Discover the latest trends and insights
Data Science
Data Science Project Management [Explained]
The notable advantage of data science over mainstream statistical practices is that it can extract insights and build accurate predictions from the junk of irrelevant data. That’s how sports analysts and team selectors in cricket and football could find the most accurate KPIs and build a team that can be regarded as the best suitable option against the rival teams.
To build such intelligent models, data scientists are trained and employed to produce solutions in machine learning data models. This blog post reveals the crucial steps the data science project manager and all the stakeholders need to go through to deal with complex data science projects.
Defining Data Science
To free from any confusion or misconception, it’s essential to clear your perspective about data science and its related methodologies. Data science itself is complicated to explain in an absolute frame of reference. If you ask different Data Science experts about the absolute definition of the theme, you will be bombarded with aspects and ideologies of varying degrees.
Also Read: Data Science and Machine Learning In Demand Forecasting For Retail
The more straightforward way to define Data science is a combination of different fields of mathematics and computer science, specifically statistics and programming that deals with the practices of extracting insights and hidden patterns from lengthy datasets. Since the dependence of businesses on data increases at a high pace, the need to grasp the concept of data science and its related methodologies is the primary step to solve most of the data-related problems.
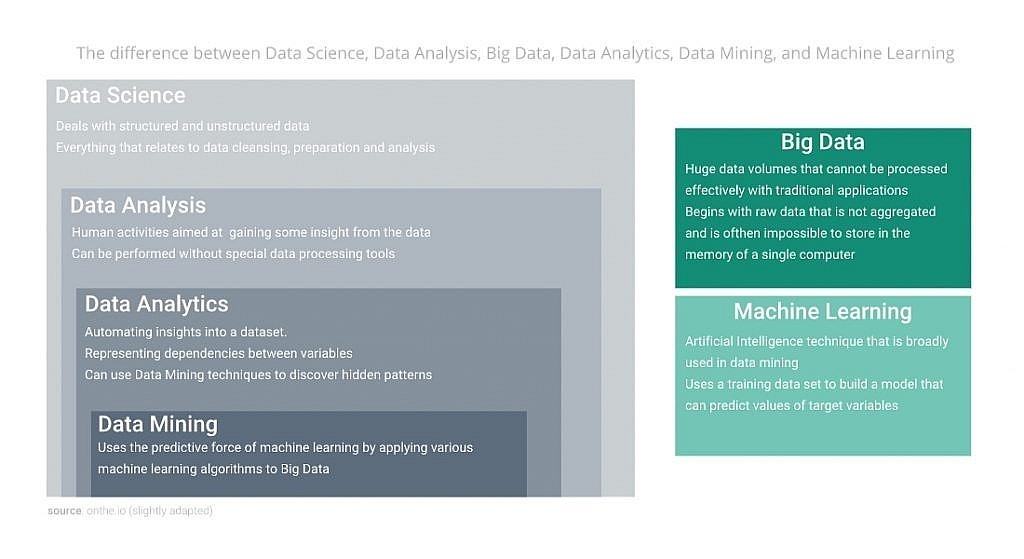
Data science and its related concepts
Despite being a technical methodology, managing a data science project is not a purely technical routine. It is a combination of both hard and soft skills. Like any other project, a data science project needs a roadmap that will guide you along the way of problem-solving. The Following rules will lead you to successful project completion while allowing you to save your valuable resources, time, and money.
Data Science Project Management
The Data Science Project involves a lifecycle to ensure the timely delivery of data science products or services. Like Agile and Scrum methodology, it is a non-linear, repetitive process richly inhabited with the critical queries, updates, and iterations based on further research and exposure to unseen depths of data as more information becomes available.

Let’s have a deeper look into how these steps allow you to handle your data science project efficiently.
Step 1: Frame the problem
Every data science project should be initialized to understand business workflows and their related challenges or hurdles. In this segment, all the stakeholders on board play a vital role in defining the problems and objectives. Even a negligible aspect of the business model should be taken into consideration to avoid any trouble later. The destiny of your project is determined at this level, making this step one of the toughest and crucial ones. But when carried out correctly, this phase can promise you a lot of savings in energy, cost, and time.
Ask business owners what they are trying to acquire. Being an analyst, the biggest challenge you will face here is to translate the blurry and obscure requirements into a well-explained and crystal-clear problem statement. The technical gap between stakeholders and experts can push a lot of communication-related problems in the process.Input from the business owner is essential, but in many cases, not substantial enough, or he/she may not convey his/her thoughts properly. A deeper understanding of business-related problems and technical expertise can help you in filling this gap. Don’t hesitate to learn anything from the sponsors regarding their domain if necessary.
A clear and concise storyline should be the primary outcome at this stage.
Since the framework is repetitive, rolling back to this stage will occur in later stages. Try to form a firm foundational basis of your project that everyone can understand and agrees on.
Step 2: Get the data
Now you can shift your focus to data gathering. You will find structured, unstructured, semi-structured; all types of data useful in different ways. Multiple data sources can cause a lot of confusion and provide space for creativity and alternatives. Potential data sources, including websites, social media, open data, or enterprise data, can be merged into data pools. The data has been identified but is not ready to be used. Before using it for the more significant motive, you need to pass it through several channels, from importing and cleaning to labeling and clustering. Cleaning the data ensures that data is free from all the errors and anomalies and loaded well into the machine. Make sure the data you are using is correct and authentic. Neglecting this crucial aspect can lead to long-term damages. Importing the data is the most time-consuming exercise as it could consume around 70 to 90 percent of the overall project time. If all the data sources are well organized, it can fall to 50 percent. You can also spare your time and effort by automating some processes in data preparation.
Step 3: Explore the data
After ensuring the quality of the collected data content, you can initiate the exploration drive. Observe the organizational efficiency of existing labels, segments, categories, and other distribution formats. Analyze the links and connections in various attributes and entities. Visualization tools may save you most of your time and energy while allowing you to better understand the layout of data content better understand the structure of data content and uncover essential better insights. The first purposeful glance at the data reveals you most of the gaps and misunderstanding that might be overseen in previous steps. Don't hesitate to roll back to the earlier stages, specifically the data collection, to close the gaps.

Example of data visualization tool to discover insights
The most troublesome phase in this step is to test those concepts and processes that are most likely to turn into insights. Revisit the results from the problem framing step to find some essential questions or ideas that will enable you to improve your data exploration drive. Like all the other projects, project duration, timelines, and deadlines will also restrict you from rolling back again and again. It bounds you to reconsider all the scenarios and aspects before revisiting the previous levels. Set your priorities aligned. Once you start observing clean patterns and insights, you are almost ready to model your data. Rethink your conclusions at every single step and filter your perspectives carefully. You are almost there.
Step 4: Model the data
At this critical level, you can perform the practical modeling of the data. Data scientists use a train set to train their machines to perform empirical predictive analysis and decision-making over a test set. A train set is a mighty chunk of the dataset with known output. This stage is highly repetitive. To make data align with the model, data scientists perform multiple adjustments to the parameters to best fit the data. Here fit means adjusting the parameters so that it can be used successfully with unseen data known as the test set. Once you’ve developed a model, you need to perform a validation test. This is how you ensure the accuracy and perfection of the model. If your model is either overfit or underfit, you need to rebuild it by changing the parameters and the size of the train set. Overfitting means the model gives accurate results only on the train set, and underfitting means the model delivers accurate results not even on the train set. The final product should be generalized, giving accurate results on both the training set and test set. Must ensure that your model addresses the business problem by revisiting the first stage. Keep in mind; not every model is perfect. The key to success is extracting the best out of your model in terms of accuracy and objectivity.
Step 5: Communicate the results
Last but not least, communicating the result means presenting your model to the business owner or sponsor. They certainly don’t know what to do with it and how it works. The more precise and clear the presentation, the easier for you to explain the project. Refrain from going into too many technical details; the client may not understand or may not be interested in knowing that. Document all your complex calculations and use easy wording to explain everything. Just Delivery of the model is not necessarily the last step in a data science project. It depends on the pact between you and the client. Sometimes, you and your developer team will deliver the developed model to those who will deploy it in a facility intended to perform. If you’re developing a predictive model used, for example, an e-commerce platform, you need to stay active on the server and extract insights and make predictions based on real-time customer data. If it is not part of your agreement, you must ensure that your whole work and calculations are documented to read like a user’s manual. Archive all of the methodologies that you’ve implemented. It includes evolution diagrams, graphs, and other visualizations of the data in every step, from raw form to cleaned information and the final model with relevant notes, comments, illustrations, and the programming code. By doing so, your client will be able to crack all the technical stuff you have done developing the model. If anybody needs to go back and verify the analysis, it becomes easier for them to use the guidelines and manual you provided.
Conclusion
The Data Science project demands immense transparency and collaboration. Irrespective of how much expertise you have, you cannot build an accurate model without knowing the crux of the business process. In the same way, business owners, CEOs, managers, and sales executives cannot achieve success without data science in a highly dynamic market. Refrain from working in isolation. Communicate with all the involved stakeholders and don’t hesitate to visit any previous stage if necessary. For the sake of authenticity, use authentic and information-rich data sets or results will always be the same or imperfect, while expenditure will be much higher in terms of time and money.
How to Build Serverless ETL Pipelines on AWS
Data has become an integral part of every company. The complexity of data processing is rising with the data amount, rate, and variety. The problem arises from the amount and complexity of measures required to bring information to a state easily accessed by business users. Data engineering teams also invest their time constructing and improving ETL pipelines. In this article, we will discuss the usage and process to design and implement the serverless ETL pipelines on AWS.
Technical developments have substantially changed the software development environment. Cloud platforms such as IaaS and PaaS have prompted businesses towards better feasibility. Likewise, serverless computing has facilitated code implementation into development.

Image Source: Cuelogic
Serverless Cloud Providers such as Amazon Web Services (AWS) operate the server and technology needed for data collection, computation, routing, event notification, and display for data applications. AWS offers a range of professionally controlled software, including planning, scaling, and management, utilizing a smart payment model to develop and maintain business software. As businesses create a serverless pipeline, Amazon S3 is typically the primary data store that they use. Since Amazon S3 is very versatile and accessible, it is ideally suited as a unified source for data.
Usage of ETL Pipeline
ETL (extract, transform, load) is a data aggregation related to the three measures used to integrate data from different sources. It is frequently used for the building of a data warehouse.
Serverless ETL has become the vision for teams who wish to concentrate on their core tasks instead of operating a massive data pipeline infrastructure.
Data is obtained and analyzed in the ETL pipeline's extraction portion from various networks, such as CSVs, online services, social networking sites, CRMs, and other business systems.
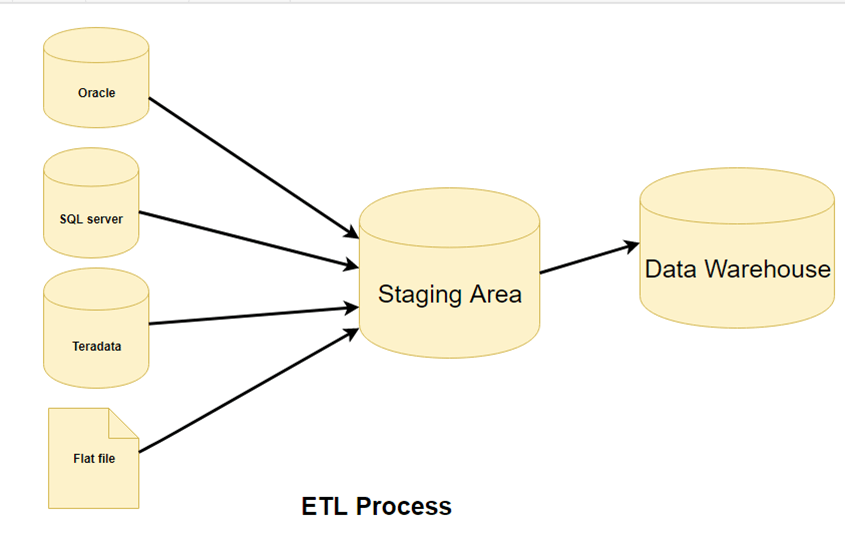
Image Source: Guru99
In the process transformation phase, the data is then modeled in a form that simplifies documentation. Data cleansing is also often part of this phase. The processed data is loaded into a single hub in the loading phase to make it convenient for all participants.
Related: ETL Pipeline and Data Pipeline – How to create an ETL Process
The ETL pipeline aims to identify, report, and maintain the correct data in a form that makes it simple to view and evaluate. An ETL tool would encourage developers to concentrate on logic/rules rather than create the means to apply the code. It eliminates the time that the developers use to develop the tools, which helps them concentrate on other essential jobs.
Building Serverless ETL Pipelines on AWS
A serverless architecture on AWS is based upon the following processes:
Data Ingestion
AWS provides a wide variety of data intake tools to help. You may use the Kinesis streaming media tools family to ingest data and use Kinesis analytics to evaluate the details while streaming and deciding on the data before it goes on in the data lake. Kinesis Analytics can evaluate the log data from the system and assess if the logs are out of the data range, and mark it for intervention before it fails. AWS also provides Database Migration Services (DMS). It offers businesses for on-prem devices that do not generally communicate to object storage or the analytics interface but instead speak to a file system. You may use an AWS Storage Gateway for the integration or an AWS Snowball for data collection and 'Lift & Shift' to the cloud.

Image Source: AllCloud
After that, you should set up AWS Direct Connect and start creating a direct network link between the on-prem setting in the AWS services, whether the cluster is existing on-prem, a data warehouse, or even a big storage unit. Data intake is essential to keeping the data feasible, and you need to select the best method with the right data form.
A Searchable Catalog
A searchable catalog is essential to build a data lake. Without it, you will just have a storage platform and not a data lake. You need it to get insights from your data. AWS Glue comes into play here as it is a stable, scalable data catalog created as data enters the Data Lake. It quickly crawls data to build a classified catalog. After processing the results, you have to be able to show them and gain insights. This can be achieved directly by Spark SQL analytical software. You get a range of resources utilizing the AWS framework, such as API Gateway, Cognito, AWS AppSync, to help you create those user interfaces over your data lake.
Also Read: Next Gen Application Microservices Architecture on Kubernetes
Data Security Management
Data confidentiality and governance management are fundamental factors, as an unsafe data lake is unusable. In the end, a data lake means taking a bunch of individual data silos, combining them, and providing more information from a full perspective. On AWS, you have a broad range of protection services such as Identity and Access Management, enabling you to safely manage access to AWS facilities and benefits. With IAM, you can build, control, and use permissions to allow and reject access to AWS services to AWS users and groups. AWS's Key Management Service (KMS) helps you to build, maintain, and track cryptographic keys through a wide variety of AWS services and applications.

Image Source: AWS
Amazon CloudWatch helps you continue tracking AWS services and programs such that configurations and adjustments can be conveniently measured, evaluated, and registered. You can use various resources to handle data in a very stable, scalable, and granular fusion.
Data Transformation
AWS helps you quickly access serverless analysis using AWS Step Functions and AWS Lambda to coordinate various ETL workflows utilizing multiple technologies. AWS Lambda helps you to execute applications without servers. One can run code with zero administration for almost any form of program or back-end operation using Lambda. You need to upload your code, and Lambda manages all the scaling. AWS Step Functions is a web service that helps organize distributed apps and microservices modules using visual workflows. Each component executes a particular role or activity that allows you to scale and modify applications quickly.

Image Source: AWS
AWS offers easy access to scalable computational and processing capabilities to quickly extend almost every large-scale framework, including data collection, fraud identification, clickstream analytics, serverless computing, and IoT processing.
Conclusion
You can use the AWS Glue ETL for two types of works: the Apache Spark and the Python shell. The Python shell work enables you to execute small tasks utilizing a fraction of the machine resources. The Apache Spark helps you conduct medium to broad functions that are more numerical and memory demanding using a distributed processing system. As serverless services become more efficient, we will see more companies using traditional architectures to be serverless. Anyone who is planning to build a new data platform should start with serverless. Let us know know in the comments section below if you have any query regarding designing and implementing the Serverless ETL Pipelines on AWS.
Data Science Vs Data Analytics Vs Machine Learning: Know the Difference
Artificial Intelligence has been around since the mid of 20th Century. Until the late 90s, there was a lack of desired processing and computational power to implement AI, and it seems impossible to acquire efficiency in this field as a futurist of that time knew would be possible in the near or far future. The rebirth of Artificial Intelligence in a previous and ongoing decade, because of the discoveries made in the processing domain, the advent of powerful Micro-Processors, and advanced GPUs, coined terms like Machine Learning, Data Science, and Data Analytics.
Data Science
Data science as revealed by its name is all about data. As Science is a general term that includes several other subfields and areas, data science is a general term for a variety of algorithms and methodologies to extract information. Under the curtain of data science, the roots exist in the form of scientific methods, detailed mathematics, statistical models, and a variety of tools. All these tools and methodologies are used to analyze and manipulate data. If any method or technique can be used as a tool to analyze data or retrieve useful information from it, it likely falls under the umbrella of data science.
Machine Learning
Machine Learning is a sub-methodology of a very general and vast field of AI. We also regard Machine Learning as one of the several ways of implementing AI. As revealed by its name Machine Learning, it is used in scenarios where we want to make a machine learn and extract from the overwhelming amounts of data.
Data Analytics
If data science is the hanger that serves as a general hub to contain tools and techniques, data analytics is a specific chamber in that hanger. It is linked with data science, but more concentrated than its parent field as instead of just uncovering connections between different elements of data, data analyst deals with the sorting of data, combining separate data segments to establish fruitful outcomes that can assist an organization in achieving their objective. It is done by grouping data into two segments.
Why The Differences Matter
These negligible differences while discussing Data Science vs Data Analytics or Data Science vs Machine Learning, can cast different shadows on the goal™s aspect. As the job roles of Data Analyst, Data Scientist, and Machine Learning Engineer are considerable. Â Experts in these fields have different prerequisite knowledge and background. A debate rises during the recruitment process while announcing a vacancy to hire these experts then it becomes obligatory for the companies to use suitable terms to attract the right people for the right job.
Data analytics and data science can be used to extract and uncover different insights from data, Machine Learning involves the development, training, and testing of Machine Learning Model to develop the intelligent machine. No doubt all these three areas possess immense importance in the IT world but they wont be used alternatively for each other. Machine Learning is usually involved in making Models for pattern recognitions, biometrics recognition, and developing intelligent machines, In contrary Data analytics is used in areas like healthcare, tourism, and stock markets, while Data Science deals with the study of patterns in internet searches and the use of resulting insights for digital marketing purposes.
Conclusion
One Coin Two Sides, One is Dark and One is Bright
There are a lot of aspects we can discuss these different technologies and terminologies. The area of AI and Data is so deep that millions of people across the globe have restricted their profession and life in better understanding and evolution of these technologies for the bright and comforting future of mankind. There also exists a group of futurists and experts who believe that AI and Humankind cant go side by side and results would be devastating as this advancement towards intelligent machines will push humanity towards extinction.
Dark Side
Yuval Noah Harari, an Israeli public intellectual, historian, a professor in the Department of History at the Hebrew University of Jerusalem and the author of the bestselling book 21 Lessons for the 21st Century, argues that Artificial intelligence has made humankind vulnerable in the same way as climate change and nuclear war and a technology race in genetics could threaten the entire humanity.
Bright Side
But another side of the story is, none of us know a bit about the future, and time travel is still not yet possible. At present were here in this world, experiencing the revolutionary advancements of AI, and one should welcome this technological revolution. We should only focus on how we can use these technological advancements for the betterment of mankind and make this world a safer and better place.
There are so many organizations and private firms researching how we can use Data Science and Machine Learning in the health sector to detect diseases and predict upcoming viruses for early preparations to save as many lives as we can. For instance, theres a lot of research going around how Machine Learning Models can help detect cancerous tumors, the early problems of data unavailability due to the privacy of patients are somehow solved after the remarkable development of deep fakes technology.
Book your free 40-minute
consultation with us.
Let's have a call and discuss your product.