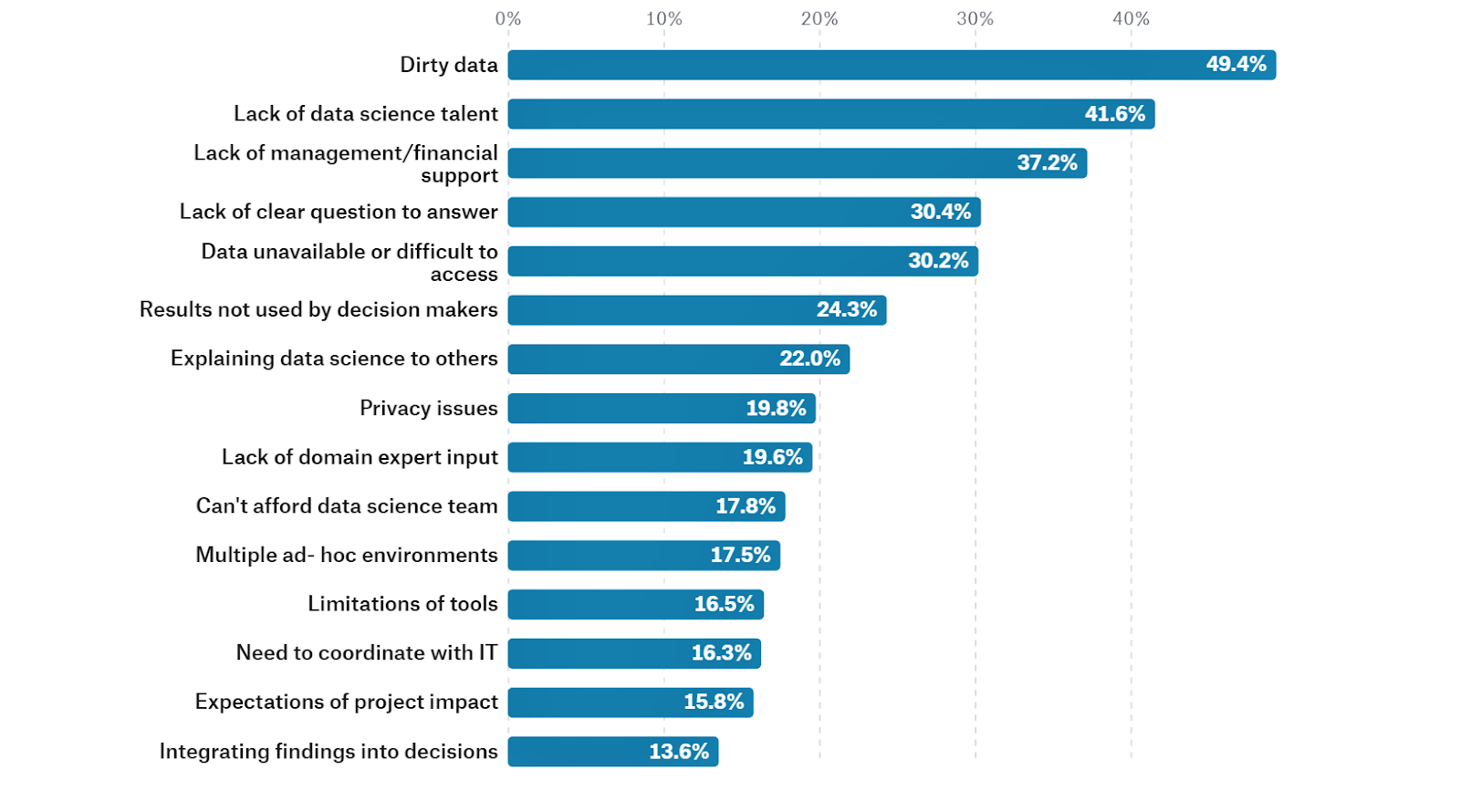
The top 15 answers about difficulties faced at work from data scientists. Source: Kaggle
Scope of work definition and preliminary results
A reliable consultant always takes time to verify whether a client has enough high-quality data before describing the scope of work and negotiating contract terms. Experts generally study a problem, scientific research articles, feed client data to algorithms that can be potentially used for a project, and present preliminary results – the estimated accuracy of an algorithm for a solution.For instance, Fareboom CEO Marko Cadez approached our data science team intending to upgrade. You must understand the existing travel booking engine with a fare-price prediction feature. Fareboom is an airline ticket search and booking site where customers can find the cheapest travel deals. Our team researched nearly 1 billion stored fares (which took about six months) before making assumptions regarding prediction confidence. Eventually, when tested on real data, the team presented models with 80 percent prediction accuracy. It’s worth noting that not every data science-related project takes so long in the initial stages. But you must be ready for long-term cooperation as DS projects require time.
Return on investment (ROI) estimation
Finding a solution to a challenge isn’t enough. The investment in the solution must pay off within a specific amount of time. So, one of the key tasks for a vendor team is to calculate the difference between a solution cost and return on investment.For example, your company loses a significant amount of money due to fraud. The simplest way to eliminate nearly 60 percent of suspicious traffic is to buy a list of IPs from which fraudulent activity was detected. An off-the-shelf software would block another part of potentially fraudulent transactions.But if you must achieve 95-100 percent fraud detection accuracy (which isn’t possible with most commercial solutions), then hiring a data science team to develop a model is reasonable. The higher the detection accuracy desired, the more significant the investment required. If you spend at least $250,000 on ML solutions every year, allocating $50,000 on a custom solution is a grounded decision. Medium and small businesses must think twice before developing a complex solution that takes more than a year to pay off. It’s essential to evaluate whether a sophisticated ML solution works well for an organization at its current development phase.Consider hiring data science consultant teams that honestly evaluate solution options, their cost, and the value of these solutions in terms of ROI.
Must ask Questions Before You Settle on a Data Science Consulting Firm
Here is a complete list of a few questions that you should necessarily ask or deliberate upon before selecting a Data Science Consultant.
A). What is his certification?
Accreditation or certification in any data science domain is the easiest way to find out that your expert has the required experience to cope with your execution work. It is also a remarkable technique to guarantee that the individual is up to date with the most recent Data Science changes.
B). What are his previous experiences?
Lookup for the past projects they have dealt with and see the correlation between those projects and the ones you want the company to deal with for you. Lookup for their client’s feedback, which shouldn’t be an issue as you can easily find it on their website, blogs, or LinkedIn feeds.
C). What is the implementation methodology that he follows?
When you plan to get the best Data Science Consultant, you must understand your accomplice’s usage approach. Agile-based incremental conveyance is the finest practice. It signifies stages and cycles, which help ensure that your undertaking keeps up with time and remains within the stipulated budget.
D). Does he provide any after-services support facilities?
Before taking Data Science Consultant on board, discuss their post-project services to keep up with your business progress. It’s best to work with an accomplice who keeps a sharp focus on recent Data Science updates so that your company can stay on the incremental curve.
E). What is his pricing technique?
Pricing is one entity you need to settle in the very first instance. Every company has its way of functioning, and you need to ensure that it meets your requirement. Always ensure that you are clear on the mode of payments, time of costs, the pattern of payments, etc. Discuss any extra fees and every minute detail mentioned in the work order
F). What is his Geographical Location?
Location plays a significant role while selecting your Data Science Consultant. You would not want a consultant sitting miles away from your location primarily because of two reasons- it causes timing issues due to the different time zones of the world, and secondly, works culture differs from place to place.
End Words
The abundance of data science and AI consultants won’t confuse you if you have a clear project goal and at least a basic understanding of what kind of expertise you need to achieve it. At the same time, a vendor must research your problem to determine whether it can be solved utilizing DS or AI. If it seems appropriate to leverage these technologies, a consultancy must provide you with a scope of work, present preliminary results, and evaluate project cost and return on investment.These are additional factors to consider before making a final choice:
- Data science team training. Find out whether a vendor provides knowledge sharing if you plan to form a data science team in the future.
- Other expertise. A company that provides related services besides modeling can help you define a product vision.
- Cooperation approach. Discuss partnership details with a potential consultant. For instance, teams following the Lean project management framework will require you or your company representative to spend the entire project with the development team.
Top 5 ETL/ELT Tools for Snowflake Data Warehouse
The data transition to a data warehouse like Snowflake can be referred to as stockpiling thousands of books in a library. By doing so, big data, companies’ most precious asset, will be safety and security. Also, Snowflake can store data for real-time big data analytics to extract fruitful insights and intelligence that helps in the decision-making process and business growth.[lwptoc numeration="none" skipHeadingLevel="h1,h4,h5,h6"]
What is Snowflake?
Snowflake is a renowned and highly scalable cloud data warehouse introduced to sustain your business intelligence (BI) needs. It's available as Software-as-a-Service (SaaS) for data-driven experts who need a warehouse to make schedule-critical decisions that fuel business growth. Snowflake integrates and centralizes Big data in structured, semi-structured, JSON & XML formats from many data sources so you can analyze it all with transparency and accuracy. Analytics is comparatively easy, but on the other hand, boarding all of your data to Snowflake in the first place can be an overwhelming task.
Why ETL/ELT for Snowflake?
ETL/ELT corrects your data and gives it a much-needed makeover to make it ready for Snowflake and then ready for analytics. But why is ETL/ELT is so important? Most data warehouses and BI utilities can't handle the overwhelming mass of data from various sources. Some platforms have to standardize data formats and join up records which delay the analytical process. When you extract, transform, and load data, none of these problems tend to occur.The ETL/ELT process scrambles heavy amounts of data for Snowflake storage, combining structured and unstructured data from even the most complex and latent data sources making the overall process less time-consuming and hurdle-free.In this blog post, we will discuss 5 top of the line ETL/ELT extensions available in Snowflake. Let’s discuss this in detail.
Top ETL/ELT Tools for Snowflake in 2022
1) Xplenty
Rating:Average user rating: 4.4/5Benefits:
- Integrated Snowflake connector
- No code platform
- Simple drag-and-drop UI
- A variety of 200+ data sources that is more than any other on this list
- Free customer support for all users
- Easy data transformations methodologies
- Charges depend on the connector, not data volume, making this service cheaper.
- Support cloud platforms, databases, systems, apps, and data warehouses, including Amazon Web Services - AWS, Microsoft Azure, Redshift, Talend, Oracle, Microsoft, Tableau, and Salesforce.
Xplenty is an all-rounder ETL tool for Snowflake, providing an easy-to-use native Snowflake connector. Unlike the other tools on this list, Xplenty offers a no-code development environment, making it a perfect choice for teams of all types. There's free customer support for all users, more than 200 data sources, simple data transformations, a drag-and-drop UI, and favorable pricing depending upon the connector rather than a data volume.
2) Apache Airflow
Rating:Average user rating: 4.1/5Benefits:
- Extensive support using Slack
- Flexible pricing
Limitations:
- Data transition from sources via plugins
- Support only Python language
Apache Airflow is an open-source utility that offers ETL modeling for Snowflake. It's one of the most renowned ETL utilities available in the market. Unlike other data platforms in this list, Airflow moves data from sources via plugins with templates based on Python. If you don't know Python programming, it becomes difficult to extract, transform, and load data into Snowflake. On the other hand, it offers extensive support via Slack and a flexible pricing structure. It suits the smaller teams with fewer ETL requirements as they can pay less than larger teams.
3) Matillion
Rating:Average user rating: 4.3/5Benefits:
- Support around 70 data sources
- Annual pricing models are available
Limitations:
- Knowledge of SQL is mandatory
- Weak click-and-point
- No tutorial clips
Matillion is a cloud-based ETL extension for Snowflake that moves data from 70 different data sources to Snowflake. But click-and-point capabilities are very limited compared to low-code counterpart Xplenty. Users can drag components to the visual framework at a certain point in a pipeline, but the complete process requires SQL expertise. Still, Matillion's data sources offer a variety of databases, social networks, CRMs, and ERPS as well as users can develop additional pipelines if necessary. Matillion ETL prices are hourly bases as well as it also offers annual plans.
4) Blendo
Rating:Average user rating: 5/5 Benefits:
- Around 50 data sources to opt from
Limitations:
- It doesn’t provide data transform mode
- Users are unable to build additional data sources
- No prerequisite knowledge is required
Blendo is an ELT (not ETL) platform that enables users to transfer data to Snowflake successfully. However, it allows extracting/loading and doesn't transform data from sources. This is troublesome for organizations to transform data from different sources before loading it to a destination warehouse, especially when compliance with data safety and regulations is the top priority. Users can request Blendo to provide data sources that fulfill their requirements but can't develop sources themselves. Still, it offers around 50 data sources to opt from, including renowned CRM and ERP systems.
5) Stitch
Rating: Average user rating: 4.7/5 Benefits
- 100 different sources as well as SaaS integrations
- Users can feed on different data sources using open-source Singer
Limitations
- Expensive, with standard plans price around $1,200 per month
- Coding skills are required
- No Data transformation
Stitch is a cloud-based ELT (not ETL) solution used by multiple companies. It doesn’t offer ELT like a previous tool, making it troublesome for organizations to prioritize data compliance and regulations. It's a perfect choice for larger teams, providing around 100+ integrations. This renowned platform makes it simple to move new data to the Snowflake database, but you must use Python programming, SQL, or another programming language.
Conclusion
Snowflake’s aforementioned ETL/ELT extensions are the perfect utilities to load your data into the destination warehouse. Still, Xplenty wins this race with its native Snowflake connector and no-code UI drag and drop interface. Xplenty enables organizations without a data engineering team to extract, transform seamlessly, and load data to Snowflake with minimum effort and technical expertise.With many integrations, a user-friendly interface for data transformations, automated workflow creations, a state-of-the-art REST API, free support, and many other incredible features, Xplenty is the perfect choice for your ETL/ELT modeling.
Snowflake vs Redshift - Complete Comparison Guide
Data is the new commodity in today’s tech-driven world. With the increasing dependencies of the world on data, it proves to be the fundamental asset for small and mid-sized businesses to the big enterprises. Dependence upon data increased as enterprises started tracking records of their data for analytics and decision-making objectives.
The international big data market is predicted to grow to 103 billion U.S. dollars by 2027 with a share of 45 percent, and the software segment will occupy a notable big data market volume by 2027.
However, to keep a managed record of these overwhelming volumes of data, a proper data warehousing solution must be adapted. A data warehouse helps users in the accessibility, integrations, and more critically on the security aspect. This blog post focuses on the discussion of state-of-the-art data warehousing solutions and their detailed comparison, i.e.,
Snowflake vs. Redshift. To understand the differences between Snowflake and Redshift, we will go through some key aspects of both platforms.
What is Redshift?
Redshift can be considered a highly managed, cloud-based data warehouse service seamlessly integrated with various business intelligence (BI) tools. The only thing left is Extract, Transform, Load - ETL process to load data into the warehouse and start making informed business decisions.Amazon makes it easier for you to initiate with a few hundred gigabytes of data and scale up or down the capacity as per your requirements. It enables businesses to enjoy the perks of their data to get fruitful business insights about themselves or their customers.
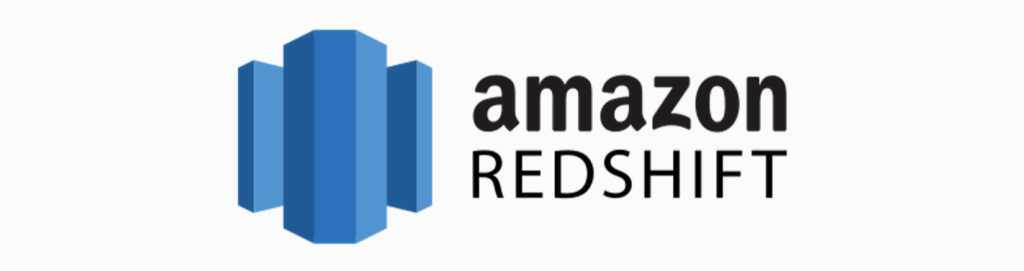
If you want to launch your cloud warehouse, you have to launch a set of nodes known as a Redshift cluster. Once you have triggered the cluster, data sets can be loaded to run different data analysis operations. Irrespective of the size of your data set, you can leverage upon fast query performance by using the same SQL-based tools and BI utilities.
What is Snowflake?
Like Redshift, Snowflake is another powerful and renowned relational database management system -RDBMS. It’s introduced as an analytic data warehouse to support structured and semi-structured data that follows a Software-as-a-Service (SaaS) infrastructure.
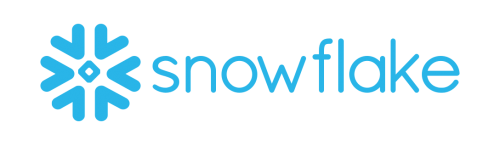
This means it’s not set up on an existing database or a big data platform (like Hadoop). Instead, Snowflake serves as an SQL database engine with a unique infrastructure specifically developed to offer cloud services.This data and analytics solution is also quick, interactive, and offers more scalability than conventional data warehouses.
Redshift vs Snowflake - Comparison
If you have used both Redshift ETL and Snowflake ETL, you’ll probably be aware of several similarities between the two platforms. However, there are additional unique capabilities and other functionalities that each platform offers differently.Suppose you’re gearing up to run your data analytics operations entirely on the cloud. In that case, the similarities between these two state-of-the-art cloud data warehousing platforms are far more than their differences.
Snowflake offers cloud-based storage and analytics in the form of the Snowflake Scalable Data Warehouse. In this case, users can analyze and store data on cloud media.Next, data will be stored in Amazon S3. If you’re using Snowflake ETL, you can benefit from the public cloud environment without any need to integrate utilities like Hadoop.These cloud warehouse infrastructures are powerful and provide some unique features for handling overwhelming amounts of data.To choose a suitable solution for your company, one must compare integrations, features, maintenance, security, and costs.
Snowflake vs Redshift: Integration and Performance
If your business is already based on AWS, then Redshift might seem like the smart choice. However, you can also opt for Snowflake on the AWS Marketplace with on-demand utilities. If you’re already using AWS services like Athena, Database Migration Service (DMS), DynamoDB, CloudWatch, Kinesis Data Firehose, etc., Redshift shows promising compatibility with all these extensions and utilities. However, if you’re planning to use Snowflake, you need to note that it doesn’t support the same integrations as Redshift. This, in turn, will make it complex to integrate the data warehouse with services like Athena and Glue. However, Snowflake is compatible with other platforms like Apache Spark, IBM Cognos, Qlik, Tableau, etc. As a result, you can conclude that both platforms are just about even equally useful and workable. While Redshift is the more defined solution, Snowflake has completed notable miles over the last couple of years.
Snowflake vs Redshift: Database Features
Snowflake makes it simpler to share data between different accounts. So if you want to share data, for instance, with your customers, you can share it without any need to copy any of the data.This is a very smart approach to working with third-party data. But at the moment, Redshift doesn’t provide such functionality. Redshift is not compatible with semi-structured data types like Array, Object, and Variant. But Snowflake is.When it comes to handling String data types, Redshift Varchar limits data types to 65535 characters. You also have to opt from the column length ahead.On the other hand, the String range in Snowflake is limited to 16MB, and the default size is the maximum String size. As a result, you don’t have to know the String size at the start of the exercise.
Snowflake vs Redshift: Maintenance
With Amazon’s Redshift, users are encouraged to look at the same cluster and compete over on-desk resources. You have to utilize WLM queues to handle it, and it can be much complex if you consider the complex set of rules that must be acknowledged and managed. Snowflake is free from this trouble. You can easily initiate different data warehouses (of various sizes) to look at the same data without any need to copy it, and multiple copies of the same data can be distributed to different users and tasks in the simplest way possible. If we talk about Vacuuming and Analyzing the tables on regular basic copying, Snowflake ensures a turnkey solution. With Redshift, it can become troublesome as it can be an overwhelming task to scale up or down. Redshift Resize operations can also become extremely expensive suddenly and lead to notable downtime. This is not the case with Snowflake due to the separate compute and storage domains, and you don’t have to copy data to scale up or down. You can just switch data compute capacity whenever required.
Snowflake vs Redshift: Security
For any big data project, security is the core of all aspects. However, it can be difficult to maintain consistency as every new data source can likely make your cloud vulnerable to evolving threats. It can generate a gap between the data generated and the data that’s being secured. When it comes to security measures, it’s not a race between Snowflake and Redshift, as both platforms provide enhanced security. However, Redshift also provides tools and utilities to handle Access management, Amazon Virtual Private Cloud, Cluster encryption, Cluster security groups, Data in transition, Load data encryption, Log-in credentials, and Secured Socket List - SSL connections. Snowflake also provides similar tools and utilities to incorporate security and regulatory compliance. But you have to be conscious while the edition as features aren’t available across all its variants.
Snowflake vs Redshift: Costs
Both Snowflake ETL and Redshift ETL have very contrasting pricing structures. If you take a deeper look, you’ll get to know that Redshift is less expensive when it comes to on-demand pricing. Both solutions provide 30% to 70% discounts for businesses who choose prepaid plans.With a one-year or three-year Reserved Instance (RI) price model, you can access additional features that you can miss out on a standard on-demand pricing model.
Redshift charges customers based on a per-hour per-node basis, and you can calculate your monthly billing amount using the following formula:
Redshift Monthly Cost = [Price Per Hour] x [Cluster Size] x [Hours per Month]
Snowflake’s price is heavily dependent on your monthly usage. This is because each bill is generated at hour granularity for each virtual data warehouse. In addition to that, data storage costs are also separate from computational costs.For instance, storage costs on Snowflake can start at an average compressed amount at a fixed rate of $23 per terabyte. It will be summed up daily and billed each month. But compute costs will be around $0.00056 per second or credit on Snowflake’s On-Demand Standard Edition.However, it can quickly become troublesome because Snowflake offers seven tiers of computational warehouses, with the most basic cluster costing one credit or $2 per hour.
The resultant bill is likely to double as you go up a level.In simple words, if you want to play safe, then Redshift is a less expensive option for you as compared to Snowflake on-demand pricing. But to leverage from notable savings, you’ll have to register for their one or three-year RI.
Snowflake vs Redshift: Pros & Cons
Amazon Redshift Pros
- Amazon Redshift is very interactive user-friendly.
- It also requires less administration and control. For instance, all you have to do is create a cluster, choose a type of instance, and then manage to scale.
- It can be easily integrated with a variety of AWS services
- If your data is stored on Amazon S3, Spectrum can easily run difficult queries. You just have to enable scaling of the compute and storage independently.
- It’s highly favorable for aggregating/denormalizing data in a reporting environment.
- It provides very fast query execution for analytics and enables concurrent analysis.
- It provides a variety of data output formats, including JSON.
- Developers with an SQL background can enjoy the perks of PostgreSQL syntax and work with the data feasibly.
- On-demand reserved instance price structure covers both compute power and data storage, per hour and per node.
- In addition to improved database security capabilities, Amazon also has a wide array of integrated compliance models.
- Offers safe, simple, and reliable backups options
Amazon Redshift Cons
- Not suitable for transactional systems.
- Sometimes you have to roll back to an old version of Redshift while you wait for AWS to launch a new service pack.
- Amazon Redshift Spectrum will cost extra, based on the bytes scanned.
- Redshift lacks modern features and data types.
- There can be complexities with hanging queries in external tables.
- To ensure the integrity of transformed tables, you’ll also have to rely on passive mediums.
Snowflake Pros
- Snowflake is suitable for enterprise-level businesses that operate mainly on the cloud.
- This data warehouse platform is extremely user-friendly and compatible with most other services.
- Its SQL interface is highly intuitive.
- Integration is simple because Snowflake itself is a cloud-based data warehouse.
- Easy to adapt and launch.
- Supports a wide array of third-party services and utilities.
- SaaS can be integrated with cloud services, data storage, and query processing.
- Data storage and compute pricing will be based on different tier and cloud providers and charged separately.
- Enable secure views and secure user-defined functions.
- Account-to-account data transfer can be done via database tables.
- Integrates easily with Amazon AWS.
Snowflake Cons
- Snowflake is not recommended if you’re running a business using on-premise infrastructure that doesn’t easily support cloud services.
- A minute’s worth of Snowflake credits will also be consumed whenever you enter a virtual warehouse but charged by the second after that.
- There’s much room for improvement as Snowflake’s SQL editor needs to be upgraded to handle automated functions.
Conclusion
The choice between Redshift and Snowflake depends upon your usage and specific business requirements. For instance, if your organization manages overwhelming workloads ranging from the millions to billions, the obvious option here is Redshift. While their model is cost-effective, companies also can reduce their expenses by opting for query speeds at a lower price value for daily active clusters. As Redshift is a renowned Amazon product, there’s also comprehensive documentation and support that can help your employees deal with any potential problem. However, the bottom line is that your data warehouse decision has to be made based on your daily usage and the amount of data you will deal with.
Data Strategy Trends for 2022 and Beyond
For many businesses, the COVID-19 pandemic changed everything from top to bottom. The recent global village shift has taught us that the world is evolving faster. Companies need to be agile and ready to move more quickly to respond to threats and opportunities that define their destiny. And this constant need to be responsive isn’t going to fade soon.At the core of successful and informed response time is data modernization that enables businesses to understand their data better. To do this, more businesses are making a transition from conventional mainframe databases to the cloud, either on-prem or a hybrid model. To implement machine learning methodologies, Robotic Process Automation, cloud computing, or any other state-of-the-art strategy to balance the turbulence of the past couple of years, businesses must adopt the changes that enable strategic utilization of the data.[lwptoc skipHeadingLevel="h1,h4,h5,h6"]Many organizations have capitalized on using data to attain scalability in their business models. The next step is to use data to yield more agility required to address troublesome black swan events in the future.
Data Strategy Trends for 2022 and Beyond
To achieve this strategic supremacy, businesses need to plan their data strategies to address trends effectively.
Data governance will take centre stage in a hybrid cloud world
Hybrid cloud will be the default option for most businesses when it comes to capacity and scalability factors. As data is distributed across the hybrid cloud, it is crucial for businesses to effectively secure and regulate their data irrespective of where it is stored or used. Businesses that lack robust security and regulatory mechanisms are on the verge of experiencing cyber-attacks and insider threats. Moreover, they’ll also struggle to comply the regulations like data privacy laws.Businesses having foreseen approaches have eliminated such issues by using an enterprise data cloud that can inhibit a state-of-the-art mechanism of security and governance policies across hybrid cloud facilities such as fine-grained access controls, data lineage, and audit logs. For instance, banks possess the most effective security methodologies and systems that can curb modern-day problems like money laundering, fake accounts, and fraudulent transactions due to the visibility of their entire data lifecycle. To counter the increasing number of cyber-attacks and data privacy concerns, more businesses should ensure that. Their data platform can provide adequate data security, regulation, and control across their hybrid cloud platforms.
Machine learning becomes accessible to everyone
As organizations are digitally evolving, they are experiencing exponential growth in amounts of data and the notable increase in complexities of modern technologies. More businesses are depending on machine learning to address those challenges. Organizations are gearing up to uncover the hidden potential of machine learning that can help them handle their network-related issues and effectively predict future uncertainties.Before adopting Machine Learning methodologies, organizations need to understand and trust on ML model’s ability to improve their business process. Businesses that have already integrated ML methodologies in their existing business model will be more likely to survive and thrive in the future.
The rise of 5G and the resulting data storm
The world will experience the 5G revolution sooner, but readiness varies between countries and regions. While China and South Korea are rapidly deploying 5G network coverage, many countries are adapting 5G infrastructure.However, more telecommunications providers around the globe are starting or continuing to enhance their existing networks to offer high-speed, low latency, and more reliable connectivity in the form of 5G. Emerging markets or new telecommunication vendors may surpass developed countries by establishing a monopoly in the 5G market as they can make a complete transition to the latest system instead of reforming their existing network infrastructure.The culture of 5G will cast a notable impact on the organization’s data strategy, as the technology can provide state-of-the-art connectivity for the Internet of Things (IoT) and live stream of data.As a 5G network can rapidly handle up to one million connected devices over one square kilometre, organizations depending on IoT must be prepared to handle the data storm created by those connected devices. It can be achieved by enterprise data cloud, which allows them to handle IoT data effectively and quickly analyze it independently or with data from other utilities like data warehouses or data lakes. This will enable the extracting of fruitful insights while securing data at every stage of the data lifecycle.
AI gets a dose of data ethics.
As more organizations capitalize on using Artificial Intelligence (AI), it will also increase regulatory and legal risks. As AI models learn from the datasets they are trained on, businesses must deal with the ethical aspect that arises from the vast collection, analysis, and use of heavy amounts of data. In recent times, ethical AI debates circulate data anonymization as more and more countries will create regulations and set SOPs for AI innovation in 2022 and beyond. Apart from that, organizations can fulfil their duty by setting up rich data governance and regulation policies across the board. Enterprise-level cloud models make this governance easy and ensure strict regulation across the data models and information infrastructures driving AI frameworks.
Provenance and Blockchain
Informed and rational data strategy involves data lineage to ensure regulatory compliance. Another way of adapting governance and regulation is to use blockchain. Provenance is a notable advantage of using blockchain. It is typically based on metadata chronicling data’s enterprise roadmap and modern approaches by utilizing distributed ledgers. Blockchain technologies are rich enough to provide the provenance of every file, including who originated and disseminated every segment of data you shared. Blockchain’s rigid traceability ensures data governance policy while restricting users from complying with it. This overwhelming capacity is applicable within and between organizations to provide transparent and satisfactory auditing.
Data Quality, Data Modeling
Data quality will always remain proactive in data strategy, data management, and data governance. It’s the basis for cleaning and preparing data to be used by decision-makers to make accurate decisions. Organizations can enhance data quality by implementing the rules and regulations that standardize how data is represented and stored. Data virtualization, knowledge graphs, and Master Data Management are unique centralized methodologies used to overcome the issues. Data modelling won’t allow you to enter bad or wrong data. Machine learning is a primary reason to ensure data quality, as flawed datasets and inputs can make ML models deliver dubious and irrational outputs. The primary centralization methods are effective because they standardize the data modelling involved, which serves as the foundation for integrating data to eliminate garbage entities from data.
End Words
Data is undoubtedly a strategic asset that enables organizations to attain the agility required to navigate through the opportunities in 2022 and beyond. However, the skyrocketing importance of data and the birth and evolution of modern technologies such as AI, ML, and Blockchain will likely create new challenges. It bounds businesses to develop intelligent and effective data strategies to address the anticipated challenges of the future and provide every employee with rich access to data to make informed and rational decisions.
Robotic Process Automation (RPA) Guide: Capabilities, Benefits & Cases
Due to skyrocketed rivalry among businesses and a race for digital supremacy, transforming the business processes needs to be considered more than ever. This is where Robotic Process Automation (RPA) comes into the frame, and industry data validates the significance of RPA.
The global robotic process automation market capital peaked at around USD 1.57 billion in 2020 and has reached USD 1.89 billion by the end of 2021. It is expected to touch $11 billion by 2027, with a growth rate of 34% from 2020 to 2027.
RPA technology is a game-changer for businesses due to its capability to eliminate or minimize the need for human effort in rules-based iterative tasks and achieve high levels of RoI. Normally, employees invest 10%-25% of their time on iterative computer tasks that reduce workers' productivity. However, a usual rules-based task can be 70%-80% streamlined by Robotic Process Automation (RPA) so that employees can spare time and effort to focus on core businesses processes.
What is Robotic Process Automation (RPA)?
With RPA, software "robots" deal with systems and data sources to streamline rules-based, iterative digital tasks. These robots are capable of performing functions 4-5 times faster than their human counterparts. These software robots perform many tasks, from logging into each application, navigating screens to copying & pasting data to assist the agent or completely automating a certain task or duty.

Depending on the nature of the task, RPA utilities are installed on back-end servers or individual employee desktops. And depending on the workload, there might be 1 to 100+ digital robots carrying out the same activity. The reason is each robot has a limited capacity like its human counterparts. However, a robot's overall capacity is much higher than a human's as robots can work 24/7 while maintaining consistency and efficiency without getting exhausted. Consider an RPA robot as a highly productive staff member who works 24/7 and never gets exhausted or bored while performing the same tasks again and again. Isn't it sound interesting?
RPA Capabilities
RPA can perform the same digital steps that humans can to accomplish iterative, clearly defined, rules-based operations. Usual RPA capabilities include:
- Searching
- Cut and paste operation
- Inputting data into multiple fields and systems within no time
- Moving data from one system to another
- Reentering data
- Deleting multiple data records
- Responding to routine queries, etc
These capabilities allow organizations to streamline any process completely or partially to make RPA ideally fit for back office and contact center use. RPA can be used for staff augmentation, spare human effort from handling iterative processes, and assist employees by providing relevant information whenever required.
How does RPA work?
There are two primary ways in which RPA can be set up, and the choice depends on the nature of the tasks that need to be streamlined and the characteristics of the systems the robots are going to interact with. Front-end RPA integrations link directly with desktop apps, but it can be done in various ways. For instance, the automation can use the UI of other apps to accomplish its tasks. This could mean the robots access the same screens and carry out the same steps as human workers. RPA can also integrate directly with databases and web APIs in the back-end as Back-end RPA. This is usually done when processes are completely streamlined and no human assistance is required. Let's see what the difference is between human supervised and unsupervised RPA.
Supervised vs. Unsupervised RPA
Back-end RPA integrations, which enable complete automation, allow operations to be performed unsupervised. The robots continuously perform tasks without any need for human supervision or assistance. This unattended automation, which completely spares employees from doing iterative tasks, is also called robotic automation.
On the contrary, supervised automation, also known as desktop automation, works parallel with human counterparts, sometimes requiring their assistance when the robots encounter unusual situations. The robots notify employees if they need human input and then continue once a response is received. Supervised automation robots can also provide workers with perspective-based assistance and suggest the appropriate next steps. This capability is fruitful for contact center agents. Supervised and Unsupervised automation aren't mutually exclusive. Organizations don't need to deploy one or the other. They can streamline some tasks using supervised and unsupervised automation to adapt the best combination of accurate, streamlined processes.
Role of AI in RPA
Not all RPA implementations leverage artificial intelligence. Some tasks are so straightforward that they don't require AI capabilities. But for more complex tasks, AI can be the right tool to make automation possible. Here are some of the forms of artificial intelligence that can enhance RPA capabilities:
Machine learning
Robots that use machine learning become smarter over time based on more data consumption and human feedback. For example, suppose a robot alerts an employee about a slight customer name discrepancy. The employee overrides the alert because the SSN on the incoming paperwork matches the SSN on the customer record. In that case, the robot will eventually learn to check the SSN for future name discrepancies. When robots get smarter, less human intervention is needed.
Natural Language Processing (NLP)
Natural language processing is sometimes called speech recognition, but its capabilities beyond just recognizing words; can also identify intent. This means, for example, robots can interpret phone conversations and act accordingly.
Also Read: How AI is Disrupting the Future of eCommerce Industry
Optical Character Recognition (OCR)
Robots with OCR capabilities can read unstructured text sources such as emails, letters, and scanned documents to identify pertinent data. This allows these robots to, for example, review a scanned driver's license, recognize the different pieces of information, and input the data into the right system fields. "Cognitive" RPA that leverages AI can further elevate automation results.
RPA in the Contact Center
Contact centers are typically replete with repetitive, rules-based tasks that are good candidates for complete or partial automation. Additionally, new and seasoned agents can always use an extra helping hand to resolve issues and perform post-interaction administrative tasks. This is why many organizations are adding RPA to their contact center software tool kits. The use of RPA for customer service generally falls into two broad categories.
Self-service
RPA can be integrated with IVR systems and chatbots to provide complete, unattended automation of self-service tasks. For example, if a customer uses a chatbot to set up a new insurance policy, the bot can interact with the customer to collect the necessary information. An RPA robot can work with the systems behind the scenes to set everything up. This is an example of advanced call center technologies working together to transform a process and provide satisfying self-service experiences.
Agent assistance
With RPA helping customers solve more of their own, simpler issues, agents will find themselves handling a more complex mix of interactions. RPA can help agents solve these tougher problems by listening to their conversations with customers and retrieving relevant knowledge base articles. Additionally, robots can suggest the next steps during the interaction. And following the conversation, robots can help perform post-contact activities like documenting calls and entering data into back-office systems. This frees up agent time and focus so they can concentrate on higher-value interactions and delivering satisfying CX.
Benefits of RPA
When designed well and used for the right tasks, RPA can deliver many benefits, including the following:
- Increasing throughput. RPA robots can be used to augment agent capacity. They work 4-5 times faster than humans and can process transactions 24/7, enabling organizations to turbocharge their throughput. With so many contact centers struggling to find qualified agent candidates, RPA can also be the tool to address labor shortages.
- Ensuring compliance. RPA robots never fat finger data entry or forget to perform process steps, increasing data accuracy and decreasing the likelihood of costly compliance violations.
- Reducing costs. Not only can RPA increase throughput and augment staff, but it does it cost-effectively. When you consider an RPA robot works around the clock at five times the speed of humans, one robot is as productive as fifteen workers.
- Increasing employee engagement. Not many people look forward to a day filled with repetitive, mundane tasks. Automating simple processes means agents can spend more time focusing on complex and engaging problem-solving.
- Easily scalable. When your organization grows, RPA is flexible enough to grow with you easily. And you don't need to hire, train, and find space for the additional robots.
Real-world RPA Cases/Usage
Real-world case studies can help illustrate how transformative robotic process automation can be. Here are examples of how three companies have effectively used RPA to streamline processes and meet their business objectives.
Banking
A major, Italian-based financial services group had established a contact center to provide business process outsourcing (BPO) services to other companies in the industry. Their 500 agents handled 650,000 calls per month, but the operation faced some challenges meeting its fraud alert SLAs. Plus, agents spent a lot of time on post-call activities, such as data entry and call documentation. To address these challenges, the organization implemented RPA. Now, robots guide agents during fraud investigations, resulting in higher accuracy and lower handle times. Additionally, robots have also reduced agent administrative burden by taking on tasks like documenting the interaction and filing claims requests. This has reduced wrap time by 82% and enabled the organization to meet process SLAs 100% of the time. And employee satisfaction has increased substantially.
Telecommunications
The telecommunications industry is highly competitive and characterized by stagnating revenue, making streamlined, cost-efficient processes a must. One major telecommunications company was struggling with inefficient, manual contact center processes causing errors and delays. In addition, costs were rapidly increasing. The organization implemented 100 robots to automate 23 back-office processes to increase accuracy, reduce delays, and decrease costs. The effort included the automation of the process used when customers rent new devices. These customers now have access to highly accurate services 24/7. The RPA reduced processing times in several areas, including an 80% reduction in the time required to rent a device. The highly scalable solution saved the business $3.5 million over 24 months.
Utilities
A leading oil and gas multinational company wanted to improve the accuracy and efficiency of customer address changes. Their 60 contact center agents processed 15,000 address changes a month with high error rates. The automation solution involved creating a single interface for agents to enter address changes. Then robots create new accounts for the new addresses and conduct meter checks. They also update the CRM system. This effort reduced agent handle times for address changes from eleven minutes to one minute, which increased their capacity to handle more interactions. Additionally, errors were eliminated, which improved CX and did away with costly error clean-up.
Is RPA taking over jobs?
RPA will inevitably lead to predictable redundancies as bots take over more work from humans. For all/most employees, once most of their responsibilities are automated, new responsibilities can be assigned. The good thing is that you will know in advance which personnel will be redundant, which gives managers time to identify new roles and train them for the transition. However, this can not be a departmental effort. HR should coordinate the new assignments, and managers across the organization should be motivated to take on employees that have become redundant. As with any industrial revolution, the post-AI world also makes some formerly valuable skills redundant. Workers who are specialized in automatable tasks will inevitably be let go if they fail to improve themselves. Though hopefully, such cases will remain rare, management must handle those cases as professionally as possible. People need support from their old managers to continue their professional lives in the best way possible.
Conclusion
Robotic Process Automation is one of the fastest-growing enterprise software categories, and there are industry experts who claim that it is hyped up. It is also frequently called dead, only to keep growing; we believe RPA will continue to help companies automate workloads, especially on on-premise systems without good API interfaces.
How to Build ETL Pipeline using Snowflake
ETL stands for Extract, Transform, and Load. With the emergence of modern cloud technologies, many businesses are shifting their data from conventional on-premise systems to cloud environments by using ETL utilities. They used to leverage conventional RDBMS, which lacked performance and scalability. To achieve excellence in performance, scalability, reliability, and recovery, organizations are shifting to cloud technologies such as Amazon Web Services, Google cloud platform, Azure, private clouds, etc.
In a general ETL scenario, ETL is a streamlined process that fetches data from conventional sources by using connectors for analysis, transforms this data by applying different methodologies like filter, aggregation, ranking, business transformation, etc. that serves business needs, and then loads onto the destination systems which is generally a data warehouse. The illustration below can give you a clear picture of how ETL works.
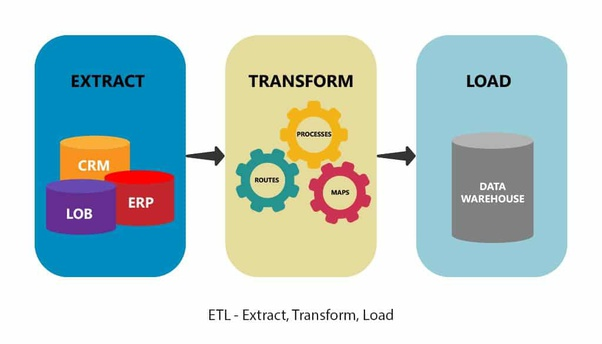
Approach towards ETL in Snowflake
The journey begins with the Snowipe, an automated utility developed using Amazon SQS and other Amazon Web Services (AWS) solutions that asynchronously listen for upcoming data as it reaches Amazon Simple Storage Service (Amazon S3) and consistently loads it into Snowflake However, Snowpipe alone does not contribute to the phase “E” (Extraction) of ELT, as only the “COPY INTO” command is allowed in a Snowpipe.
In other words, we can achieve the following objectives using Snowpipe:
- Loading data files in different formats such as CSV, JSON, XML, Parquet, and ORC
- Adopting and improving the source database for better synchronization, such as stripping outer array for JSON and stripping outer element for XML
- Altering column names
- Altering column orders
- Omitting columns
- Parsing of data/time string into data/time object
Snowpipe is not capable enough to eliminate all problems that one can face while building a data pipeline. Therefore, for the following three reasons, Streams and Tasks are required for the rest of the process:
- Snowflake does not support data transformations such as numbers calculation and string concatenation.
- The data source is not in a typical 3N normalized form, so it must be loaded into multiple tables based on certain relations.
- The ELT jobs may not be restricted to create table joins but also involve more complex requirements such as SCD (Slowly Changing Dimension).
Roadmap to Build ETL Pipeline
There are multiple ways to build the ETL pipeline. You can either create shell scripts and orchestrate using crontab, or utilize the ETL tools available to develop a customized ETL pipeline. ETL pipelines are mainly classified into two types are Batch processing and Stream processing. Let’s discuss how you can create a pipeline for batch and stream data.
Build ETL Pipeline with Batch Processing
The data is processed in batches from the source database to the destination data warehouses in a conventional ETL infrastructure. There are different tools that you can use to create ETL pipelines for your batch processing. Below are the detailed steps that you need to go through while building an ETL pipeline for batch processing :
- Step 1. Create reference data: Reference data possess the static references or permitted values that your data may involve. You need the reference data while transforming the data from source to destination. However, it is an optional step and can be excluded if you want to omit transformation (as that of an ELT process).
- Step 2. Connectors to Extract data from sources: To build the connection and extract the data from the source, you need the connectors or the defined toolset that establish the connection. The data can be from a multitude of sources and formats like API, RDBMS, XML, JSON, CSV, and any other file formats. You need to fetch all diverse data entities and convert them into a single format for further processing.
- Step 3. Validate data: After fetching or extracting the data, it is crucial to validate the data to ensure it is in the expected range and omit it. For instance, you need to extract the data for the past seven days, and you will filter out the data that will contain records older than seven days.
- Step 4. Transform data: Upon validation, further data makeup includes de-duplication of the data, cleansing, standardization, business rule application, data integrity check, aggregations, and much more.
- Step 5. Stage data: This is the phase where you store the transformed data. It is not recommended to load transformed data directly into the destination warehouse. Instead, the phase allows you to roll back your operations easily if something goes against the criteria. The staging phase also provides Dashboard and Audit Reports for analysis, diagnosis, or regulatory compliance.
- Step 6. Load to data warehouse: From the staging phase, the data is pushed to destination data warehouses. You can either opt to overwrite the existing information or add new data with the existing record whenever the ETL pipeline loads a new batch.
- Step 7. Scheduling: This is the last and most crucial phase of streamlining your ETL pipeline. You can choose the schedule to refresh and load new data based on daily, weekly, monthly, or any custom time frame. The data loaded with the schedules can include a timestamp to identify the load date, making it easier to roll back any information and check the life of available information. Scheduling and task dependencies have to be done critically to refrain from facing any memory and performance issues.
Build ETL Pipeline with Real-time Stream Processing
Many modern sources such as social media, eCommerce platforms, etc., produce real-time data that requires constant transformations as it appears. You cannot perform ETL in the same way as you do in batch processing as it requires you to perform ETL on the streams of the data by cleaning and transforming the data while it is in the transition phase to the destination systems. Several real-time stream processing tools are available in the market, such as Apache Storm, AWS Kinesis, Apache Kafka, etc. The below illustration elaborates the ETL pipeline built on the renowned and frequently used Kafka.

To create a stream processing ETL pipeline with Kafka, you have to:
- Step 1. Data Extraction:
The first step that you need to do is extract data from the source databases to Kafka using the Confluent JDBC connector or by writing custom codes that fetch each record from the source and then shift it into Kafka topic. Kafka automatically fetches the data whenever new records are found and pushes it to the topic as an update, making it a real-time data stream.
- Step 2. Pull data from Kafka topics:
The ETL application extracts the data from the Kafka topics either in JSON or in AVRO format. It is then deserialized to perform transformations by creating Kstreams. Deserialization in computing is the conversion of a string into an object.
- Step 3. Transform data:
Once you fetch the data from Kafka topics, you can perform the transformation on KStream objects with the help of Spark, Java, Python, or any other programming language. The Kafka streams handle one record and generate one or more outputs depending upon the transformation design.
- Step 4. Load data to other systems: The ETL application loads the streams into destination warehouses or data lakes after the transformation.
Conclusion
We can create an ETL pipeline using Snowflake to continuously shift data from the source to the destination data lake or warehouse. Often, raw data is first loaded temporarily into a staging table used as an interim container and then transformed with SQL queries before it is loaded into the destination. In-stream processing, this interim container is replaced by Kafka for deserialization.
Book your free 40-minute
consultation with us.
Let's have a call and discuss your product.